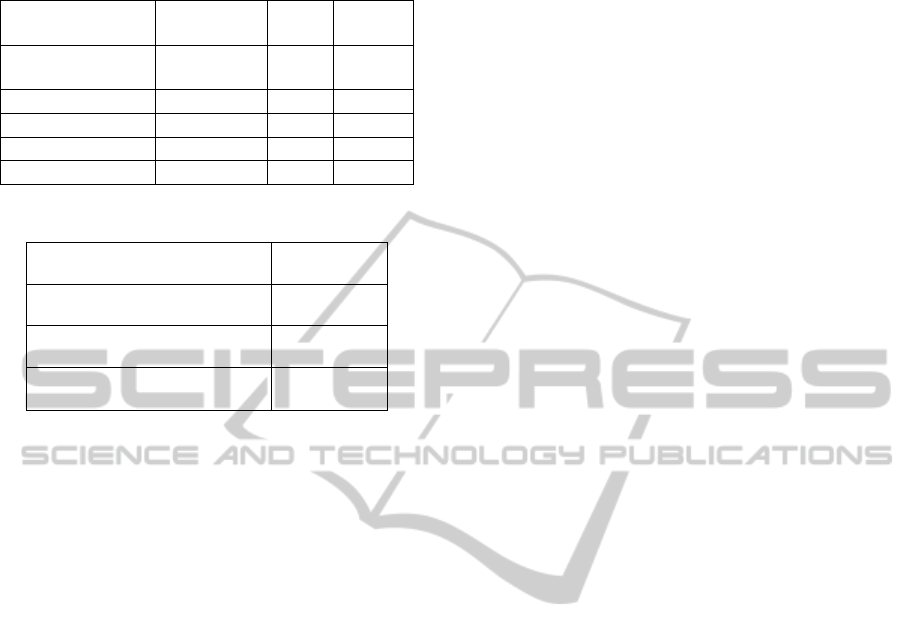
Table 2: Recognition accuracy of different pipeline safety
events.
Type of samples
Truck
passing
Digging Excavator
Number of
samples
20 20 20
Truck passing 20 0 0
Digging 0 18 1
Excavator 0 2 19
Accuracy 100% 90% 95%
Table 3: Recognition accuracy of different method.
Pattern recognition method Accuracy
WES-SVM 81.7%
WES-WIE-SVM 85%
WES-WIE-FWSVM 95%
As can be seen from table 2 and table 3, the
FWSVM proposed in this paper is very effective for
recognizing pipeline safety events. The recognition
accuracy can reach 95%. Traditional SVM cannot
satisfy the accuracy requirement because of its bad
stability. Therefore FWSVM can be applied to the
pattern recognition module of optical fiber pre-
warning system.
5 CONCLUSIONS
This paper studies the recognition of pipeline safety
events applied to optical fiber pre-warning system.
In order to solve the typical recognition problem of
pipeline safety events, the FWSVM is used in this
paper. Firstly, wavelet energy spectrum and wavelet
information entropy are used to extract features of
signals, then the FWSVM is used for recognizing the
three typical safety events. Through field
experiment, the results show that FWSVM has high
identification accuracy. The accuracy 95% is much
higher than traditional SVM. The calculation of
feature weighted kernel function can avoid kernel
function being dominated by trivial relevant or
irrelevant features. Therefore this method can satisfy
the requirement of optical fiber pre-warning system.
In the future work, recognition of more types of
pipeline safety events still need further research and
fieldexperiment.
REFERENCES
Yang, J., Wang, G. Z., 2004. Leak detection and location
methods for gas transport pipeline, Instruments in
Chemical Industry, Vol.31, No.2, pp.1-3.
Zhou, Y., Jin, S. J., Feng, H., 2007. Study on oil and gas
pipeline leakage real-time inspection system based on
distributed optical fiber, Conference Committee of the
8
th
International Symposium On Measurement
Technology and Intelligent Instruments, pp.507-510.
Qu, Z. G., Jin, S. J., Zhou, Y., 2006. Study on the
Distributed Optical Fiber Pre-warning System for the
Safety of Oil and Gas Pipeline, Piezoelectectrics
&Acoustooptics, Vol.28, No.6, pp.1-3.
Qu, W., Jia, X., Pei, S. B., Wu, J., 2008. Non-stationary
signal noise suppression based on wavelet analysis,
Congress on Image and Signal Processing, pp.303-
306.
EI-Zonkoly, A. M., Desouki, H., 2011. Wavelet entropy
based algorithm for fault detection and classification
in FACTS compensated transmission line,
International Journal of Electrical Power & Energy
Systems, Vol.33, No.8, pp.1368-1374.
Kurek, J., Osowski, S., 2010. Support vector machine for
fault diagnosis of the broken rotor bars of squirrel-
cage induction motor, Neral Computing &
Applications, Vol.19, No.4, pp.557-564.
Zhang, Y., Liu, X.D., Xie, F. D., Li, K. Q., 2009. Fault
classifier of rotating machinery based on weighted
support vector data description, Expert Systems with
Applications, Vol.36, No.4, pp.7928-7932.
RecognitionofPipelineSafetyEventsAppliedtoOpticalFiberPre-warningSystem
77