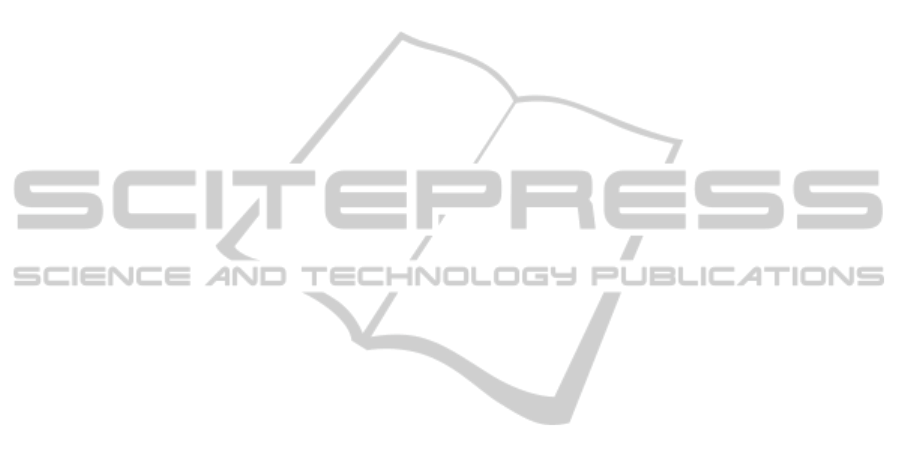
of the approach in (Jegou et al., 2008) using small
and large vocabulary with our saliency matching us-
ing the same small vocabulary. As can be seen, our
saliency matching using small vocabulary, not only
outperforms HE + WGC using the same small vocab-
ulary both in precision and recall, by 30% and 26% re-
spectively on average, but also surpasses HE + WGC
using large vocabulary by 240% and 13% respectively
on average.
Our proposed approach performs significantly
well in detecting some severe transformations, e.g.
picture in picture and text insertion, in which new vi-
sual content appears continuously in a local time win-
dow In such cases, salient visual words can capture
these discriminative visual information. However, it
is not so promising in gamma transformation. Our
conjecture is that saliency matching may not have ob-
vious advantage in videos with insufficient salient vi-
sual words.
5 CONCLUSIONS
In this paper, we propose a salient visual words selec-
tion algorithm and saliency matching to measure the
similarity between two videos. Due to the high con-
sistency in salient coordinate of BoW descriptors, the
number of mismatches will be reduced and the perfor-
mance of BoW - based approaches with small vocab-
ulary in copy detection can be improved by saliency
matching.
Our experiments have shown that our proposed
method performs well in copy detection, especially
in transformations that visual content has significant
changes along time. Despite the good performance in
some sever transformations, e.g. picture in picture,
post production and some combination of transfor-
mations, our methods do not perform well in gamma
transformation, even when enlarging the number of
matching frames. This is partly due to the insuffi-
cience of visual words, partly due to a loss of infor-
mation during quantization in BoW features, which
could be our future research directions.
REFERENCES
Douze, M., J
´
egou, H., Schmid, C., and P
´
erez, P. (2010).
Compact video description for copy detection with
precise temporal alignment. In Proceedings of the
11th European conference on Computer vision: Part
I, ECCV’10. Springer-Verlag.
Gengembre, N. and Berrani, S. (2008). A probabilistic
framework for fusing frame-based searches within a
video copy detection system. In Proceedings of the
2008 international conference on Content-based im-
age and video retrieval. ACM.
Jegou, H., Douze, M., and Schmid, C. (2008). Ham-
ming embedding and weak geometric consistency for
large scale image search. In Proceedings of the 10th
European Conference on Computer Vision: Part I.
Springer-Verlag.
Jiang, Y., J. Yang, C. N., and Hauptmann, A. (2010). Repre-
sentations of keypoint-based semantic concept detec-
tion: A comprehensive study. IEEE Transactions on
Multimedia, 12.
Kim, C. and Vasudev, B. (2005). Spatiotemporal sequence
matching for efficient video copy detection. IEEE
Trans. Circuits Syst. Video Techn.
Law-To, J., Chen, L., Joly, A., Laptev, I., Buisson, O.,
Gouet-Brunet, V., Boujemaa, N., and Stentiford, F.
(2007). Video copy detection: a comparative study.
In CIVR. ACM.
Li, D., Yang, L., Hua, X., and Zhang, H. (2010). Large-
scale robust visual codebook construction. In Pro-
ceedings of the international conference on Multime-
dia. ACM.
Liu, D., Hua, G., Paul, A., and Tsuhan, C. (2008). Inte-
grated feature selection and higher-order spatial fea-
ture extraction for object categorization. In CVPR.
IEEE Computer Society.
Mallapragada, P., Jin, R., and Jain, A. (2010). Online vi-
sual vocabulary pruning using pairwise constraints. In
CVPR’10. IEEE Computer Society.
Nist
´
er, D. and Stew
´
enius, H. (2006). Scalable recognition
with a vocabulary tree. In CVPR (2). IEEE Computer
Society.
Philbin, J., Chum, O., Isard, M., Sivic, J., and Zisserman, A.
(2007). Object retrieval with large vocabularies and
fast spatial matching. In CVPR. IEEE Computer So-
ciety.
Philbin, J., Isard, M., Sivic, J., and Zisserman, A. (2008).
Lost in quantization: Improving particular object re-
trieval in large scale image databases. In In CVPR.
Poullot, S., Buisson, O., and Crucianu, M. (2010). Scal-
ing content-based video copy detection to very large
databases. Multimedia Tools Appl.
Ren, H., Ramampiaro, H., Zhang, Y., and Lin, S. (2012).
An incremental clustering based codebook construc-
tion in video copy detection. In 2012 IEEE South-
west Symposium on Image Analysis and Interpreta-
tion. IEEE.
Wang, L. (2007). Toward a discriminative codebook: Code-
word selection across multi-resolution. In CVPR.
IEEE Computer Society.
Zhang, L., Chen, C., Bu, J., Chen, Z., Tan, S., and He, X.
(2010). Discriminative codeword selection for image
representation. In ACM Multimedia. ACM.
SmallVocabularywithSaliencyMatchingforVideoCopyDetection
773