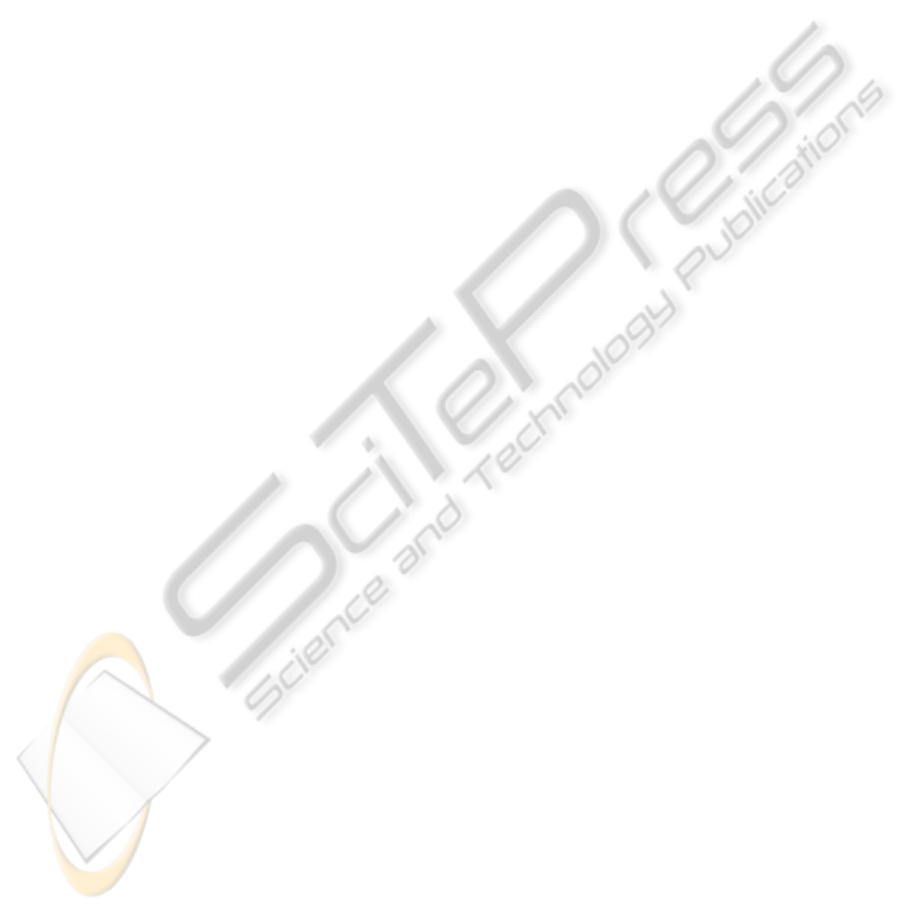
5 CONCLUSIONS
We have proposed a methodology called WGKVS,
which using image sequences recorded by stationary
cameras, segments the moving objects from the scene.
The aim of the proposed WGKVS is to construct a
background model based on an optical flow method-
ology, and using a MKL background subtraction ap-
proach, incorporates different information sources,
each source is weighted using a relevance analysis
and a tuned Kmeans algorithm is used to segment
the resulting weighted feature space. Experiments
showed that the weighted incorporation of the spa-
tial and rgb features enhances the data separability
for further clustering procedures. Moreover, the at-
tained results expose that the proposed WGKVS has
stable results using the same parameters for all the
experiments, and that it is suitable for supporting real
surveillance applications like the classification of ab-
bandoned objects. As future work, the inclussion of
other features which could enhance the process and
a methodology for the automatic actualization of the
background model are to be studied. Furthermore, the
proposed WGKVS is to be implemented as a real time
application.
ACKNOWLEDGEMENTS
This research was carried out under grants provided
by a MSc. and a PhD. scholarship provided by
Universidad Nacional de Colombia, and the project
15795, funded by Universidad Nacional de Colombia.
REFERENCES
Chen, T.-W., Hsu, S.-C., and Chien, S.-Y. (2007). Robust
video object segmentation based on k-means back-
ground clustering and watershed in ill-conditioned
surveillance systems. In Multimedia and Expo, 2007
IEEE International Conference on, pages 787 –790.
Cuesta-Frau, D., P
´
erez-Cort
´
es, J., and Andreu-Garcıa, G.
(2003). Clustering of electrocardiograph signals in
computer-aided holter analysis. Computer methods
and programs in Biomedicine, 72(3):179–196.
Daza-Santacoloma, G., Arias-Londoo, J. D., Godino-
Llorente, J. I., Senz-Lechn, N., Osma-Ruz, V., and
Castellanos-Domnguez, G. (2009). Dynamic feature
extraction: An application to voice pathology detec-
tion. Intelligent Automation and Soft Computing.
Elgammal, A., Duraiswami, R., Harwood, D., and Davis,
L. (2002). Background and foreground modeling us-
ing nonparametric kernel density estimation for visual
surveillance. Proceedings of the IEEE, 90(7):1151–
1163.
Gonen, M. and Alpaydin, E. (2010). Localized multiple
kernel regression. In Proceedings of the 20th Interna-
tional Conference on Pattern Recognition (ICPR).
Gonz
´
alez, J. C.,
´
Alvarez-Meza, A., and Castellanos-
Dom
´
ınguez, G. (2012). Feature selection by rele-
vance analysis for abandoned object classification. In
CIARP, pages 837–844.
Gutchess, D., Trajkovics, M., Cohen-Solal, E., Lyons, D.,
and Jain, A. (2001). A background model initializa-
tion algorithm for video surveillance. In Computer
Vision, 2001. ICCV 2001. Proceedings. Eighth IEEE
International Conference on, volume 1, pages 733–
740. IEEE.
Klare, B. and Sarkar, S. (2009). Background subtraction
in varying illuminations using an ensemble based on
an enlarged feature set. In Computer Vision and Pat-
tern Recognition Workshops, 2009. CVPR Workshops
2009. IEEE Computer Society Conference on, pages
66 –73.
Maddalena, L. and Petrosino, A. (2008). A self-organizing
approach to background subtraction for visual surveil-
lance applications. Image Processing, IEEE Transac-
tions on, 17(7):1168–1177.
Rakotomamonjy, A., Bach, F. R., Canu, S., and Grandvalet,
Y. (2008). SimpleMKL. Journal of Machine Learning
Research, 9:2491–2521.
Raty, T. (2010). Survey on contemporary remote surveil-
lance systems for public safety. Systems, Man, and
Cybernetics, Part C: Applications and Reviews, IEEE
Transactions on, 40(5):493–515.
VideoSegmentationbasedonMulti-kernelLearningandFeatureRelevanceAnalysisforObjectClassification
401