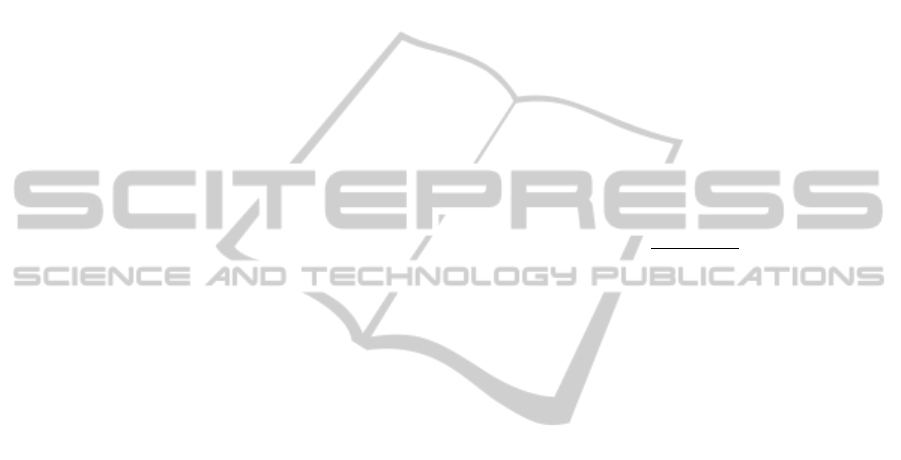
4
3
3
3
3
3
3
3
4
3
3
3
3
(11)
These moments have invariance of transform,
rotation and scaling. Thus, we can obtain a 7-
dimensional shape feature vector.
In this paper, the gaussian normalization is
applied before similarity comparison. After the
gaussian normalization, the values of feature vector
are ranged within 1,1 . Therefore, we can
effectively eliminate the difference which generates
due to the different range of the values in similarity
comparison, and make the weight coefficients of the
different components of the same feature roughly the
same.
Finally, integrating the three kinds of feature
vectors together, we can get a 111-dimensional
feature vector to represent the image content.
2.2 Image Feature Encryption
In this paper, AES and other encryption algorithms
are used to encrypt the image content, and will not
be described here due to the limited paper space.
Next, we will describe the image feature encryption
method, which is the key component in our proposed
secure image retrieval scheme.
The CBIR is based on the distance between the
image features. In order to achieve the goal of
comparing the encrypted image features without
decryption, this paper applies an algorithm called
bit-plane randomization (Lu et al., 2009) which can
preserve the distance between the encrypted image
features. In this paper, we preserve the highest bit-
planes and encrypt the subsequent two bit-planes.
The so-called bit-plane is the bits which have the
same weight. Clearly, different bit-planes have
different significance. The most significant bits
(MSB) have the most importance of value.
The specific process of encryption is as follow:
First of all, the values of the feature vector have
been normalized into [-1,1] after gaussian
normalization. In order to extract bit-planes, we use
the following equation to process all the values:
1
∗100
(12)
where e’ and e represents the processed and original
values, respectively. Then, the range of all the values
of the feature vector is [0,200]. Due to200
11001000
, we can get 8 bit-planes. Next, a
random binary string is generated as the
cryptographic key. After that, the first five MSBs are
extracted. The fourth and the third bit-planes are
XORed with the cryptographic key respectively,
while the highest three bit-planes (7-5) are preserved.
Finally, the binary values should be transformed
back into decimal notation. Thus, the values of the
image feature vector can be protected efficiently.
2.3 Similarity Comparison
In this paper, both L1 and L2 distance are used to
perform similarity comparison. L1 and L2 distance
between N-dimensional vectors can be defined as
|
|
(13)
and
∑
(14)
3 EXPERIMENTS
AND ANALYSIS
To validate the effectiveness of the proposed secure
image retrieval, we conduct an experiment on an
image database which contains two image datasets:
The Corel image database: this database contains
1000 color images which are classified into 10
categories (100 images in each category) images,
i.e. African, Beach, Architecture, Buses, Dinosaurs,
Elephants, Flowers, Horses, Mountain and Food.
Image sizes are either 256*384 or 384*256. This
database has been widely used in evaluating color
image retrieval;
The existing public datasets: we select 1232
images from the existing public datasets, such as
MSRC and Flickr. In this database, images are
classified into 8 categories, i.e. Bicycles, Buses,
Cars, Cats, Cows, Motorbikes, People and Sheep.
Image sizes are not fixed. Most of the images are
social images and are generally used for image
segmentation or recognition.
In this database, the total number of images is 2232.
After merging the similar classifications, we get 16
categories, i.e. Bicycles, Cars, Cats, Cows,
Motorbikes, People, Sheep, Beach, Architecture,
Buses, Dinosaurs, Elephants, Flowers, Horses,
Mountain and Food.
In this experiment, we evaluate the performances
SecureImageRetrievalSchemeintheEncryptedDomain
785