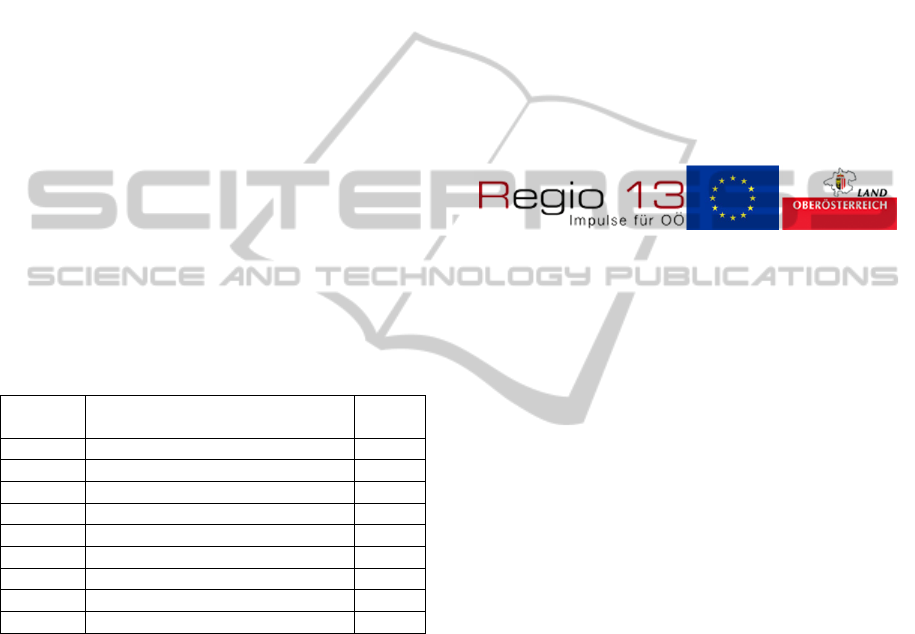
Concerning classification, for each feature the
prediction reliability is calculated based on
distributions with mean μ and variance δ calculated
as statistical average of all reference segmentations
and each particular anatomical structure to
distinguish. The reliability thereby indirectly
correlates with the error due to overlapping areas.
The overall prediction reliability is calculated via
stepwise integration over the entire feature value
range. The distributions of the anatomical structures
are normalized with respect to their overall
probability, i.e. statistical differences in anatomical
structure size.
Currently we evaluate 34 different feature types
for their prediction reliability when training an
anatomical topography model. Some of the most
notable are presented in Table 1 for analysis on
brainweb datasets. For construction of the feature
vector, a number of 10 of the best discriminating
features, should be selected. Despite choosing the
features with highest prediction reliability, it has to
be assured, that correlation within the feature vector
is low.
Table 1: Different types of features and their reliability for
classifying the different anatomical structure feature value
distributions.
feature
ID
description prec.
1 maximum intensity value 67.93
3 median intensity value 87.07
4 mean intensity value 88.43
5 quantile 25 intensity 90.37
7 anatomical structure size 82.34
21 surface-to-volume-ratio 82.29
22 entropy of intensities 75.40
23 energy of intensities 74.12
25 mean probability of intensities 78.46
5 CONCLUSIONS
A generic segmentation concept for fast model-
based adjustment to particular image segmentation
tasks and imaging modalities has been presented.
Hybrid pre-segmentation is perfectly applicable for
context-free pre-processing of arbitrary image data
for first region labelling. Correlation of the analyzed
texture and geometric features shows promising
results for future heuristics-based classification
according to pre-defined anatomical topography.
The discussed and continuously refined rapid
prototyping image processing chain is perfectly
applicable for fast and robust preparation of
reference segmentations for training the a priori
model.
ACKNOWLEDGEMENTS
Thanks to our medical partners from the Wagner-
Jauregg state mental hospital, Linz, Upper Austria,
at the institute for neuro-nuclear medicine headed by
Primarius Dr.Dr. Robert Pichler for providing
medical image data and for valuable discussion.
This research is part of the INVERSIA project
(http://inversia.fh-linz.at) which was funded by the
European Regional Development Fund (ERDF) in
cooperation with the Upper Austria state
government (Regio13).
REFERENCES
Beare, R., and Lehmann, G., 2006. The watershed
transform in ITK – discussion and new developments.
In Insight Journal.
Beyer, T., Schwenzer, N., Bisdas, S., Claussen C.D., and
Pichler, B.J., 2010. MR/PET – Hybrid Imaging for the
Next Decade. In MAGNETOM Flash 3/2010.
Felipe, J. C., 2003. Retrieval by content of medical images
using texture for tissue identification. In CBMS.
Goldberg, D. E., 1989. Genetic Algorithms in Search
Optimization and Machine Learning. In Addison-
Wesley Professional.
Kwan, R. K.-S., Evans, A. C., Pike, G.B., 1999. MRI
simulation-based evaluation of image-processing and
classification methods. In IEEE Transactions on
Medical Imaging 18(11):1085-1097.
Rechenberg, I., 1973. Evolutionsstrategie-Optimierung
technischer Systeme nach Prinzipien der biologischen
Evolution. In Frommann-Holzboog Verlag, Stuttgart,
Germany.
Ritter, F., 2007. Visual Programming for Prototyping of
Medical Applications. In IEEE Visualization
workshop.
Sato, Y. S., Atsumi, H., Koller, T., Gerig, G., Yoshida, S.,
Kikinis, R., 1998. Three-dimensional multi-scale line
filter for segmentation and visualization curvilinear
structures in medical images. In Medical Image
Analysis 2 (2), 143-168.
Zwettler, G., Backfrieder, W., Swoboda, R., Pfeifer, F.,
2009. Fast Fully-automated Model-driven Liver
Segmentation Utilizing Slicewise Applied Levelsets
on Large CT Data. In Proc. of the 21st European
Modeling and Simulation Symposium, 161-166.
Generic3DSegmentationinMedicinebasedonaSelf-learningTopologicalModel
107