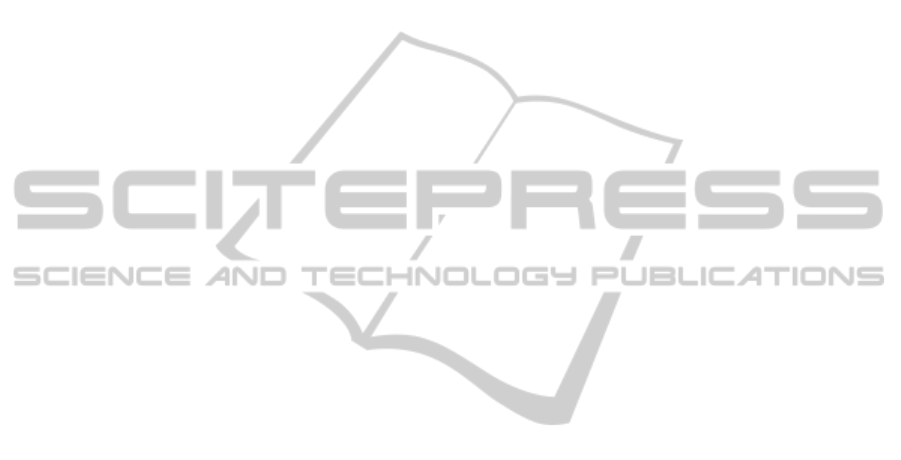
regional histograms) represents the best strategy to
identify pedestrians in a multi-camera context.
6 CONCLUSIONS AND FUTURE
WORKS
In this paper, we have introduced a new regional
color histograms feature vector to characterize a per-
son which is integrated into an extensive comparative
study between different existing descriptors based on
color, texture and shape information applied to peo-
ple re-identification and tracking in multi-camera. To
ensure this objective, two separate tests have been
performed. The first one consists in evaluating the
performances of already introduced feature vectors in
terms of people re-identification as CMC curves on
VIPER pedestrians dataset. The second test, that is
more generic, allows us to evaluate simultaneously
the discriminatory power of these descriptors in terms
of persons tracking and re-identification.
Given the complexity of the multi-camera pedes-
trians re-identification and the number of constraints
to manage, a new approach based on a fusion of de-
scriptors selected from two performed comparative
studies is presented in this paper. Two variants of the
proposed approach (cascade of color descriptors and
cascade of color and texture descriptors) are tested
and compared with several existing approaches. Ex-
perimental results show that the proposed color-based
approach provides very satisfactory performances de-
spite the highly articulated human body, lighting con-
ditions changes and large pose variations.
Future work will focus on developing a robust
behavioral analysis module and merging it with the
proposed cascade of color descriptors to improve the
multi-camera people tracking and identification per-
formances.
REFERENCES
Alahi, A., Vandergheynst, P., and Bierlaire, M. (2010). Cas-
cade of descriptors to detect and track objects across
any network of cameras. Computer Vision and Image
Understanding, 114(6):624–640.
Cai, Y., Huang, K., and Tan, T. (2008). Matching tracking
sequences across widely separated cameras. Interna-
tional Conference on Image Processing, pages 765–
768.
Derbel, A., Ben Jemaa, Y., Canals, R., Emile, B., Treuillet,
S., and Ben Hamadou, A. (2012). Comparative study
between color texture and shape descriptors for multi-
camera pedestrians identification. IEEE International
Conference on Image Processing Theory Tools and
Applications, pages 313–318.
Fleuret, F., Berclaz, J., Lengagne, R., and Fua, P. (2008).
Multi-camera people tracking with a probabilistic oc-
cupancy map. IEEE Transactions on Pattern Analysis
and Machine Intelligence, 30(2):267–282.
Freund, Y., Iyer, R., Schapire, R. E., and Singer, Y. (2003).
An efficient boosting algorithm for combining prefer-
ences. Journal ofMachine Learning Research, 4:933–
969.
Gilbert, A. and Bowden, R. (2006). Tracking ob-
jects across cameras by incrementally learning inter-
camera colour calibration and patterns of activity. Eu-
ropean Conference on Computer Vision, pages 125–
136.
Gray, D. and Tao, H. (2008). Viewpoint invariant pedes-
trian recognition with an ensemble of localized fea-
tures. European Conference on Computer Vision,
pages 262–275.
Nam, Y., Ryu, J., Choi, Y., and Cho, W. (2007). Learning
spatio-temporal topology of a multi-camera network
by tracking multiple people. World Academy of Sci-
ence Engineering and Technology, 24:175–180.
Prosser, B., Zheng, W. S., Gong, S., and Xiang, T. (2010).
Person re-identification by support vector ranking.
British Machine Vision Conference, pages 1–11.
Sharma, P. K., Huang, C., and Nevatia, R. (2009). Evalua-
tion of people tracking, counting and density estima-
tion in crowded environments. IEEE Int’l Workshop
Perf. Eval. of Tracking and Surveillance, pages 39–46.
Truong Cong, D. N., Meurie, C., Khoudour, L., Achard, C.,
and Lezoray, O. (2010). People re-identification by
spectral classification of silhouettes. Signal Process-
ing, 90(8):2362–2374.
Tung, T. and Matsuyama, T. (2008). Human motion track-
ing using a color-based particle filter driven by optical
flow. InternationalWorkshop on Machine Learning for
Vision-based Motion Analysis.
Yang, C., Duraiswami, R., and Davis, L. (2005). Fast mul-
tiple object tracking via a hierarchical particle filter.
IEEE International Conference on Computer Vision,
pages 212–219.
RobustDescriptorsFusionforPedestrians'Re-identificationandTrackingAcrossaCameraNetwork
97