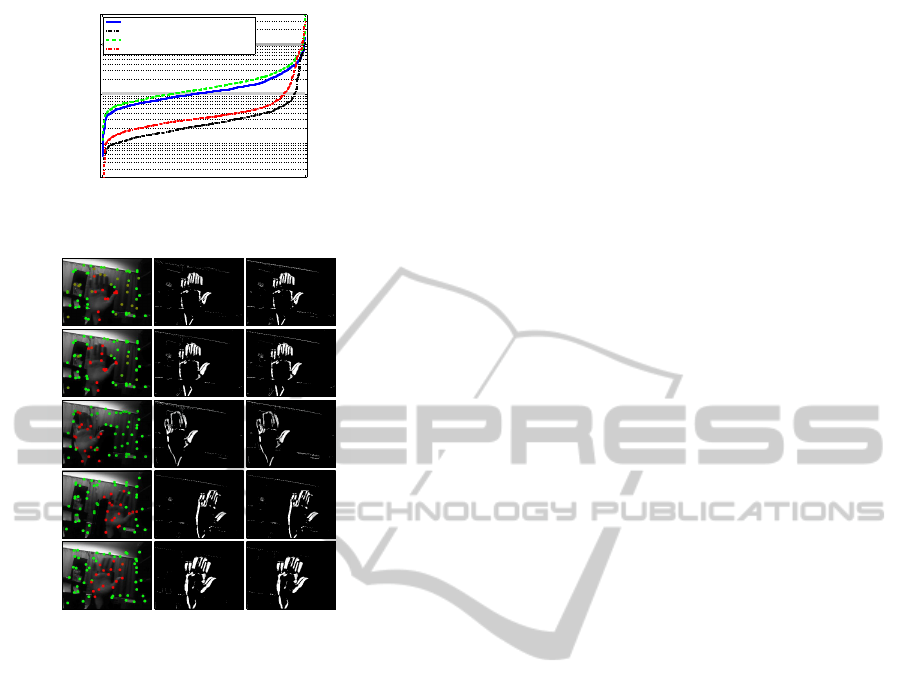
1 800
10
−2
10
−1
10
0
Analyzed frame pair instance (sorted)
RMSE [pixels]
WLSPR (64 features)
Ref. method (full support)
WLSPR (regular grid, 144 features)
Ref. method (regular grid, 12% support)
Figure 6: Comparison against the reference method.
Figure 7: Snapshots from the experiment with the Hand
sequence, Left: patch/object assignment, Middle: segmen-
tation masks computed from the WLSPR output, Right:
masks computed from the reference output.
cate that the object motion estimates provide the same
level of performance in post-processing.
4 CONCLUSIONS
In this paper, we have proposed an approach to extrac-
tion of background and foregroundmotions where the
temporal propagation of probabilistic feature associa-
tions is done. This is based on estimated displace-
ments which provides labeled seed points. Spatial
proximity of the new feature patches to those points
is then used to predict the labelling of features. This
propagation technique was integrated with iterative
refinement under the WLS estimation framework.
Experiments show that feature-based prediction of
motion provides a better starting point for segmen-
tation than the approach using dynamics. In addi-
tion, experiments show importance of using direc-
tional uncertainty information about the block motion
estimates in improving the precision and robustness
of the feature-based approach.
REFERENCES
Fradet, M., Robert, P., and P´erez, P. (2009). Clustering point
trajectories with various life-spans. In Proc. Conf. on
Visual Media Production, pages 7–13.
Hannuksela, J., Barnard, M., Sangi, P., and Heikkil¨a, J.
(2011). Camera-based motion recognition for mobile
interaction. ISRN Signal Processing, Art. Id 425621.
Kalal, Z., Mikolajczyk, K., and Matas, J. (2010). Forward-
backward error: automatic detection of tracking fail-
ures. In Proc. Int. Conf. on Pattern Recognition, pages
2756–2759.
Karavasilis, V., Blekas, K., and Nikou, C. (2011). Mo-
tion segmentation by model-based clustering of in-
complete trajectories. In ECML PKDD, volume 6912
of LNAI, pages 146–161. Springer-Verlag.
Lim, T., Han, B., and Han, J. H. (2012). Modeling and
segmentation of floating foreground and background
in videos. Pattern Recognition, 45(4):1696 – 1706.
Nickels, K. and Hutchinson, S. (2001). Estimating uncer-
tainty in SSD-based feature tracking. Image and Vi-
sion Computing, 20:47–58.
Odobez, J. and Bouthemy, P. (1995a). Robust multiresolu-
tion estimation of parametric motion models. J. Vi-
sual. Comm. Image Repr., 6(4):348–365.
Odobez, J.-M. and Bouthemy, P. (1995b). Direct model-
based image motion segmentation for dynamic scene
analysis. In Proc. Asian Conf. on Computer Vision,
pages 306–310.
Pundlik, S. J. and Birchfield, S. (2008). Real-time motion
segmentation of sparse feature points at any speed.
IEEE Trans. SMC-B, 38(3):731–742.
Sangi, P., Hannuksela, J., and Heikkil¨a, J. (2007). Global
motion estimation using block matching with uncer-
tainty analysis. In Proc. European Signal Processing
Conf., pages 1823–1827.
Tao, H., Sawhney, H. S., and Kumar, R. (2002). Object
tracking with Bayesian estimation of dynamic layer
representations. IEEE Trans. PAMI, 24(1):75–89.
Tekalp, A. M. (2000). Video segmentation. In Bovik, A.,
editor, Handbook of Image & Video Processing, chap-
ter 4.9. Academic Press, San Diego.
Tsai, D., Flagg, M., and Rehg, J. M. (2010). Motion coher-
ent tracking with multi-label MRF optimization. In
Proc. British Machine Vision Conf.
Wills, J., Agarwal, S., and Belongie, S. (2003). What went
where. In Proc. IEEE Conf. on Computer Vision and
Pattern Recognition, volume 1, pages 37–45.
Wong, K. Y. and Spetsakis, M. E. (2004). Motion segmen-
tation by EM clustering of good features. In Proc.
CVPR Workshop, pages 166–173.
Zappella, L., Llad´o, X., and Salvi, J. (2009). New trends
in motion segmentation. In Yin, P.-Y., editor, Pattern
Recognition, pages 31–46. INTECH.
SparseMotionSegmentationusingPropagationofFeatureLabels
401