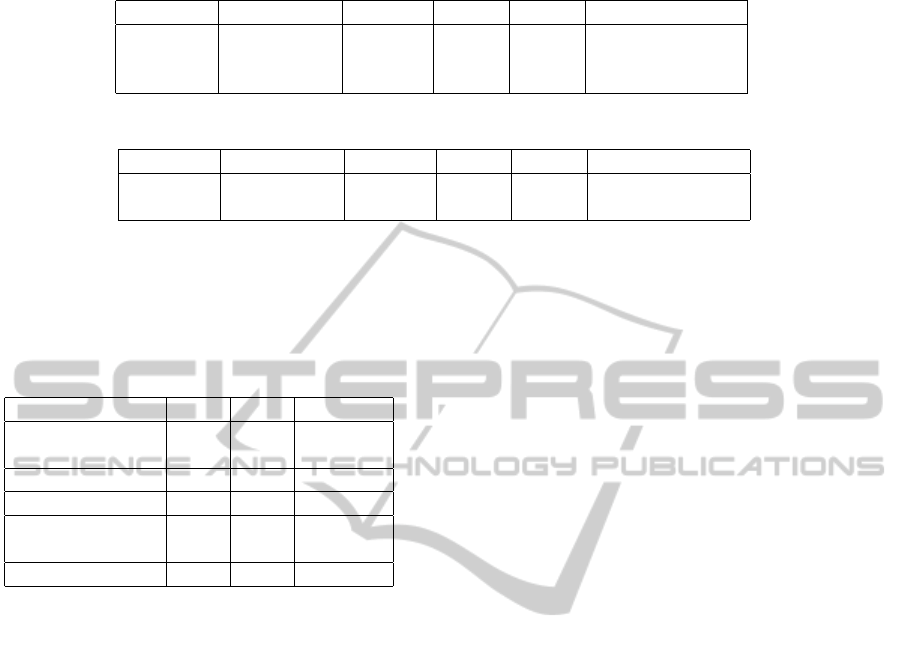
Table 1: EER for frame level abnormality detection on Ped1 and Ped2 subsets of UCSD.
Approach Social Force MPPCA MDT Reddy Proposed method
Ped1 31.0% 40.0% 25.0% 22.5% 20.0%
Ped2 42.0% 30.0% 25.0% 20.0% 15.0%
Average 37.0% 35.0% 25.0% 21.2% 17.5%
Table 2: EER for pixel level abnormality detection on Ped1 and Ped2 subsets of UCSD.
Approach Social Force MPPCA MDT Reddy Proposed method
Ped1 79.0% 82.0% 55.0% 32.0% 31.0%
Ped2 - - - - 21.0%
running at 2.30 GHz and are calculated with an aver-
age FPS in an entire test sequence run. As seen from
Table 3, the system is able to work even faster than
real-time.
Table 3: Datasets with their respective FPS using different
features.
Ped1 Ped2 Direction
Size 238 360 320
x158 x240 x240
Motion FPS 50 40 -
Size/Texture FPS 20 15
Motion & Size 18 12 -
/Texture FPS
Direction FPS 50
4 CONCLUSIONS
We propose a framework to detect multiple abnor-
malities in video surveillance, in which motion, size,
texture, with direction features are used to train inde-
pendent classifiers. Experiments show improvements
compared to related work. This result is partly caused
by less abnormalities in size and texture compared to
motion abnormalities in the datasets. Equally impor-
tantly, our proposed system is able to run faster than
real-time, which allows for connecting four or five
cameras to a single computer.
REFERENCES
(2008). Ucsd anomaly dataset. http://www.svcl.ucsd.edu/
projects/anomaly/dataset.html.
(2012). Maryland department of transportation. http://
www.traffic.md.gov/.
Boslaugh, S. and Watters, P. (2008). Statistics in a Nutshell.
O’Reilly Media, Inc.
Bouguet, J. (2000). Pyramidal implementation of the lucas
kanade feature tracker description of the algorithm.
Cui, X., Liu, Q., Gao, M., and Metaxas, D. (2011). Abnor-
mal detection using interaction energy potentials. In
CVPR.
Horn, B. and Schunck, B. (1981). Determining optical flow.
Artificial Intelligence.
Hu, W., Xiao, X., Fu, Z., Xie, D., Tan, T., and Maybank,
S. (2006). A system for learning statistical motion
patterns. PAMI.
Johnson, N. and Hogg, D. (1995). Learning the distribution
of object trajectories for event recognition. In British
conference on Machine vision.
Kim, J. and Grauman, K. (2009). Observe locally, infer
globally: A space-time mrf for detecting abnormal ac-
tivities with incremental updates. In CVPR.
Lee, T. (1996). Image representation using 2d gabor
wavelets. PAMI, 18:959–971.
Li, L., W. Huang, I. G., and Tian, Q. (2003). Foreground ob-
ject detection from videos containing complex back-
ground. In ACM international conference on Multi-
media.
Lucas, B. and Kanade, T. (1981). An iterative image regis-
tration technique with an application to stereo vision.
In International joint conference on Artificial intelli-
gence.
Mahadevan, V., Li, W., Bhalodia, V., and Vasconcelos, N.
(2010). Anomaly detection in crowded scenes. In
CVPR.
Mehran, R., Oyama, A., and Shah, M. (2009). Abnormal
crowd behavior detection using social force model. In
CVPR.
Piciarelli, C. and Foresti, G. L. (2006). On-line trajectory
clustering for anomalous events detection. Pattern
Recognition Letters, 27(15):1835–1842.
Reddy, V., Sanderson, C., and Lovell, B. (2011). Improved
anomaly detection in crowded scenes via cell-based
analysis foreground speed, size and texture. In Inter-
national Workshop on Machine Learning for Vision-
based Motion Analysis (CVPRW).
Stauffer, C. and Grimson, W. (2000). Learning patterns of
activity using real-time tracking. PAMI, 22(8):747–
757.
Yu, Q. and Medioni, G. (2009). Motion pattern interpre-
tation and detection for tracking moving vehicles in
airborne video. In CVPR.
Zhao, B., Li, F., and Xing, E. (2011). Online detection of
unusual events in videos via dynamic sparse coding.
In CVPR.
Real-timeMultipleAbnormalityDetectioninVideoData
395