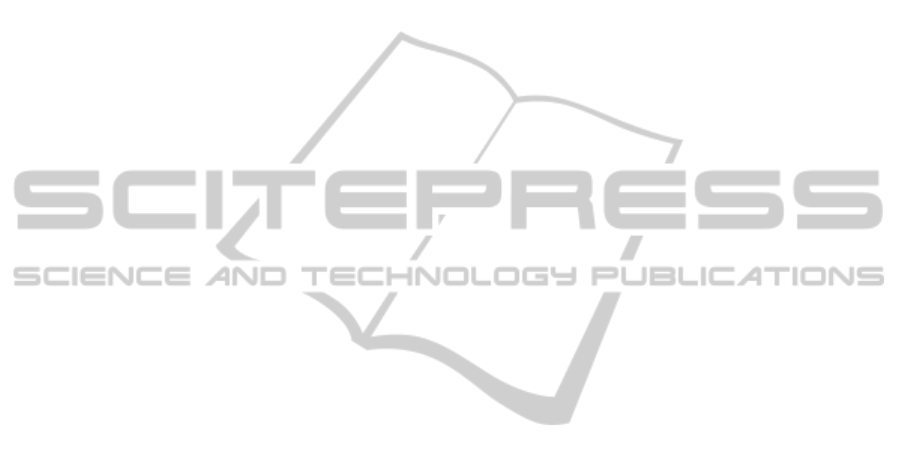
of the blur caused by defocusing is unchanging be-
fore and after the camera rotations. Our method uses
a reference image and a blurred image for processing,
and both have the same defocusing-blur, hence our
method can cancel automatically the defocusing-blur.
Next, we explain the advantages of our method
over the depth-from-focus method (Nayar and Nak-
agawa, 1994). In the depth-from-focus method, fo-
cus should be varied accurately in several different
ways. However, in our method, a camera has only
to be rotated randomly, i.e. accurate control of a cam-
era is not required. Since, in our future method, the
deviation of the random camera rotations will be es-
timated from the observed images, there will be no
need to know the deviation before processing. An-
other advantage of our motion-blur scheme is that a
lot of still images having no motion-blur, which are
averaged to generate a motion-blur image, can be ob-
served and processed if needed. Depth recoverybased
on either motion-blur or defocusing-blur is fitted for
sufficiently fine textures, hence surfaces with origi-
nally blurred texture cannot be handled. However, if
camera rotations are adopted, we can deal with such a
smooth texture using the differential method (Tagawa,
2010). Hence, we can adaptively recover the depth by
switching the integral method proposed in this study
and the differential method according to the fineness
of the surface texture.
6 CONCLUSIONS
We propose a new method to recover a depth map
using the camera rotations imitating fixational eye
movements, in particular tremor-related movements.
The proposed method can compute a depth map di-
rectly from blurred image. In this study, we approx-
imate the motion-blurred image by averaging a huge
number of images artificially generated by the random
camera rotations, and we are yet to examine the ef-
fectiveness of our method by real image experiments
using an actual imaging system in the future work.
The simulations in this study did not consider lighting
condition and reflection characteristics of an imaging
target. Especially, to examine an influence of spec-
ular reflection components, at first numerical evalua-
tions have to be done throughly, and subsequent ex-
periments are strongly required.
An outline of a depth map can be recovered by
the method in the simulations, but its accuracy may
be insufficient. The proposed method cannot be used
for small image motions relative to a texture pattern.
For this case, the differential formed method (Tagawa,
2010) is effective. On the other hand, from the funda-
mental principle of our method that the image blur is
used for depth recovery, the spatial resolution of the
recovered depth is not so high, no matter how careful
we are on selecting the camera motion size. For this
case, we can use the results from the proposedintegral
method as an initial depth for the method (Tagawa
et al., 2008) (Tagawa and Naganuma, 2009). Hence,
we plan on unifying those methods to deal with var-
ious situations. Especially to combine the differen-
tial method (Tagawa, 2010) and the integral method
in this paper, we have to develop a suitable segmenta-
tion method, which divides observed images into fine
texture regions and rough texture regions, taking into
account the size of camera rotations. Additionally, to
use both of the differential and the integral methods
simultaneously, the motion-blurred image has to be
generated by averaging many captured images with-
out motion blur instead of simply capturing analog
blur image using the suitable exposure time. For the
case, lesser number of the image used for averaging is
desirable for computational costa and real-time opera-
tion, but this requirement cannot realize the ideal mo-
tion blur supposed in this study. Therefore, we have
to improve the integral method in this study to give a
good performance using such the insufficient motion
blur.
REFERENCES
Bruhn, A. and Weickert, J. (2005). Locas/kanade meets
horn/schunk: combining local and global optic flow
methods. Int. J. Comput. Vision, 61(3):211–231.
Dempster, A. P., Laird, N. M., and Rubin, D. B. (1977).
Maximum likelihood from incomplete data. J. Roy.
Statist. Soc. B, 39:1–38.
Gammaitoni, L., Hanggi, P., Jung, P., and Marchesoni, F.
(1998). Stochastic resonance.
Greenwood, P. E., Ward, L. M., and Wefelmeyer, W. (1999).
Statistical analysis of stochastic resonance in a simple
setting. Physical Rev. E, 60:4687–4696.
Hongler, M.-O., de Meneses, Y. L., Beyeler, A., and Jacot,
J. (2003). The resonant retina: exploiting vibration
noise to optimally detect edges in an image. IEEE
Trans. Pattern Anal. Machine Intell., 25(9):1051–
1062.
Horn, B. P. and Schunk, B. (1981). Determining optical
flow. Artif. Intell., 17:185–203.
Jazwinski, A. (1970). Stochastic processes and filtering the-
ory. Academic Press.
Martinez-Conde, S., Macknik, S. L., and Hubel, D. (2004).
The role of fixational eye movements in visual percep-
tion. Nature Reviews, 5:229–240.
Nayar, S. K. and Nakagawa, Y. (1994). Shape from focus.
IEEE Trans. Pattern Anal. Machine Intell., 16(8):824–
831.
DirectDepthRecoveryfromMotionBlurCausedbyRandomCameraRotationsImitatingFixationalEyeMovements
185