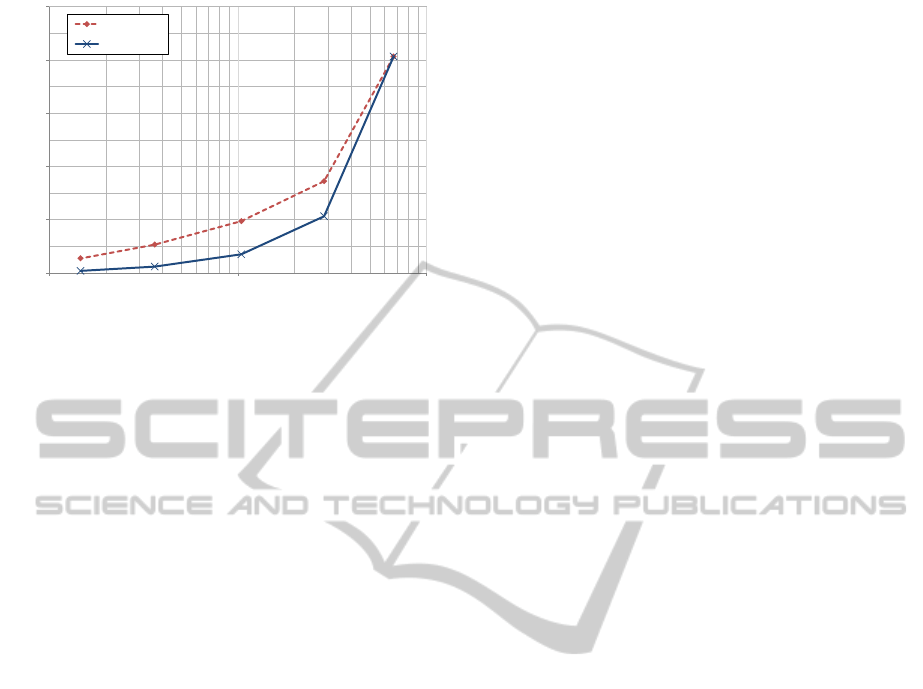
0
0.1
0.2
0.3
0.4
0.5
10 100 1000
Average Seconds per Query
Avg Distinct Words per Image
query
query&data
Figure 6: Average search time with respect to the average
number of distinct words per image obtained reducing the
visual words on the query and on query and dataset.
based on statistics of the usage of visual words in im-
ages (tf ), across the database (idf ), and on the tf*idf
combination.
In the content based image retrieval scenario the
scale approach performed best and even better than
using all the words. However, for reduction over
an order of magnitude effectiveness significantly de-
crease. In the landmark recognition task, the most in-
teresting results were obtained considering the macro-
averaged F
1
effectiveness measure with respect to the
average number of distinct words per image. The
tf*idf obtained the best results, but it is interesting
to see that the tf approach, which does not rely on
dataset information, obtained very similar results. It
is worth to note that the recognition task is more ro-
bust than the retrieval to words reduction. Moreover,
for small local features reductions scale was the over-
all best.
We plan to define new approaches and compare
with the ones proposed in this work on larger dataset
in the near future.
REFERENCES
Amato, G. and Falchi, F. (2011). Local feature based im-
age similarity functions for kNN classfication. In
Proceedings of the 3rd International Conference on
Agents and Artificial Intelligence (ICAART 2011),
pages 157–166. SciTePress. Vol. 1.
Amato, G., Falchi, F., and Gennaro, C. (2011). Geometric
consistency checks for knn based image classification
relying on local features. In SISAP ’11: Fourth In-
ternational Conference on Similarity Search and Ap-
plications, SISAP 2011, Lipari Island, Italy, June 30 -
July 01, 2011, pages 81–88. ACM.
Douze, M., J
´
egou, H., Sandhawalia, H., Amsaleg, L., and
Schmid, C. (2009). Evaluation of gist descriptors
for web-scale image search. In Proceedings of the
ACM International Conference on Image and Video
Retrieval, CIVR ’09, pages 19:1–19:8, New York, NY,
USA. ACM.
Jegou, H., Douze, M., and Schmid, C. (2009). Packing bag-
of-features. In Computer Vision, 2009 IEEE 12th In-
ternational Conference on, pages 2357 –2364.
J
´
egou, H., Douze, M., and Schmid, C. (2010). Improving
bag-of-features for large scale image search. Int. J.
Comput. Vision, 87:316–336.
J
´
egou, H., Perronnin, F., Douze, M., S
´
anchez, J., P
´
erez,
P., and Schmid, C. (2012). Aggregating local im-
age descriptors into compact codes. IEEE Transac-
tions on Pattern Analysis and Machine Intelligence.
QUAERO.
Lowe, D. G. (2004). Distinctive image features from scale-
invariant keypoints. International Journal of Com-
puter Vision, 60(2):91–110.
Perronnin, F., Liu, Y., Sanchez, J., and Poirier, H. (2010).
Large-scale image retrieval with compressed fisher
vectors. In Computer Vision and Pattern Recogni-
tion (CVPR), 2010 IEEE Conference on, pages 3384
–3391.
Philbin, J., Chum, O., Isard, M., Sivic, J., and Zisserman,
A. (2007). Object retrieval with large vocabularies
and fast spatial matching. In Proceedings of the IEEE
Conference on Computer Vision and Pattern Recogni-
tion.
Salton, G. and McGill, M. J. (1986). Introduction to Mod-
ern Information Retrieval. McGraw-Hill, Inc., New
York, NY, USA.
Sivic, J. and Zisserman, A. (2003). Video google: A text
retrieval approach to object matching in videos. In
Proceedings of the Ninth IEEE International Confer-
ence on Computer Vision - Volume 2, ICCV ’03, pages
1470–, Washington, DC, USA. IEEE Computer Soci-
ety.
Thomee, B., Bakker, E. M., and Lew, M. S. (2010). Top-
surf: a visual words toolkit. In Proceedings of the in-
ternational conference on Multimedia, MM ’10, pages
1473–1476, New York, NY, USA. ACM.
Zhang, X., Li, Z., Zhang, L., Ma, W.-Y., and Shum, H.-
Y. (2009). Efficient indexing for large scale visual
search. In Computer Vision, 2009 IEEE 12th Inter-
national Conference on, pages 1103 –1110.
VISAPP2013-InternationalConferenceonComputerVisionTheoryandApplications
662