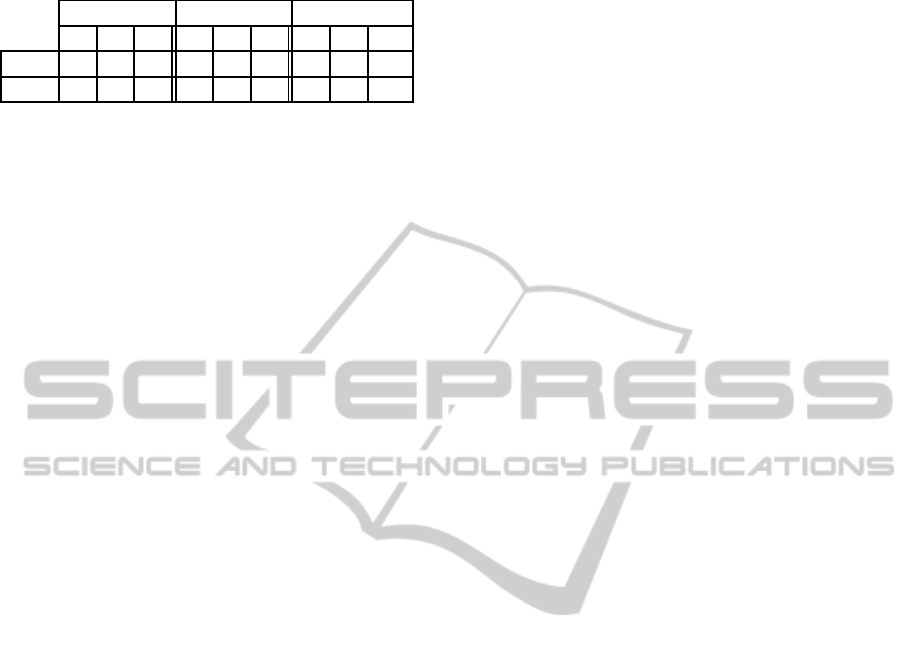
Table 5: Separator detection after recurrence classification.
Audio Visual Audio
S
Visual
P R F1 P R F1 P R F1
Games 0.84 0.76 0.79 0.78 0.65 0.71 0.85 0.78 0.82
M&N 0.84 0.52 0.65 0.74 0.47 0.57 0.85 0.62 0.72
cation accuracy in the previous section and we evalu-
ated the results using the separators ground-truth. The
results are presented in Table 5.
The general tendency after classification is an im-
provementof precision at the cost of a decrease for the
recall. This means that recurrences that are not sep-
arators are filtered out but in the same time some of
the true separators too. This could be a consequence
of the noise induced by the different technologies that
we used when computing the attributes. Another rea-
son, equally important, could be the erroneous choice
of attributes that are not as relevant for our task as we
might have expected.
Nevertheless, if we take a close look in Table 5 for
the case of visual recurrences the classification does
not influence the results significantly. The most im-
portant changes appear for the case of audio recur-
rences. In this manner, when considering the games,
there is an important increase of the precision with
77% after classification, with a decrease in the recall
of 19%. However the F1 score that combines the pre-
cision and recall measures is superior with 66%. For
the magazines and news the variation of the precision
and recall is around 40%, with an increase of the F1
score of only 10%.
5 CONCLUSIONS
In this paper we proposed an approach for the detec-
tion and classification of audio and visual recurrences
based on decision trees. The idea was to compute the
performance of such system on the detection of ”sep-
arators”, that are at the root of an automatic recurrent
TV program structuring system.
Experiments showed that our main assumption,
that separators are repeated and could be detected as
recurrences is validated. When classifying these re-
currences, using decision trees trained with the 3 pro-
posed criteria, the performances regarding the classi-
fication accuracy are very similar. Moreover, for the
case of visual recurrences, these do not exceed signif-
icantly the naive classifier meaning that in this case a
classification step in not really necessary.
The evaluation of the whole solution, showed that
a lot of false alarms are filtered out (especially for au-
dio recurrences) but with them a part of the separators
too. This resulted in an increase of the precision but at
the cost of a decrease in the recall. However, globally,
the F-measure for the audio case is better after per-
forming the classification step. For the visual-based
approach, results are not significantly influenced.
In perspective, we intend to extend further the use
of decision trees by trying combinations of attributes
in the training stage. We would also like to consider
the use of another type of classifier, such as the SVMs.
This would allow us to compare the results and con-
clude if the actual results are more influenced by the
limitations of decision trees or by the attributes we
have defined.
REFERENCES
Abduraman, A. E., Berrani, S.-A., and Merialdo, B.
(2011a). Audio recurrence contribution to a video-
based tv program structuring approach. In IEEE Int.
Symposium on Multimedia, Dana Point, CA, USA.
Abduraman, A. E., Berrani, S.-A., Rault, J.-B., and Blouch,
O. L. (2011b). From audio recurrences to tv program
structuring. In 4th Int. Workshop on Automated Me-
dia Analysis and Production for Novel TV Services,
Scottsdale, Arizona, USA.
Ben, M. and Gravier, G. (2011). Unsupervised mining of
audiovisually consistent segments in videos with ap-
plication to structure analysis. In IEEE Int. Conf. on
Multimedia and Exhibition, Barcelone, Espagne.
Berrani, S.-A., Manson, G., and Lechat, P. (2008). A
non-supervised approach for repeated sequence detec-
tion in tv broadcast streams. Image Communication,
23(7):525–537.
Chaisorn, L., Chua, T.-S., and Lee, C.-H. (2003). A multi-
modal approach to story segmentation for news video.
World Wide Web, 6(2):187–208.
Claude Barras, Xuan Zhu, S. M. and Gauvain, J.-L. (2006).
Multistage speaker diarization of broadcast news.
IEEE Trans. On Audio, Speech and Language Pro-
cessing, 14(5):1505–1512.
Eickeler, S., Wallhoff, F., Iurgel, U., and Rigoll, G. (2001).
Content based indexing of images and video using
face detection and recognition methods. In IEEE Int.
Conf. on Acoustics, Speech and Signal Processing,
Salt Lake City, Utah, USA.
Garcia, C. and Delakis, M. (2004). Convolutional face
finder: A neural architecture for fast and robust face
detection. IEEE Trans. on Pattern Analysis and Ma-
chine Intelligence, 26(11):1408–1423.
Goela, N., Wilson, K., Niu, F., and Divakaran, A.
(2007). An svm framework for genre-independent
scene change detection. In IEEE Int. Conf. on Mul-
timedia and Expo, Beijing, China.
Herley, C. (2006). Argos: automatically extracting repeat-
ing objects from multimedia streams. In IEEE Trans.
on Multimedia, vol. 8, pages 115–129.
Audio/VisualRecurrencesandDecisionTreesforUnsupervisedTVProgramStructuring
707