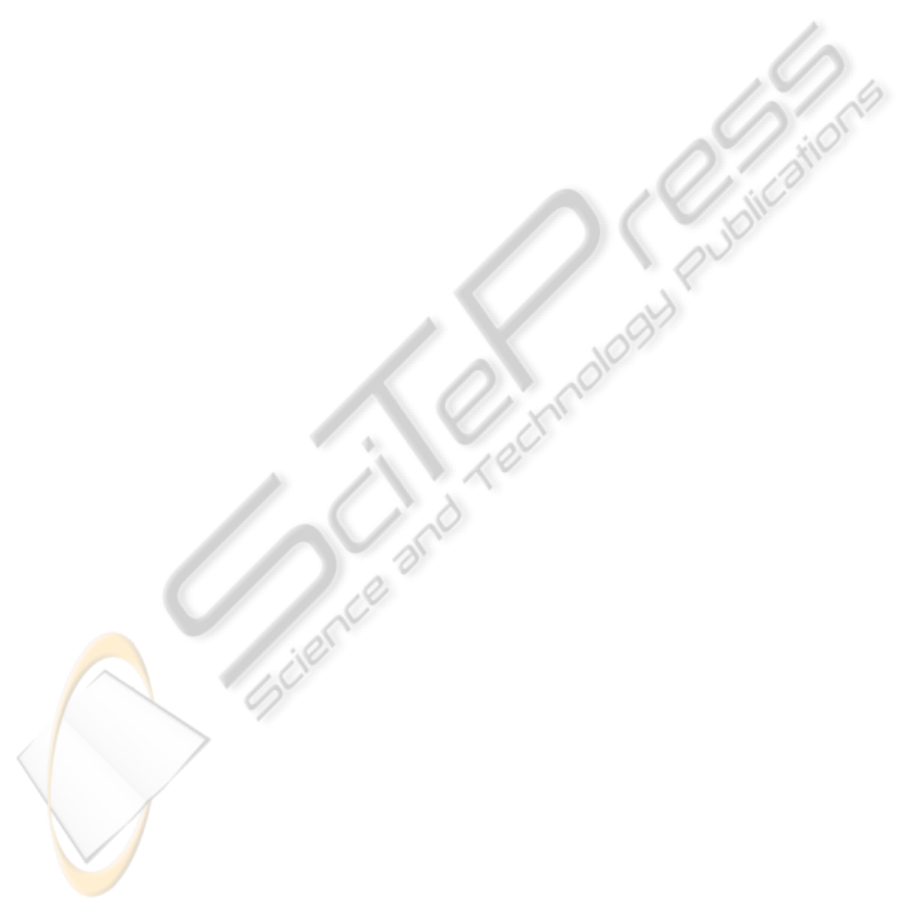
Cuturi, M., Vert, J.-P., Birkenes, O., and Matsui, T. (2006).
A kernel for time series based on global alignments.
Compute Research Repository.
Damianou, A. C., Titsias, M. K., and Lawrence, N. D.
(2011). Variational gaussian process dynamical sys-
tems. In Neural Information Processing Systems Con-
ference, pages 2510–2518.
Gong, D. and Medioni, G. (2011). Dynamic manifold warp-
ing for view invariant action recognition. In Interna-
tional Conference on Computer Vision.
H
¨
ardle, W. and Simar, W. (2003). Applied Multivariate Sta-
tistical Analysis. Springer Verlag.
Lawrence, N. D. (2003). Gaussian process latent variable
models for visualisation of high dimensional data. In
Neural Information Processing Systems Conference.
Lawrence, N. D. and Qui
˜
nonero Candela, J. (2006). Lo-
cal distance preservation in the gp-lvm through back
constraints. In International Conference on Machine
learning, pages 513–520.
Li, Y., Ferm
¨
uller, C., Aloimonos, Y., and Ji, H. (2010).
Learning shift-invariant sparse representation of ac-
tions. In International Conference on Computer Vi-
sion and Pattern Recognition, pages 2630–2637.
Microsoft, C. (2010). Kinect. http://www.xbox.com/
en-US/kinect.
mocapdata.com (2011). Eyes, japan co. ltd. http://
www.mocapdata.com/.
Moeslund, T. B., Hilton, A., and Kr
¨
uger, V. (2006). A sur-
vey of advances in vision-based human motion cap-
ture and analysis. Computer Vision and Image Under-
standing, 104(2-3):90–126.
