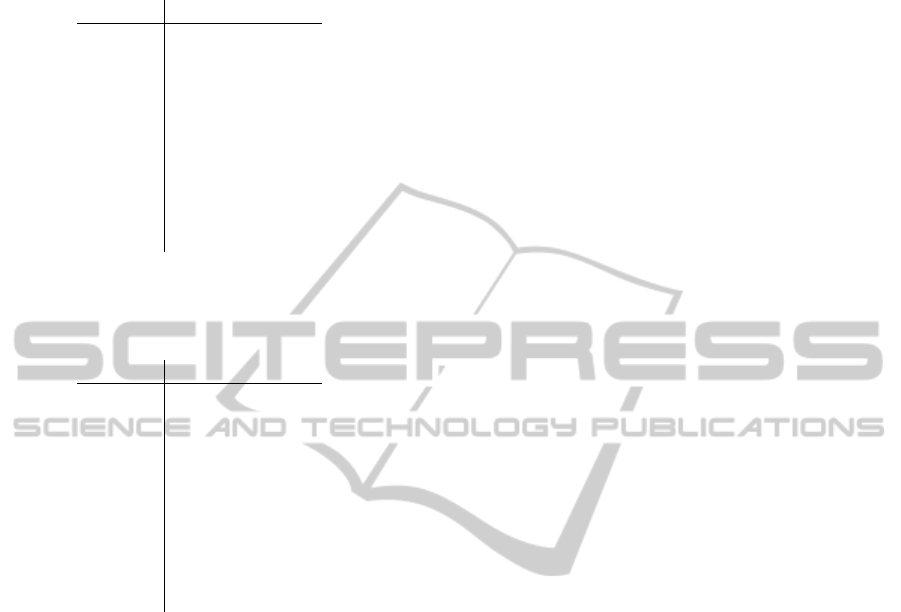
Table 1: EPF
rec
-CMA: Average RMSE-values (best and
worst) for ten different runs of the system (22), each av-
eraged over 30 repeats.
λ g best worst
100 1 13.221 15.755
100 2 12.612 14.769
100 3 12.076 15.469
100 4 12.161 14.795
100 5 12.706 14.746
200 1 14.733 16.843
200 2 14.977 17.796
200 3 15.93 19.895
200 4 15.991 19.721
200 5 16.424 19.956
Table 2: EPF
rec
-CMSA: Average RMSE-values for ten dif-
ferent runs of the system (22), each averaged over 30 re-
peats.
λ g best worst
100 1 10.335 9.263
100 2 7.39 8.778
100 3 6.072 8.312
100 4 5.54 6.958
100 5 5.592 7.632
200 1 8.829 10.576
200 2 6.505 8.156
200 3 6.358 7.924
200 4 5.81 7.428
200 5 5.832 6.870
used. Therefore, usual rank-dependent weights of the
CMSA-ES were applied. We compared the perfor-
mance of the EPF-strategy with the EPF
rec
-CMSA for
system (21), that is, without adapting the covariance
matrices. The experiments reveal no advantage for
using the EPF strategy. Instead the RMSE is always
larger than the error for the EPF
rec
-CMSA. Not using
recombination probably requires larger populations in
order to cope with stochastic fluctuations.
4 CONCLUSIONS
This paper presents new approaches for state esti-
mation in dynamical systems. State estimations of
dynamical systems have various application areas
ranging from tracking and localization tasks in au-
tonomous robots to air traffic control and fault de-
tection. In contrast to nearly all approaches, the pa-
per does not assume that the probabilistic model gov-
erning the evolution of the state variables of the sys-
tem is completely known. Instead it assumes that the
evolution equation cannot be recovered analytically
and only measurement information arrives during the
tracking. We propose the use of evolution strategies
which have been successfully applied to noisy and dy-
namic optimization. The paper presents a proof-of-
concept applying selected evolutionary particle filters
to simple dynamical systems. Since the first exper-
iments were successful, in-depth investigations will
be carried out in order to fine-tune the approaches.
REFERENCES
Arnold, D. V. and Beyer, H.-G. (2006). Optimum tracking
with evolution strategies. Evolutionary Computation,
14:291 – 308.
Arulampalam, M., Maskell, S., Gordon, N., and Clapp,
T. (2002). A tutorial on particle filters for on-
line nonlinear/non-gaussian bayesian tracking. Signal
Processing, IEEE Transactions on, 50(2):174 –188.
Beyer, H.-G. and Meyer-Nieberg, S. (2006). Self-adaptation
of evolution strategies under noisy fitness evalua-
tions. Genetic Programming and Evolvable Machines,
7(4):295–328.
Beyer, H.-G. and Schwefel, H.-P. (2002). Evolution strate-
gies: A comprehensive introduction. Natural Com-
puting, 1(1):3–52.
Beyer, H.-G. and Sendhoff, B. (2008). Covariance matrix
adaptation revisited - the CMSA evolution strategy -.
In Rudolph, G. et al., editors, PPSN, volume 5199 of
Lecture Notes in Computer Science, pages 123–132.
Springer.
Doucet, A. and Johansen, A. M. (2011). A tutorial on par-
ticle filtering and smoothing: Fifteen years later. In
Crisan, D. and Rozovsky, B., editors, Oxford Hand-
book of Nonlinear Filtering, 2011,. Oxford University
Press.
Hansen, N. (2006). The CMA evolution strategy: A com-
paring review. In Lozano, J. et al., editors, To-
wards a new evolutionary computation. Advances in
estimation of distribution algorithms, pages 75–102.
Springer.
Johansson, A. and Lehmann, E. (2009). Evolutionary op-
timization of dynamics models in sequential monte
carlo target tracking. Evolutionary Computation,
IEEE Transactions on, 13(4):879 –894.
Meyer-Nieberg, S. and Beyer, H.-G. (2007). Self-adaptation
in evolutionary algorithms. In Lobo, F., Lima, C., and
Michalewicz, Z., editors, Parameter Setting in Evo-
lutionary Algorithms, pages 47–76. Springer Verlag,
Heidelberg.
Schwefel, H.-P. (1981). Numerical Optimization of Com-
puter Models. Wiley, Chichester.
Uosaki, K. and Hatanaka, T. (2005). Evolution strategies
based gaussian sum particle filter for nonlinear state
estimation. In Evolutionary Computation, 2005. The
2005 IEEE Congress on, volume 3, pages 2365 –
2371.
ICORES2013-InternationalConferenceonOperationsResearchandEnterpriseSystems
300