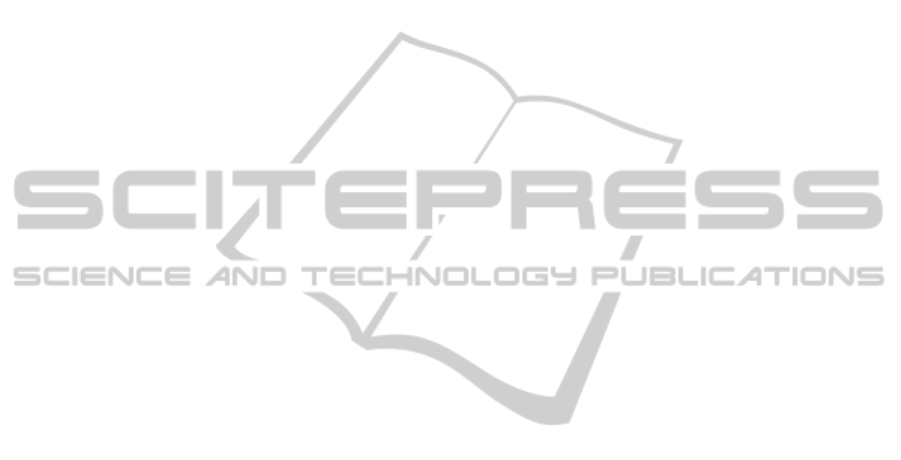
traffic data. A lot of effort is needed and a system
that enables graphical and real-time statistical anal-
ysis of traffic data is desired. The traffic police of-
ficers are interested in a real-time calculation of the
traffic because this can help them to place units ahead
where the masses are moving. Finally, the city plan-
ners consider traffic estimation as valuable informa-
tion for city designs.
During our preliminary evaluation, we got feed-
back from our interviewees. They were enthusiastic
about our tool like and they had even requested sim-
ilar improvements for their tools. They provided im-
provement ideas for our visualization. For instance,
the traffic management employees suggested that our
tool could be combined with the Oulu statistical mod-
els to estimate traffic data. The traffic police officers
could combine the information provided by our tool
with their accident database to get more insights about
traffic consequences. For the traffic city planner, it is
important to combine road traffic and pedestrian data
in real-time. This provides the opportunity to offer
optimal places for companies to set their outlets based
on the pedestrian traffic around the city.
5 CONCLUSIONS
We described our case study for a space-time visu-
alization of traffic data in the city of Oulu. We pre-
sented our exploratory visualization design by build-
ing a Web mashup, applying several visualization
techniques and validating it with three Oulu different
decision-makers. We argue that a proper combina-
tion of different visualization techniques that consider
multiple data dimensions, and an interactive synchro-
nization between space-time visualizations are suit-
able for traffic planning activities. This can sup-
port data analysis and evaluation of traffic data to re-
veal trends about interesting traffic events. In future
work, we will address some limitations that affect our
current implementation: (i) it needs to be combined
with city statistical models that adjusts traffic capac-
ity per road to support estimation of traffic data, (ii)
it currently refers only to traffic data but, according
to decision-makers’ needs, it can be easily extended
to pedestrian information, and (iii) real-time data is
needed to support real-time decision-making activi-
ties.
ACKNOWLEDGEMENTS
We would like to thank the traffic control authorities
of the city of Oulu and University of Oulu for the sup-
port during the development of this project.
REFERENCES
Bacon, J., Bejan, A., Beresford, A., Evans, D., Gibbens, R.,
and Moody, K. (2011). Using real-time road traffic
data to evaluate congestion. Dependable and Historic
Computing, pages 93–117.
Claramunt, C., Jiang, B., and Bargiela, A. (2000). A new
framework for the integration, analysis and visualisa-
tion of urban traffic data within geographic informa-
tion systems. Transportation Research Part C: Emerg-
ing Technologies, 8(1-6):167–184.
Ehmke, J., Meisel, S., and Mattfeld, D. (2010). Floating
car data based analysis of urban travel times for the
provision of traffic quality. Traffic data collection and
its standardization, pages 129–149.
Maciejewski, R., Rudolph, S., Hafen, R., Abusalah, A.,
Yakout, M., Ouzzani, M., Cleveland, W., Grannis, S.,
and Ebert, D. (2010). A visual analytics approach
to understanding spatiotemporal hotspots. Visualiza-
tion and Computer Graphics, IEEE Transactions on,
16(2):205 –220.
Shekhar, S., Lu, C., Liu, R., and Zhou, C. (2002). Cube-
view: a system for traffic data visualization. In In-
telligent Transportation Systems, 2002. Proceedings.
The IEEE 5th International Conference on, pages 674
– 678.
Shneiderman, B. (1996). The eyes have it: A task by
data type taxonomy for information visualizations. In
IEEE Symposium on Visual Languages, pages 336–
343.
Song, Y. and Miller, H. (2012). Exploring traffic flow
databases using space-time plots and data cubes.
Transportation, pages 1–20.
Van Wijk, J. and Van Selow, E. (1999). Cluster and calendar
based visualization of time series data. In Information
Visualization, 1999. (Info Vis ’99) Proceedings. 1999
IEEE Symposium on, pages 4 –9, 140.
Wang, X. (2005). Integrating gis, simulation models, and
visualization in traffic impact analysis. Computers,
Environment and Urban Systems, 29(4):471–496.
Wood, J., Dykes, J., Slingsby, A., and Clarke, K. (2007). In-
teractive visual exploration of a large spatio-temporal
dataset: Reflections on a geovisualization mashup. Vi-
sualization and Computer Graphics, IEEE Transac-
tions on, 13(6):1176 –1183.
Zhang, J., Wang, F., Wang, K., Lin, W., Xu, X., and Chen,
C. (2011). Data-driven intelligent transportation sys-
tems: A survey. Intelligent Transportation Systems,
IEEE Transactions on, (99):1–16.
TrafficVisualization-ApplyingInformationVisualizationTechniquestoEnhanceTrafficPlanning
557