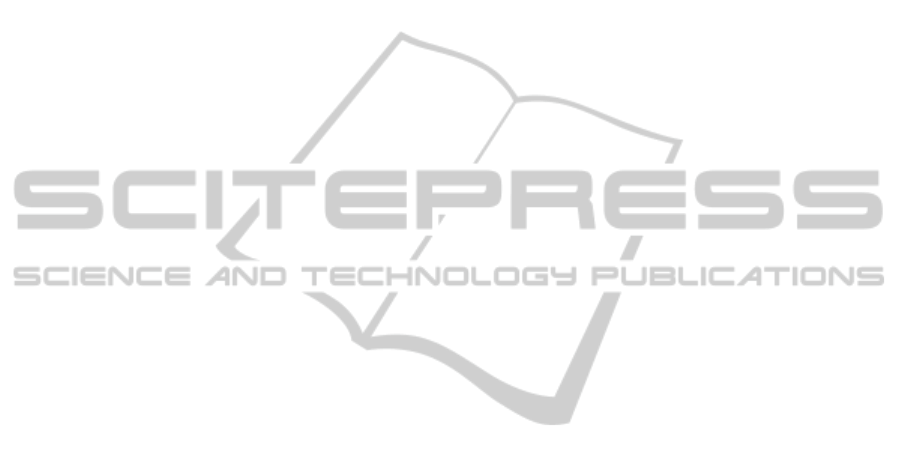
mented it, is rather global compared to point signa-
tures and line profiles and showed a relatively robust
performance. However, with suitable landmark local-
izations point signatures and line profiles outperform
curvature analyis. We used two approaches for auto-
mated landmark detection: Active Appearance Mod-
els and nose tip estimation by curvatures. The com-
bination of both lead to the best results. Line pro-
files showed weak contribution to the classification
process, if landmark positions are detected automat-
ically. Nevertheless, the results based on manually
defined regions are promising. Besides considera-
tions of making the line profiles more robust, a more
sophisticated approach for automated landmark de-
tection might be the most beneficial solution. Con-
strained AAMs (Cootes and Taylor, 2001) including
prior estimates of some shape point positions will
be investigated in order to improve the fitting of the
AAM. Curvature analysis and a-priori knowledge re-
lated to the anatomy of the face may be valuable for
the estimation of these prior positions.
ACKNOWLEDGEMENTS
We would like to thank the m&i Fachklinik Bad
Liebenstein (in particular Prof. Dr. med. Gustav
Pfeiffer, Eva Schillikowski) and Logop
¨
adische Praxis
Irina Stangenberger, who supported our work by giv-
ing valuable insights into rehabilitation and speech-
language therapy requirements and praxis. This work
is partially funded by the TMBWK ProExzellenz ini-
tiative, Graduate School on Image Processing and Im-
age Interpretation.
REFERENCES
Besl, P. and Jain, R. (1986). Invariant surface characteristics
for 3d object recognition in range images. Computer
Vision, Graphics, and Image Processing, 33(1):33–
80.
Chang, C.-C. and Lin, C.-J. (2011). LIBSVM:
A library for support vector machines. ACM
Transactions on Intelligent Systems and Technol-
ogy, 2:27:1–27:27. Software available at url-
http://www.csie.ntu.edu.tw/ cjlin/libsvm.
Chua, C.-S., Han, F., and Ho, Y.-K. (2000). 3d human face
recognition using point signature. In Proceedings of
the 4th Int. Automatic Face and Gesture Recognition
Conf., pages 233–238.
Chua, C. S. and Jarvis, R. (1997). Point signature: a new
representation for 3d object recognition. In Int. Jour-
nal of Computer Vision, volume 25, pages 63–85.
Colombo, A., Cusano, C., and Schettini, R. (2006). 3d face
detection using curvature analysis. Pattern Recogni-
tion, 39(3):444–455.
Cootes, T., Edwards, G., and Taylor, C. (2001). Active ap-
pearance models. IEEE Transactions on Pattern Anal-
ysis and Machine Intelligence, 23(6):681–685.
Cootes, T. and Taylor, C. (2001). Constrained active ap-
pearance models. In Proceedings of the 8th Int. Conf.
on Computer Vision, volume 1, pages 748–754.
Gebhard, A., Paulus, D., Suchy, B., and Wolf, S. (2000). A
system for diagnosis support of patients with facialis
paresis. Kl, 3/2000:40–42.
Grosse, M., Schaffer, M., Harendt, B., and Kowarschik, R.
(2011). Fast data acquisition for three-dimensional
shape measurement using fixed-pattern projection and
temporal coding. Optical Engineering, 50:100503.
Haase, D. and Denzler, J. (2011). Anatomical landmark
tracking for the analysis of animal locomotion in x-
ray videos using active appearance models. In Image
Analysis, volume 6688 of Lecture Notes in Computer
Science, pages 604–615.
Hartley, R. and Zisserman, A. (2000). Multiple view geom-
etry in computer vision. Cambridge University Press.
Hsu, C., Chang, C., and Lin, C. (2009). A prac-
tical guide to support vector classification.
TR available at http://www. csie. ntu. edu.
tw/ cjlin/papers/guide/guide. pdf.
Khoshelham, K. (2011). Accuracy analysis of kinect depth
data. In ISPRS Workshop Laser Scanning, volume 38.
Nakamura, K., Toda, N., Sakamaki, K., Kashima,
K., and Takeda, N. (2003). Biofeedback reha-
bilitation for prevention of synkinesis after facial
palsy. Otolaryngology–Head and Neck Surgery,
128(4):539–543.
Salomon, D. (2004). Data compression: the complete ref-
erence. Springer-Verlag New York Inc.
Viola, P. and Jones, M. (2004). Robust real-time face detec-
tion. Int. Journal of Computer Vision, 57(2):137–154.
Wang, J., Yin, L., Wei, X., and Sun, Y. (2006). 3d facial
expression recognition based on primitive surface fea-
ture distribution. Int. Conf. on Computer Vision and
Pattern Recognition, 2:1399–1406.
Wang, Y., Chua, C.-S., and Ho, Y.-K. (2002). Facial fea-
ture detection and face recognition from 2d and 3d
images. In Pattern Recognition Letters, volume 23,
pages 1191–1202.
Webb, A., Copsey, K., and Cawley, G. (2011). Statistical
pattern recognition. Wiley.
AutomatedClassificationofTherapeuticFaceExercisesusingtheKinect
565