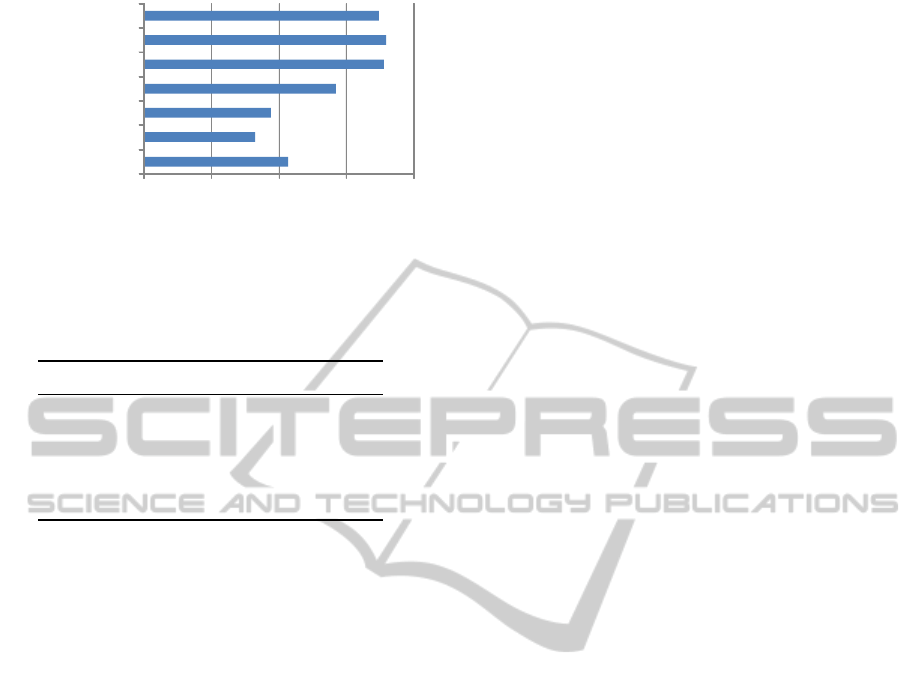
Figure 7: Comparison of the proposed SMC-LBP descrip-
tors and other texture descriptors(SMC-LBPs, N=24, the
distribution chosen Gaussian(0, 25)).
Table 2: Comparison of the proposed SMC-LBP and the
SIFT(SMC-LBPs, N=24, the distribution chosen Gaus-
sian(0, 25)).
mAP(%)
LBP(original) 28.40
SMC-LBP(hue) 34.82
SMC-LBP(Opponent) 35.87
SMC-LBP(RGB) 35.59
SIFT 38.00
is one of the most powerful image descriptors in the
literature. Comparison of the proposed SMC-LBP
and the SIFT, the performance of our texture SMC-
LBP descriptor is close to SIFT.
6 CONCLUSIONS
In this paper, we propose a novel SM-LBP which can
obtain multi-scale patterns and provide a patch texture
representation. Moveover, in order to deal with the
deficiency of color information and sensitivity to non-
monotonic lighting condition changes, SMC-LBP de-
scriptor is proposed. The main contributions are that
SM-LBP and SMC-LBP not only have more discrimi-
native power by obtaining more local information, but
also possess invariance properties to different light-
ing condition changes. In addition, they keep the ad-
vantage of computational simplicity from the original
LBP descriptor. The proposed descriptors are vali-
dated by applying on on the PASCAL VOC 2007 im-
age benchmark. Compared with the original LBP, the
experimental results exhibit better recognition accu-
racy.
REFERENCES
Blas, M. R., Agrawal, M., Sundaresan, A., and Konolige,
K. (2008). Fast color/texture segmentation for outdoor
robots. In IROS, pages 4078–4085.
Calonder, M., Lepetit, V., Strecha, C., and Fua, P. (2010).
Brief: binary robust independent elementary features.
In Proceedings of the 11th European conference on
Computer vision: Part IV, ECCV’10, pages 778–792,
Berlin, Heidelberg. Springer-Verlag.
Caputo, B., Hayman, E., and Mallikarjuna, P. (2005). Class-
specific material categorisation. IEEE International
Conference on Computer Vision (ICCV), 2:1597–
1604.
Finlayson, G. D., Hordley, S. D., and Xu, R. (2005). Convex
programming colour constancy with a diagonal-offset
model. In ICIP (3)’05, pages 948–951.
Guo, Z., 0006, L. Z., Zhang, D., and Mou, X. (2010). Hier-
archical multiscale lbp for face and palmprint recog-
nition. In ICIP, pages 4521–4524. IEEE.
Liao, S., Zhu, X., Lei, Z., Zhang, L., and Li, S. Z. (2007).
Learning multi-scale block local binary patterns for
face recognition. In Lee, S.-W. and Li, S. Z., editors,
ICB, volume 4642 of Lecture Notes in Computer Sci-
ence, pages 828–837. Springer.
Lowe, D. G. (2004). Distinctive image features from scale-
invariant keypoints. Int. J. Comput. Vision, 60(2):91–
110.
Ojala, T., Pietik
¨
ainen, M., and M
¨
aenp
¨
a
¨
a, T. (2002). Mul-
tiresolution gray-scale and rotation invariant texture
classification with local binary patterns. IEEE Trans.
Pattern Anal. Mach. Intell., 24(7):971–987.
Ozuysal, M., Calonder, M., Lepetit, V., and Fua, P. (2010).
Fast keypoint recognition using random ferns. IEEE
Trans. Pattern Anal. Mach. Intell., 32(3):448–461.
Shan, C., Gong, S., and McOwan, P. W. (2009). Fa-
cial expression recognition based on local binary pat-
terns: A comprehensive study. Image Vision Comput.,
27(6):803–816.
Tuceryan, M. and Jain, A. K. (1998). Texture analysis. In
Handbook of Pattern Recognition and Computer Vi-
sion, pages 235–276.
van de Sande, K. E. A., Gevers, T., and Snoek, C. G. M.
(2010). Evaluating color descriptors for object and
scene recognition. IEEE Transactions on Pattern
Analysis and Machine Intelligence, 32(9):1582–1596.
Yue, Y., Finley, T., Radlinski, F., and Joachims, T. (2007).
A support vector method for optimizing average preci-
sion. In Proceedings of the 30th annual international
ACM SIGIR conference on Research and development
in information retrieval, SIGIR ’07, pages 271–278,
New York, NY, USA. ACM.
Zhang, D., Wong, A., Indrawan, M., and Lu, G. (2000).
Content-based image retrieval using gabor texture fea-
tures. In IEEE Transactions PAMI, pages 13–15.
Zhu, C., Bichot, C.-E., and Chen, L. (2010). Multi-scale
Color Local Binary Patterns for Visual Object Classes
Recognition. In IEEE, editor, International Confer-
ence on Pattern Recognition (ICPR), pages 3065–
3068.
VISAPP2013-InternationalConferenceonComputerVisionTheoryandApplications
308