Mordohai, P. and Medioni, G. G. (2010). Dimension-
ality estimation, manifold learning and function ap-
proximation using tensor voting. Journal of Machine
Learning Research, 11:411–450.
M
¨
uller, M. (2007). Information Retrieval for Music and
Motion. Springer Verlag.
M
¨
uller, M., R
¨
oder, T., and Clausen, M. (2005). Efficient
content-based retrieval of motion capture data. In SIG-
GRAPH, pages 677–685.
Muller, M., Roder, T., Clausen, M., Eberhardt, B., Kr
¨
uger,
B., and Weber, A. (2007). Documentation mocap
database hdm05. Technical Report CG-2007-2, Uni-
versit
¨
at Bonn.
Ntouskos, V., Papadakis, P., and Pirri, F. (2012). A compre-
hensive analysis of human motion capture data for ac-
tion recognition. In Proceedings of the International
Conference on Computer Vision Theory and Applica-
tions, pages 647–652.
Poggio, T. (1985). Early vision: From computational struc-
ture to algorithms and parallel hardware. Computer
Vision, Graphics, and Image Processing, 31(2):139–
155.
Rasmussen, C. and Williams, C. (2006). Gaussian pro-
cesses for machine learning. Adaptive computation
and machine learning. MIT Press.
Roweis, S. and Saul, L. (2000). Nonlinear dimensional-
ity reduction by locally linear embedding. Science,
290(5500):2323–2326.
Sheikh, Y., Sheikh, M., and Shah, M. (2005). Exploring the
space of a human action. International Conference on
Computer Vision, 1:144–149.
Shimodaira, H., Noma, K., Nakai, M., and Sagayama, S.
(2001). Dynamic Time-Alignment Kernel in Support
Vector Machine. Neural Information Processing Sys-
tems Conference, 2:921–928.
Taylor, G. W., Hinton, G. E., and Roweis, S. T. (2006).
Modeling human motion using binary latent variables.
In Neural Information Processing Systems Confer-
ence, pages 1345–1352.
Tenenbaum, J. B., Silva, V. D., and Langford, J. C. (2000).
A global geometric framework for nonlinear dimen-
sionality reduction. Science.
Titsias, M. K. and Lawrence, N. D. (2010). Bayesian gaus-
sian process latent variable model. Journal of Ma-
chine Learning Research - Proceedings Track, 9:844–
851.
Turaga, P. K., Chellappa, R., Subrahmanian, V. S., and
Udrea, O. (2008). Machine recognition of human ac-
tivities: A survey. IEEE Transasctions on Circuits and
Systems for Video Technology, 18(11):1473–1488.
Urtasun, R. and Darrell, T. (2007). Discriminative gaussian
process latent variable model for classification. In In-
ternational Conference on Machine Learning, pages
927–934.
Urtasun, R., Fleet, D. J., and Fua, P. (2006). 3d people track-
ing with gaussian process dynamical models. In Inter-
national Conference on Computer Vision and Pattern
Recognition, pages 238–245.
Urtasun, R., Fleet, D. J., Geiger, A., Popovic, J., Dar-
rell, T., and Lawrence, N. D. (2008). Topologically-
constrained latent variable models. In International
Conference on Machine Learning, pages 1080–1087.
Waltisberg, D., Yao, A., Gall, J., and Van Gool, L. (2010).
Variations of a hough-voting action recognition sys-
tem. In International conference on Pattern Recogni-
tion, pages 306–312.
Wang, J. M., Fleet, D. J., and Hertzmann, A. (2006). Gaus-
sian process dynamical models. In Neural Information
Processing Systems Conference, volume 18, pages
1441–1448.
Yao, A., Gall, J., Fanelli, G., and Gool, L. V. (2011). Does
human action recognition benefit from pose estima-
tion? In British Machine Vision Conference, pages
67.1–67.11.
Yao, A., Gall, J., and Gool, L. J. V. (2010). A hough
transform-based voting framework for action recogni-
tion. In International Conference on Computer Vision
and Pattern Recognition, pages 2061–2068.
Zhang, X. and Fan, G. (2011). Joint gait-pose manifold for
video-based human motion estimation. In European
Conference on Computer Vision, pages 47–54.
ICPRAM2013-InternationalConferenceonPatternRecognitionApplicationsandMethods
96