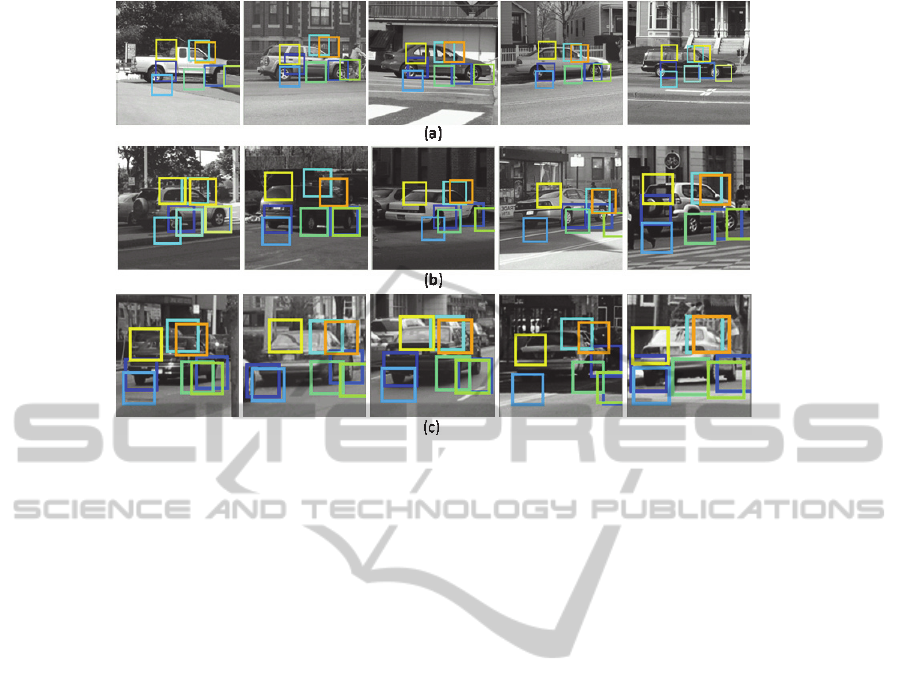
Figure 6: Parts detection examples of the adaptive model after it was applied to a single video sequence of a turning car. Car
images are from the ETHZ dataset (Cornelis et al., 2006): (a) at side-view, (b) at 30
°
view, (c) at around 60
°
view.
Transmission, 9–16.
Crandall, D., Felzenszwalb, P., & Huttenlocher, D. (2005).
Spatial priors for part-based recognition using
statistical models. Proc IEEE Conf Computer Vision
and Pattern Recognition, 10–17.
Dalal, N., & Triggs, B. (2005). Histograms of Oriented
Gradients for Human Detection. Proc IEEE Conf
Computer Vision and Pattern Recognition, 886–893.
Dalal, N., Triggs, B., & Schmid, C. (2006). Human
Detection Using Oriented Histograms of Flow and
Appearance. Proc IEEE Int Conf Computer Vision,
428–441.
Epshtein, B., & Ullman, S. (2007). Semantic Hierarchies
for Recognizing Objects and Parts. Proc IEEE Conf
Computer Vision and Pattern Recognition, 1–8.
Everingham, M., Van Gool, L., Williams, C. K. I., Winn,
J., & Zisserman, A. (2009). The PASCAL Visual
Object Classes Challenge 2009 (VOC2009) Results.
Retrieved from http://www.pascal-
network.org/challenges/VOC/voc2009
Fei-Fei, L., Fergus, R., & Perona, P. (2007). Learning
generative visual models from few training examples:
An incremental Bayesian approach tested on 101
object categories. Computer Vision and Image
Understanding, 106(1), 59–70.
Felzenszwalb, P. F., Girshick, R. B., McAllester, D., &
Ramanan, D. (2010). Object Detection with
Discriminatively Trained Part-Based Models. IEEE
Trans Pattern Anal Mach Intell, 1–20.
Felzenszwalb, P. F., & Huttenlocher, D. P. (2005).
Pictorial structures for object recognition. Int J
Computer Vision, 61(1), 55–79.
Fergus, R., Perona, P., & Zisserman, A. (2003). Object
class recognition by unsupervised scale-invariant
learning. Proc IEEE Conf Computer Vision and
Pattern Recognition, 2, 264–271.
Fergus, R., Perona, P., & Zisserman, A. (2005). A sparse
object category model for efficient learning and
exhaustive recognition. Proc IEEE Conf Computer
Vision and Pattern Recognition, 1, 380–387.
Ferryman, J. M. (2009). Workshop on Performance
Evaluation of Tracking and Surveillance. Retrieved
from http://www.cvg.rdg.ac.uk/PETS2009
Godec, M., Roth, P. M., & Bischof, H. (2011). Hough-
based tracking of non-rigid objects. Proc IEEE Int
Conf Computer Vision, 81–88.
Kalal, Z., Mikolajczyk, K., & Matas, J. (2011). Tracking-
Learning-Detection. IEEE Trans Pattern Anal Mach
Intell, 34(7), 1409–1422.
Kwon, J., & Lee, K. M. (2009). Tracking of a non-rigid
object via patch-based dynamic appearance modeling
and adaptive basin hopping monte carlo sampling.
Proc IEEE Conf Computer Vision and Pattern
Recognition, 1208–1215.
Lim, J., Ross, D., Lin, R., & Yang, M. (2005). Incremental
learning for visual tracking. In L. Saul, Y. Weiss, & L.
Bottou (Eds.), Advances in Neural Inform Process Syst
(Vol. 7, pp. 793– 800). Cambridge: MIT Press.
Lowe, D. G. (2004). Distinctive image features from
scale-invariant keypoints. Int J Computer Vision,
60(2), 91–110.
Ramanan, D., Forsyth, D., & Zisserman, A. (2007).
Tracking People by Learning Their Appearance. IEEE
Trans Pattern Anal Mach Intell, 29(1), 65–81.
Ullman, S., Vidal-Naquet, M., & Sali, E. (2002). Visual
features of intermediate complexity and their use in
classification. Nature Neuroscience, 5(7), 682–687.
Vidal-Naquet, M., & Ullman, S. (2003). Object
recognition with informative features and linear
classification. Proc IEEE Int Conf Computer Vision,
281–288.
VISAPP2013-InternationalConferenceonComputerVisionTheoryandApplications
640