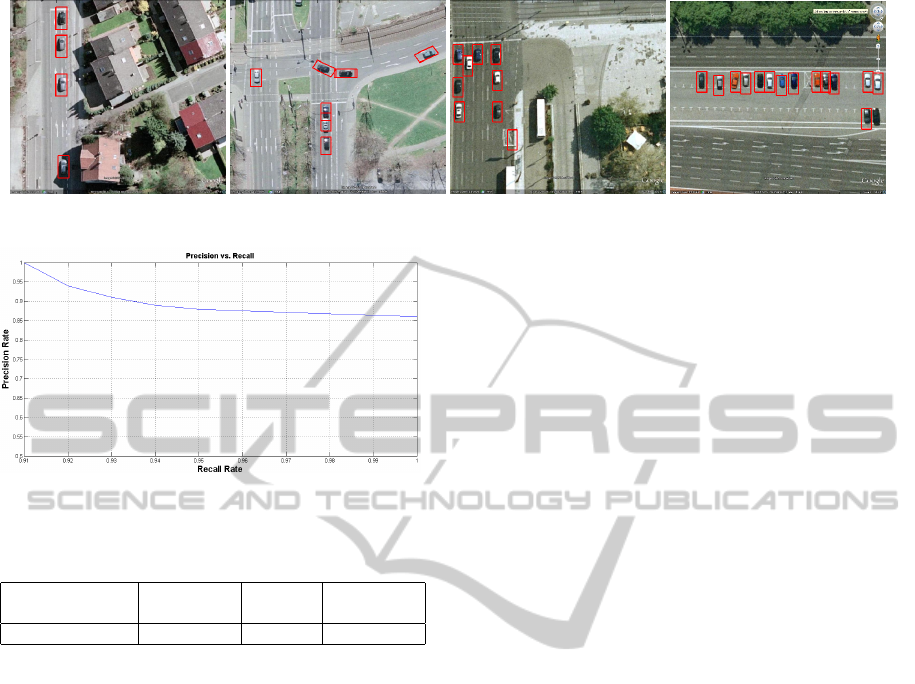
Figure 8: Shows some more results from Google Earth images.
Figure 7: Illustrates the performance of the two-stage algo-
rithm on Google Earth data set.
Table 2: Performance of our two-stage approach
No. of images
processed
No. of cars Overall
RR
Overall PR
36 374 90% 94%
The overall recall and precision rate gives a clearer picture
of an impressive performance obtained through our
approach.
two strong classifiers one after the other. In the pro-
cess, we improve the detection rate of the first clas-
sifier in order not to miss objects in the first stage
and improve the precision of the second classifier.
Hence, we were able to achieve a high recall rate and
with very high precision rate. We have achieved very
good results in terms of accuracy, however, to make
it a robust system, more work in this direction is re-
quired. Knowing that the proposed system performs
well, we would be interested in a faster implementa-
tion of sophisticated approach such as part-based de-
tection methods so that we are able to detect objects in
large images in real time. Besides, we expect to de-
velop a more efficient rotation-invariance scheme to
be used in the first stage.
REFERENCES
Chang, C.-C. and Lin, C.-J. (2001). LIBSVM: a library for
support vector machines.
Cortes, C. and Vapnik, V. (1995). Support vector networks.
In Machine Learning, volume 20, pages 273–297.
Dalal, N. and Triggs, B. (2005). Histograms of oriented
gradients for human detection. In International Con-
ference on Computer Vision & Pattern Recognition,
volume 2, pages 886–893.
Felzenszwalb, P., McAllester, D., and Ramanan, D. (2008).
A discriminatively trained, multiscale, deformable
part model. In Computer Vision and Pattern Recog-
nition, 2008. CVPR 2008. IEEE Conference on.
Felzenszwalb, P. F., Girshick, R. B., McAllester, D., and
Ramanan, D. (2010). Object detection with discrimi-
natively trained part-based models. IEEE Trans. Pat-
tern Anal. Mach. Intell., 32(9).
Fischler, M. A. and Elschlager, R. A. (1973). The repre-
sentation and matching of pictorial structures. IEEE
Trans. Comput., 22.
Han, F., Shan, Y., Cekander, R., Sawhney, H., and Kumar,
R. (2006). A two-stage approach to people and vehicle
detection with HOG-based SVM. In The 2006 Perfor-
mance Metrics for Intelligent Systems Workshop.
Hinz, S. (2003). Detection and counting of cars in aerial
images. In International Conference on Image Pro-
cessing.
Kembhavi, A., Harwood, D., and Davis, L. (2011). Vehicle
detection using partial least squares. Pattern Analy-
sis and Machine Intelligence, IEEE Transactions on,
33(6):1250 –1265.
Meng, X., Lin, J., and Ding, Y. (2012). An extended HOG
model: SCHOG for human hand detection. In Systems
and Informatics (ICSAI), 2012 International Confer-
ence on.
Monzo, D., Albiol, A., Albiol, A., and Mossi, J. (2011).
Color HOG-EBGM for face recognition. In Im-
age Processing (ICIP), 2011 18th IEEE International
Conference on.
Nguyen, T., Grabner, H., Bischof, H., and Gruber, B.
(2007). On-line boosting for car detection from aerial
images. In International Conference on Research, In-
novation and Vision for the Future.
Ruskone, R., Guigues, L., Airault, S., and Jamet, O.
(1996). Vehicle detection on aerial images: A struc-
tural approach. In International Conference on Pat-
tern Recognition, pages 900–904.
Wang, Q. J. and Zhang, R. B. (2008). LPP-HOG: A
new local image descriptor for fast human detection.
In Knowledge Acquisition and Modeling Workshop,
2008. KAM Workshop 2008. IEEE International Sym-
posium on.
Zhao, T. and Nevatia, R. (2001). Car detection in low res-
olution aerial image. In International Conference on
Computer Vision.
VISAPP2013-InternationalConferenceonComputerVisionTheoryandApplications
686