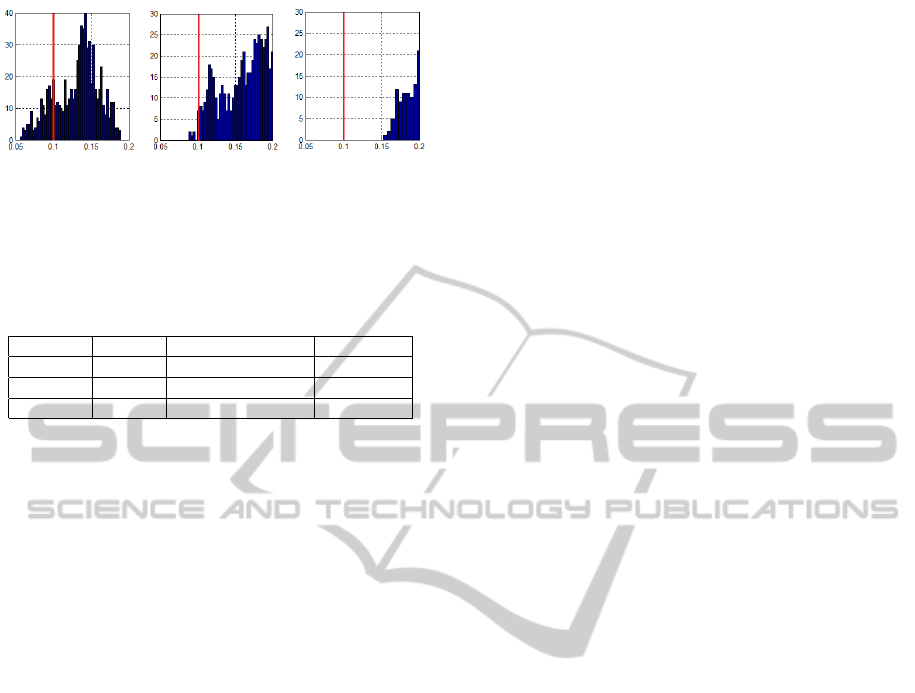
(a) .1 ≤ µ−σ. (µ=.13,
σ=.026).
(b) .1 ≤ µ−2σ. (µ=.17,
σ=.034).
(c) .1 ≤ µ−3σ. (µ=.30,
σ=.063).
Figure 5: The LI (minµ(L
B
(s) subject to .1≤L
B
) is modeled
with three values of a (from left to right: a=1, 2, and 3).
Table 1: Comparative of optimization approaches against
our statistical technique.
Method Tests/s Total time (min) Speed-Up
SIL 2083 4 -
LRR+ 59 141 35
LRR 8 1041 260
6 CONCLUSIONS AND FUTURE
WORK
In this paper is presented a new methodology for
achieving LI for inverse lighting problems. Our ap-
proach is based on the use of µ and σ as statistical pa-
rameters for the lighting values. Using a low-rank for-
mulation, we demonstrate that µ and σ for L
B
can be
computed in O(n) and O(n + e
2
). This result allows
to perform thousands of global illumination evalua-
tion on a desktop PC, reducing drastically the over-
all optimization time required. We believe that this
technique could open a new avenue in the search for
optimal inverse lighting solutions. The results shown
could lead to the use of the method in more complex
scenes with more elaborated lighting intentions. Re-
lated to further development, more effort should be
focus on an automatic parametrization of the LI.
ACKNOWLEDGEMENTS
This work was partially funded by Programa de
Desarrollo de las Ciencias B
´
asicas (Uruguay) and
by grant TIN2010-20590-C02-02 from Ministerio de
Ciencia e Innovaci
´
on (Spain).
REFERENCES
Canavos, G. (1984). Applied probability and statistical
methods. Little, Brown.
Cassol, F., Schneider, P. S., Franc¸a, F. H., and Neto, A.
J. S. (2011). Multi-objective optimization as a new
approach to illumination design of interior spaces.
Building and Environment, 46(2):331 – 338.
Castro, F., del Acebo, E., and Sbert, M. (2012). Energy-
saving light positioning using heuristic search. En-
gineering Applications of Artificial Intelligence,
25(3):566 – 582.
Cohen, M., Wallace, J., and Hanrahan, P. (1993). Radiosity
and realistic image synthesis. Academic Press Profes-
sional, Inc., San Diego, CA, USA.
Contensin, M. (2002). Inverse lighting problem in radiosity.
Inverse Problems in Engineering, 10(2):131–152.
Fern
´
andez, E. (2009). Low-rank radiosity. In Rodr
´
ıguez,
O., Ser
´
on, F., Joan-Arinyo, R., and J. Madeiras,
J. Rodr
´
ıguez, E. C., editors, Proceedings of the IV
Iberoamerican Symposium in Computer Graphics,
pages 55–62. Sociedad Venezolana de Computaci
´
on
Gr
´
afica, DJ Editores, C.A.
Fern
´
andez, E. and Besuievsky, G. (2012). Inverse lighting
design for interior buildings integrating natural and ar-
tificial sources. Computers & Graphics, 36(8):1096–
1108.
Fern
´
andez, E., Ezzatti, P., Nesmachnow, S., and Be-
suievsky, G. (2012). Low-rank radiosity using sparse
matrices. In Proceedings of GRAPP2012, pages 260–
267.
Hansen, P. and Mladenovic, N. (2001). Variable neighbor-
hood search: Principles and applications. European
Journal of Operational Research, 130(3):449–467.
Kawai, J. K., Painter, J. S., and Cohen, M. F. (1993). Ra-
dioptimization - goal based rendering. In ACM SIG-
GRAPH 93, pages 147–154, Anaheim, CA.
Patow, G. and Pueyo, X. (2003). A survey of inverse render-
ing problems. Computer Graphics Forum, 22(4):663–
688.
Russell, S. (2008). The Architecture of Light: Architectural
Lighting Design, Concepts and Techniques: a Text-
book of Procedures and Practices for the Architect,
Interior Designer and Lighting Designer. Concept-
nine.
Russell, S. J. and Norvig, P. (2003). Artificial Intelligence:
A Modern Approach. Pearson Education, 2 edition.
Schneider, P. S., Mossi, A. C., Franca, F. H. R., de Sousa,
F. L., and da Silva Neto, A. J. (2009). Application of
inverse analysis to illumination design. Inverse Prob-
lems in Science and Engineering, 17(6):737–753.
Schoeneman, C., Dorsey, J., Smits, B., Arvo, J., and Green-
berg, D. (1993). Painting with light. In Proceedings of
the 20th annual conference on Computer graphics and
interactive techniques, ACM SIGGRAPH 93, pages
143–146, New York, NY, USA. ACM.
GRAPP2013-InternationalConferenceonComputerGraphicsTheoryandApplications
190