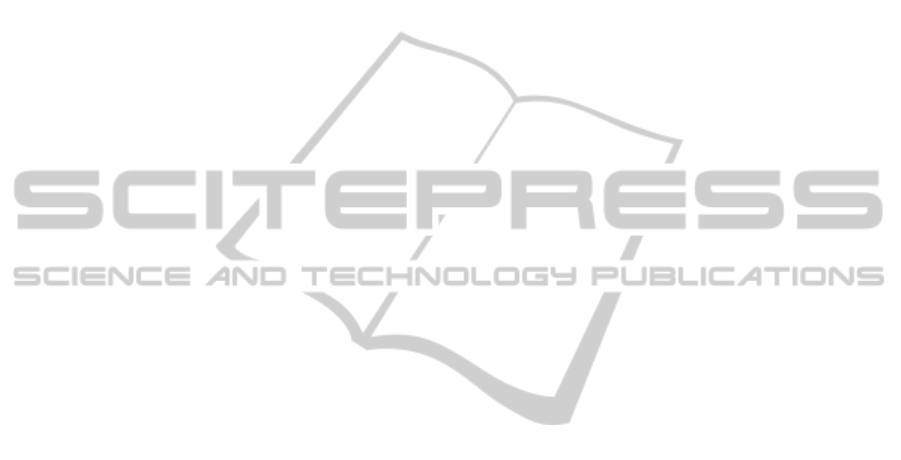
This does not preclude major changes, such as, for in-
stance, those illustrated by Figure 6 (top), in which
transitions are towards GTM nodes that, even if dis-
tant, share a rather high churn rate. In this particu-
lar case, the abrupt evolution was motivated by inad-
equate commercial actions (indiscriminate landline-
to-mobile call card gifts) that artificially modified the
usage profile without modifying the underlying cus-
tomer behaviour and propensity to churn.
Figure 6 (bottom) singles out the opposite case
of an adequate commercial action that took part of
the customers away from churn regions. In the illus-
trated example, a friend numbers campaign allowed
transferring part of the landline-to-mobile usage into
landline-to-landline usage, increasing customer usage
stability, commercial margin and LTV as a result.
5 CONCLUSIONS
The analysis of business information often requires
the use of exploratory data mining techniques.
Amongst them, MVD visualization is likely to pro-
vide invaluable insights for knowledge discovery. In
the world of telecommunication services providers,
the discovery of adequate models for the analysis
of customer churn has become paramount for the
achievement of competitive advantage. In the current
study, we have proposed a novel method of MVD vi-
sualization that combines the flexibility of the GTM
nonlinear manifold learning model with the abili-
ties of two visualization techniques from the field of
geographical representation: Cartograms and Flow
Maps. A number of experiments with a large database
of telecommunication customers have illustrated the
usefulness and actionability of the proposed MVD vi-
sualization method. High churn areas, or customer
departure gates, have been visually identified in a
manner that allows their description in terms of cus-
tomer usage and, thus, the implementation of com-
mercial campaigns oriented to increase customer re-
tention. Importantly, the method has also provided
a detailed visualization of customer migration routes,
which should enable preventive marketing actions to
avoid churn.
REFERENCES
Bishop, C. M. (1998). Latent variable models, pages 371–
404. Learning in Graphical Models. M.I.T. Press.
Bishop, C. M., Svens´en, M., and Williams, C. K. I. (1997).
Magnification factors for the GTM algorithm. In IEE
Fifth International Conference on Artificial Neural
Networks, pages 64–69. IEE.
Bishop, C. M., Svens´en, M., and Williams, C. K. I. (1998).
GTM: The Generative Topographic Mapping. Neural
Computation, 10(1):215–234.
Buchin, K., , Speckmann, B., and Verbeek, K. (2011). Flow
map layout via spiral trees. IEEE Trans. on Visualiza-
tion and Computer Graphics, 17(12):2536–2544.
Garc´ıa, D. L., Vellido, A., and Nebot, A. (2007a). Find-
ing relevant features for the churn analysis-oriented
segmentation of a telecommunications market. In
IEEE SICO 2007, II Simposio de Inteligencia Com-
putacional, pages 301–310. Thomson.
Garc´ıa, D. L., Vellido, A., and Nebot, A. (2007b). Identi-
fication of churn routes in the Brazilian telecommuni-
cations market. In ESANN’07, pages 585–590.
Gastner, M. T. and Newman, M. E. J. (2004). Diffusion-
based method for producing density-equalizing maps.
Proceedings of the National Academy of Sciences,
101(20):7499–7504.
Hadden, J., Tiwari, A., Roy, R., and Ruta, D. (2007). Com-
puter assisted customer churn management: State-of-
the-art and future trends. Computers and Operations
Research, 34(10):2902–2917.
Jain, A. K. (2010). Data clustering: 50 years beyond k-
means. Pattern Recognition Letters, 31(8):651–666.
Jolliffe, I. T. (2002). Principal Component Analysis.
Springer Series in Statistics. Springer Verlag.
Kohonen, T. (2000). Self-Organizing Maps. Information
Science Series. Springer Verlag, 3rd edition.
Lee, J. A. and Verleysen, M. (2007). Nonlinear Dimension-
ality Reduction. Information Science and Statistics.
Springer Verlag.
Phan, D., Xiao, L., Yeh, R., Hanrahan, P., and Winograd,
T. (2005). Flow Map Layout. In InfoVis’05, pages
219–224. IEEE.
Slocum, T. A. (1998). Thematic Cartography and Visual-
ization. Prentice Hall, New Jersey, U.S.A.
Tobler, W. R. (2004). Thirty-five years of computer car-
tograms. Annals of the Association of American Ge-
ographers, 94:58–73.
Tosi, A. and Vellido, A. (2012). Cartogram representation
of the batch-SOM magnification factor. In ESANN’12,
pages 203–208. d-side pub.
Vellido, A., Garc´ıa, D., and Nebot, A. (2012a). Car-
togram visualization for nonlinear manifold learning
models. Data Mining and Knowledge Discovery, doi:
10.1007/s10618-012-0294-6.
Vellido, A., Mart´ın, J. D., and Lisboa, P. J. G. (2012b).
Making machine learning models interpretable. In
ESANN’12, pages 163–172. d-side pub.
Vellido, A., Mart´ın, J. D., Rossi, F., and Lisboa, P. J. G.
(2011). Seeing is believing: The importance of visu-
alization in real-world machine learning applications.
In ESANN’11, pages 219–226. d-side pub.
IVAPP2013-InternationalConferenceonInformationVisualizationTheoryandApplications
460