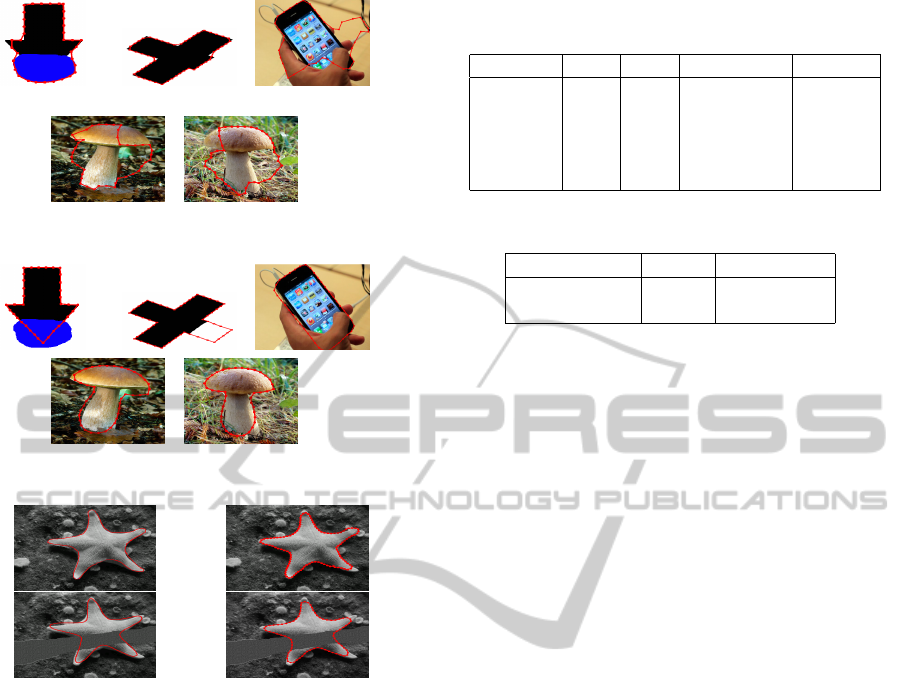
Figure 3: Results without shape priors.
Figure 4: Results with our Fourier based shape priors.
(a) DBSS (b) our method
Figure 5: Results by (DBSS) method (a) and by our method
(b).
we compare our method with another state-of-the-art
method which uses the distance-based shape statistics
(DBSS) (Charpiat et al., 2007) as shape priors.
Results presented in Table 2,clearly indicates that
our method is more accurate as compared to (DBSS).
6 CONCLUSIONS
In this paper, a new model with translation, scale, ro-
tation and starting point invariant shape priors for ex-
plicit active contour has been presented. Calculation
of shape based energy was entirely performed in the
descriptors space, i.e. there is no need to reconstruct
the prior shape during evolution of active contours,
which is a gain in terms of computation time. Visual
and numerical evaluations on both synthetic and real
images have shown that our method greatly improves
the segmentation results, even in presence of occlu-
sion and incomplete shapes. In the near future, we
Table 1: Segmentation results using Pratt criterion with and
without shape priors (SP).
Image k ζ
max
Without SP With SP
Arrow 0.03 1.5 28.09 82.86
Cross 0.05 2.5 20.64 85.03
Phone 0.03 1.5 16.09 46.85
Boletus1 0.03 1.5 10.08 12.40
Boletus2 0.02 1.2 13.69 15.96
Table 2: Comparison of segmentation results.
Image DBSS Our method
Non-occluded 23.53 29.00
Occluded 12.57 13.62
would also like to test our method on medical images
and video data. The implementation for the level set
method will be considered as well.
REFERENCES
Bartolini, I., Ciaccia, P., and Patella, M. (2005). Warp: Ac-
curate retrieval of shapes using phase of fourier de-
scriptors and time warping distance. IEEE Transac-
tions on Pattern Analysis and Machine Intelligence,
27(1):142–147.
Caselles, V., Kimmel, R., and Sapiro, G. (1995). Geodesic
active contours. International Journal of Computer
Vision, 22(1):61–79.
Charmi, M. A., Derrode, S., and Ghorbel, F. (2008).
Fourier-based geometric shape prior for snakes. Pat-
tern Recognition Letters, 29(7):897–904.
Charpiat, G., Faugeras, O. D., and Keriven, R. (2007).
Shape statistics for image segmentation with prior. In
CVPR.
Cohen, L. D. and Cohen, I. (1991). Finite element methods
for active contour models and balloons for 2d and 3d
images. IEEE Transactions on Pattern Analysis and
Machine Intelligence, 15:1131–1147.
Cremers, D., Tischhäuser, F., Weickert, J., and Schnörr, C.
(2002). Diffusion snakes: Introducing statistical shape
knowledge into the mumford-shah functional. Inter-
national Journal of Computer Vision, 50(3):295–313.
Fabbri, R., Costa, L. D. F., Torelli, J. C., and Bruno, O. M.
(2008). 2d euclidean distance transform algorithms:
A comparative survey. ACM Computing Surveys,
40(1):2:1–2:44.
Kass, M., Witkin, A., and Terzopoulos, D. (1988). Snakes:
Active contour models. International Journal of Com-
puter Vision, 1(4):321–331.
Mille, J., Boné, R., Makris, P., and Cardot, H. (2006).
Greedy algorithm and physics-based method for ac-
tive contours and surfaces: A comparative study. In
ICIP, pages 1645–1648. IEEE.
Pratt, W. K., Faugeras, O. D., , and Gagalowicz, A.
(1978). Visual discrimination of stochastic texture
AnActiveContourModelwithImprovedShapePriorsusingFourierDescriptors
475