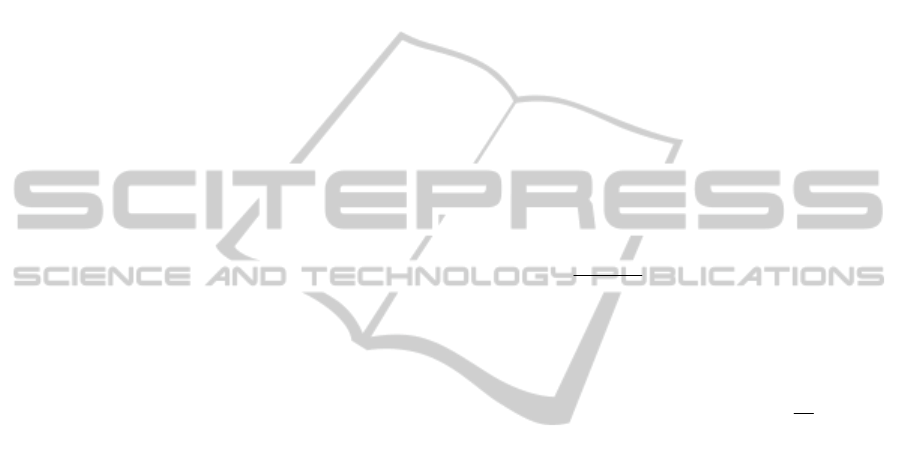
8.09%) and produce a more reliable saturation
volume than the Gompertz model.
The sensitivity analysis of both volatilities of the
Logistic models shows similar linear relations with
the stochastic optimal cut. The wood stock volatility
elasticity of 0.687 almost double the stumpage price
volatility elasticity of 0.350 due to its lower actual
volatility.
REFERENCES
Alvarez L. R., Koskela E., 2007. Optimal Harvesting
under Resource Stock and Price Uncertainty, Journal
of Economics Dynamics & Control, Vol. 31 , Issue 7,
pp. 2461-2485.
Beskos A., Papaspliopoulos O., Roberts G., 2006. Exact
computationally efficient likelihood-based estimation
for discretely observed diffusion, J. Statist.Soc.
B,68Part2, pp1-29.
Clark R., Reed W., 1989. The Tree Cutting Problem in a
Stochastic Environment, Journal of Economics
Dynamics and Control, N° 13. 569-595.
Faustmann M., 1995, (Originally,1849). Calculation of the
Value which Forest Land and Immature Stands
Processess for Forestry, Journal of Forest Economics
Vol.1: pp.7-44.
Garcia O., 2005. Unifying Sigmoid Univariate Growth
Equations, FBMIS.
Gutierrez R.,Gutierrez-Sanchez , Nafidi A:, 2008.
Modelling and forecasting vehicle stocks using trends
of stochastic Gompertz diffusion models,
Appl.Stochastic Model Bus.Ind., 25,:385.
Insley M., (2002). “A Real Option Approach to the
Valuation of a Forestry on Investment,” Journal of
Environmental Economics and Management. Vol. 44,
471-492
Insley M., Rollins K., 2005. On solving the multi-
rotational timber harvesting problem with stochastic
prices: a linear complimentarily formulation.
American Journal of Agriculture Economics.Vol87, N
3, pp. 735-755.
Jacco,J. J.,Thijssen, 2010. Irreversible Investment and
discounting: an arbitrage pricing approach, Annals of
Finance, Volume 6, Number 3, 295-315.
Johnson T.C., 2006. The optimal Timing of Investment
Decisions, PhD thesis, University of London.
Kloeden P., Platen E., 1991. Numerical Solution of
Stochastic Differential Equation, page 125, Springer-
Verlag Berlin
Meyer P., Yung J., Ausubel J., 1999. A primer on Logistic
Growth and Substitution: The Mathematics of the
Logolet Lab Software, Technological Foresting and
Social Change.
Morck, R., E. Schwartz, 1989. The valuation of Forestry
Resources under Stochastic Prices and Inventories, J.
Financial and Quantitative Analysis.Vol. 24, pp 473-
487.
Navarrete E., 2011. Modelling Optimal Pine Stands
Harvest under Stochastic Wood Stock and Price in
Chile, Journal of Forest Policy and Economics,
Doi:10.1016/j.forpol.2011.09.005.
Oksendal, B., 2000. Stochastic Differential Equations,
(Fith Ed.) Springer Verlag.
Samuelson P., 1976. Economics of Forestry in an evolving
Economy, Economic Inquiry Vol.14, pp. 466-491
Willassen Y., 1998. The stochastic rotation problem: a
generalization of Faustmann`s formula to a
stochastic forest growth, Journal of Economics
Dynamics & Control. 22, 573-596.
APPENDIX A
Proof of Lemma 1
Theorem 1: A probabilistic measure Q exists and is
equivalent to the actual metric R, such that it is
proven (see, Jacco J.J. Thijssen, 2010)
)}1/()(sup{
)1/()(
)(
sup
),(
)(
0
00
rt
t
trQ
rt
tt
rtR
o
V
eVeEP
eVPeE
tt
PVW
(A1)
Furthermore, under the metric Q, the process Vt
follows the diffusion (A2)
WdVdtVVdV
tttt
)(})(){
(A2)
Proof.
Replacing the integral solution of (2) in this last
expression (A1), Pt = P0 eαt exp {βWt - 1/2β2t],
since Mt = exp {βWt - 1/2β2t] is a martingale, a new
metric Q (dQ/dR = Mt ) can be defined via the
Radon-Nikodym derivative. Considering that, in this
case, β is positive, a straightforward application of
Girsanov´s theorems I and II (Oksendal, 2000,
pages155-157) yields the equivalent objective for
metric Q, and the ITO diffusion (A2)
ICORES2013-InternationalConferenceonOperationsResearchandEnterpriseSystems
64