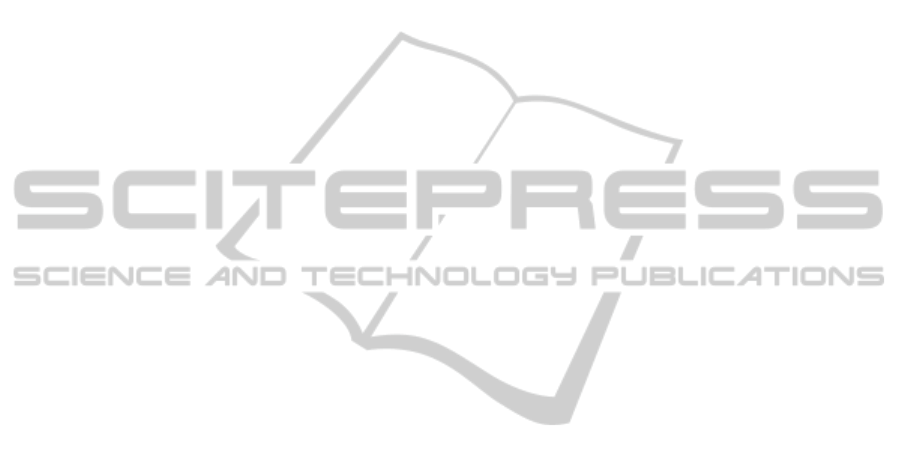
and Dynamic Scenes Using Fine-Grain Top-Down Vi-
sual Attention. In ICVS 5008, LNCS, pages 3 – 12, San-
torini, Greece.
Backer, M., T
¨
unnermann, J., and Mertsching, B. (2012).
Parallel k-Means Image Segmentation Using Sort, Scan
& Connected Components on a GPU. In FTMC-III,
LNCS.
Belardinelli, A., Pirri, F., and Carbone, A. (2009). Atten-
tion in cognitive systems. chapter Motion Saliency Maps
from Spatiotemporal Filtering, pages 112–123. Springer,
Berlin - Heidelberg.
Blanz, V., Sch
¨
olkopf, B., B
¨
ulthoff, H., Burges, C., Vapnik,
V., and Vetter, T. (1996). Comparison of View-based Ob-
ject Recognition Algorithms Using Realistic 3D Models.
In von der Malsburg, C., von Seelen, W., Vorbr
¨
uggen,
J., and Sendhoff, B., editors, Artificial Neural Networks,
volume 1112 of LNCS, pages 251–256. Springer, Berlin
- Heidelberg.
Borji, A. and Itti, L. (2012). State-of-the-Art in Visual At-
tention Modeling. Accepted for: IEEE TPAMI.
Hilkenmeier, F., T
¨
unnermann, J., and Scharlau, I. (2009).
Early Top-Down Influences in Control of Attention: Ev-
idence from the Attentional Blink. In KI 2009: Advances
in Artificial Intelligence. Proceeding of the 32nd Annual
Conference on Artificial Intelligence.
Hou, X. and Zhang, L. (2007). Saliency Detection: A Spec-
tral Residual Approach. In IEEE CVPR, pages 1–8.
Itti, L. and Koch, C. (2001). Feature Combination Strategies
for Saliency-Based Visual Attention Systems. Journal of
Electronic Imaging, 10(1):161–169.
Itti, L., Koch, C., and Niebur, E. (1998). A Model of
Saliency-Based Visual Attention for Rapid Scene Anal-
ysis. IEEE TPAMI, 20(11):1254–1259.
Jian Li, Martin Levine, X. A. and He, H. (2011). Saliency
Detection Based on Frequency and Spatial Domain Anal-
yses. In BMVC, pages 86.1–86.11. BMVA Press.
Kalal, Z., Matas, J., and Mikolajczyk, K. (2009). Online
Learning of Robust Object Detectors During Unstable
Tracking. On-line Learning for Computer Vision Work-
shop.
Koch, C. and Ullman, S. (1985). Shifts in Selective Atten-
tion: Towards the Underlying Neural Circuitry. Human
Neurobiology, 4:219–227.
Kotth
¨
auser, T. and Mertsching, B. (2010). Validating Vision
and Robotic Algorithms for Dynamic Real World Envi-
ronments. In Ando, N., Balakirsky, S., Hemker, T., Reg-
giani, M., and Stryk, O., editors, Simulation, Modeling,
and Programming for Autonomous Robots, volume 6472
of LNCS, pages 97–108. Springer, Berlin - Heidelberg.
Kouchaki, Z. and Nasrabadi, A. M. (2012). A Nonlinear
Feature Fusion by Variadic Neural Network in Saliency-
based Visual Attention. VISAPP, pages 457–461.
Li, W., Pi
¨
ech, V., and Gilbert, C. D. (2004). Perceptual
Learning and Top-Down Influences in Primary Visual
Cortex. Nature Neuroscience, 7(6):651–657.
Navalpakkam, V. and Itti, L. (2006). An Integrated Model
of Top-Down and Bottom-Up Attention for Optimal Ob-
ject Detection. In IEEE CVPR, pages 2049–2056, New
York, NY.
Oliva, A. and Torralba, A. (2006). Building the Gist of a
Scene: The Role of Global Image Features in Recogni-
tion. In Progress in Brain Research, page 2006.
Torralba, A., Oliva, A., Castelhano, M. S., and Hender-
son, J. M. (2006). Contextual Guidance of Eye Move-
ments and Attention in Real-world Scenes: The Role of
Global Features in Object Search. Psychological Review,
113(4):766–786.
Treisman, A. M. and Gelade, G. (1980). A Feature-
Integration Theory of Attention. Cognitive psychology,
12(1):97–136.
T
¨
unnermann, J. and Mertsching, B. (2012). Continuous
Region-Based Processing of Spatiotemporal Saliency. In
VISAPP, pages 230 – 239.
Wischnewski, M., Belardinelli, A., Schneider, W. X., and
Steil, J. J. (2010). Where to Look Next? Combining
Static and Dynamic Proto-objects in a TVA-based Model
of Visual Attention. Cognitive Computation, pages 326–
343.
Wolfe, J. M. and Horowitz, T. S. (2004). What Attributes
Guide the Deployment of Visual Attention and How Do
They Do It? Nature Reviews Neuroscience, 5(6):495–
501.
Yarbus, A. L. (1967). Eye Movements and Vision. Plenum.,
New York, NY.
Top-DownVisualAttentionwithComplexTemplates
377