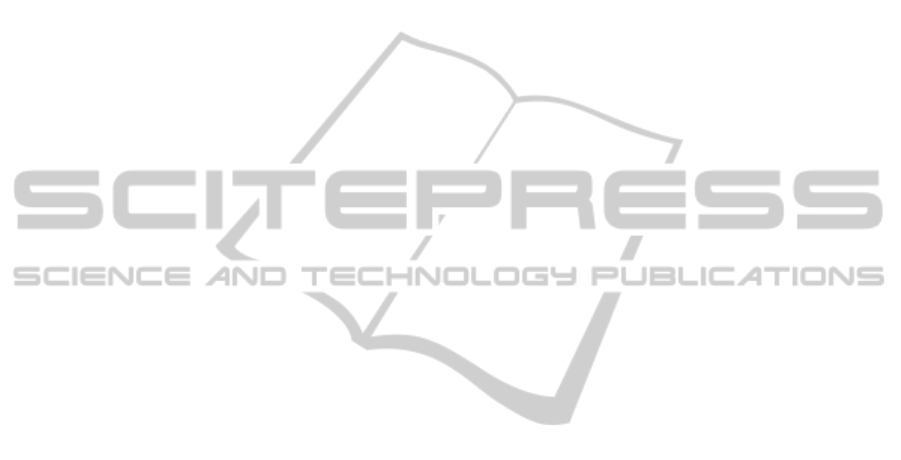
structures and combines it with contextual informa-
tion. Those contextual information are obtained from
the distribution of local structures formed from lo-
cal structures in the original image, to perform scene
recognition task. CMCT combines a modification of
CENTRIST with contextual information. Comparing
the results of MCT(8 bits) histogram and CMCT, one
can see that the introduction of contextual informa-
tion improves the image representation. Furthermore,
CMCT preserves the advantages of CENTRIST (easy
to implement, almost no parameter to tune, low illu-
mination dependence) and shows better performance,
as one can see in the presented experiments. As CEN-
TRIST, CMCT is not invariant to rotation.
The GistCMCT, in its turn, is a combination of
two holistic approach: Gist and CMCT. In Tables 1
and 2 it is possible to see that CMCT is overcomed
by Gist when only outdoor scenes are presented and
outperforms Gist when indoor scenes are classified.
The combination of these two different global des-
criptors improves classification and outperforms as
much Gist as CMCT. Besides the good performance,
GistCMCT does not need creating codebooks, which
is often computationally intense.
In our future research, we intend to use some
form of associating spatial layout information, as
subregions of different resolution levels, and include
another type of information to improve the classifica-
tion performance.
REFERENCES
Chang, C.-C. and Lin, C.-J. (2011). Libsvm: A library
for support vector machines. ACM Trans. Intell. Syst.
Technol., 2(3):1–27.
Fei-Fei, L. and Perona, P. (2005). A bayesian hierarchi-
cal model for learning natural scene categories. In
Proceedings of the 2005 IEEE Computer Society Con-
ference on Computer Vision and Pattern Recognition
(CVPR’05) - Volume 2, pages 524–531. IEEE Com-
puter Society.
Fr¨oba, B. and Ernst, A. (2004). Face detection with the
modified census transform. In Proceedings of the
Sixth IEEE international conference on Automatic
face and gesture recognition, pages 91–96. IEEE
Computer Society.
Grauman, K. and Darrell, T. (2005). The pyramid match
kernel: Discriminative classification with sets of
image features. In Proceedings of the Tenth IEEE In-
ternational Conference on Computer Vision - Volume
2, ICCV ’05, pages 1458–1465. IEEE Computer So-
ciety.
Lazebnik, S., Schmid, C., and Ponce, J. (2006). Beyond
bags of features: Spatial pyramid matching for rec-
ognizing natural scene categories. In Proceedings
of the 2006 IEEE Computer Society Conference on
Computer Vision and Pattern Recognition - Volume 2,
CVPR ’06, pages 2169–2178. IEEE Computer Soci-
ety.
Li, L.-J. and Fei-Fei, L. (2007). What, where and who?
classifying events by scene and object recognition. In
IEEE 11th International Conference on Computer Vi-
sion, pages 1–8. IEEE Computer Society.
Liu, S., Xu, D., and Feng, S. (2011). Region contextual
visual words for scene categorization. Expert Systems
with Applications, 38(9):11591–11597.
Lowe, D. G. (1999). Object recognition from local scale-
invariant features. In Proceedings of the International
Conference on Computer Vision - Volume 2, ICCV
’99, pages 1150–1157. IEEE Computer Society.
Oliva, A. and Torralba, A. (2001). Modeling the shape of
the scene: A holistic representation of the spatial en-
velope. Int. J. Comput. Vision, 42(3):145–175.
Oliva, A. and Torralba, A. (2006). Building the gist of a
scene: The role of global image features in recogni-
tion. Progress in Brain Research, 155:23–36.
Qin, J. and Yung, N. (2010). Scene categorization
via contextual visual words. Pattern Recognition,
43(5):1874–1888.
Quattoni, A. and Torralba, A. (2009). Recognizing indoor
scenes. In Proceedings IEEE CS Conf. Computer Vi-
sion and Pattern Recognition, pages 413–420. IEEE
Computer Society.
Salton, G. and McGill, M. J. (1983). Introducrion to mod-
ern information retrieval. New York: McGraw-Hill.
Vapnik, V. (1998). The support vector method of function
estimation. Nonlinear Modeling advanced blackbox
techniques Suykens JAK Vandewalle J Eds, pages 55–
85.
Wei Liu, S. K. and Gabbouj, M. (2012). Robust scene clas-
sification by gist with angular radial partitioning. In
Communications Control and Signal Processing (IS-
CCSP), 2012 5th International Symposium on, pages
2–4.
Wu, J. and Rehg, J. M. (2009). Beyond the euclidean dis-
tance : Creating effective visual codebooks using the
histogram intersection kernel. In Computer Vision,
2009 IEEE 12th International Conference on, pages
630–637. IEEE Computer Society.
Wu, J. and Rehg, J. M. (2011). Centrist: A visual descriptor
for scene categorization. IEEE Trans. Pattern Anal.
Mach. Intell., 33(8):1489–1501.
Zabih, R. and Woodfill, J. (1994). Non-parametric lo-
cal transforms for computing visual correspondence.
In Proceedings of the third European conference on
Computer Vision - Volume 2, ECCV ’94, pages 151–
158. Springer-Verlag New York, Inc.
VISAPP2013-InternationalConferenceonComputerVisionTheoryandApplications
320