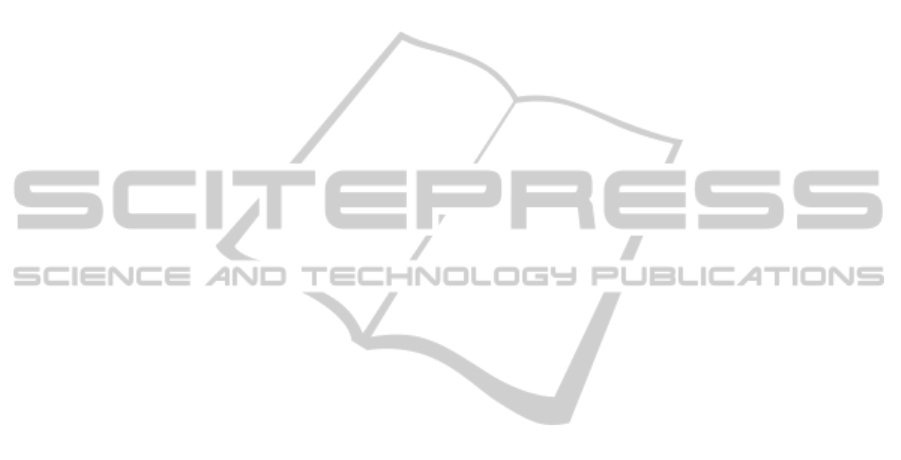
In the same conditions, training time is also de-
creased by our approach. The training takes 22 hours
for Tuzel et al. approach while it takes 9 hours for our
approach.
Note finally that the clustering in tangent space
provide better results for first cascade levels training,
but after few levels, it becomes less precise. This can
be explained by the fact that at the first level,negative
samples are densly regrouped. The computed mean
for tangent space projection is significant. After few
level training, and removing correct classified neg-
atives, the remaining negatives became sparse and
computing a mean on sparse samples make it less sig-
nicative, the projection to tangent space is not suit-
able.
5 CONCLUSIONS
We have proposed an approach to optimize people de-
tection using covariance descriptors. This approach
consists in clustering negative data before training to
obtain better classifier structure. The resulting de-
tector is faster that original one and was trained in
shorter time. The experimental results on a challeng-
ing dataset validate our approach.
REFERENCES
Comaniciu, D. and Meer, P. (2002). Mean shift: A robust
approach toward feature space analysis. IEEE Trans.
Pattern Anal. Machine Intell., 24:603619.
Dalal, N. and Triggs, B. (2005). Histograms of oriented
gradients for human detection. In IEEE Conf. Comp.
Vision and Pattern Recognition (CVPR).
Dalal, N., Triggs, B., and Schmid, C. (2006). Human de-
tection using oriented histograms of flow and appear-
ance. In Europe Conf. Comp. Vision (ECCV), volume
II, pages 428441.
Friedman, J., Hastie, T., and Tibshira, R. (2000). Additive
logistic regression: a statisticalview of boosting. Ann.
Statist., 23(2):337C407.
Mikolajczyk, K., Schmid, C., and Zisserman, A. (2004).
Human detection based on a probabilistic assembly of
robust part detectors. In Europe Conf. Comp. Vision
(ECCV), volume I, pages 6981.
Mu, Y., Yan, Y., Liu, Y., Huang, T., and Zhou, B. (2008).
Discriminative local binary patterns for human detec-
tion in personal album. In CVPR 2008, pages 18.
Papageorgiou, P. and Poggio, T. (2000). A trainable sys-
tem for object detection. Int. J. of Computer Vision,
38(1):1533.
Pennec, X., Fillard, P., and Ayache, N. (2006). A rieman-
nian framework for tensor computing. Int. Journal of
Comp. Vision, 66(1):4166.
Schwartz, W., Kembhavi, A., Harwood, D., and Davis, L.
(2009). Human detection using partial least squares
analysis. In ICCV.
Simard, P., Bottou, L., Haffner, P., and LeCun, Y. (1998).
Boxlets: A fast convolution algorithm for signal pro-
cessing and neural networks. Proc. Conf. Advances
in Neural Information Processing Systems II, pp. 571-
577.
Tuzel, O., Porikli, F., and Meer, P. (2007). Human detection
via classification on riemannian manifolds. In IEEE
Conf. Comp. Vision and Pattern Recognition (CVPR).
Viola, P. and Jones, M. (2001). Rapid object detection
using a boosted cascade of simple features. Proc.
IEEE Conf. Computer Vision and Pattern Recognition
(CVPR 01), vol. 1, pp. 511-518.
Viola, P., Jones, M., and Snow, D. (2006). Detecting pedes-
trians using patterns of motion and appearance. In Eu-
rope Conf. Comp. Vision (ECCV), volume II, pages
589600.
Yao, J. and Odobez, J. (2008). Fast human detection from
videos using covariance feature. In: ECCV 2008 Vi-
sual Surveillance Workshop.
VISAPP2013-InternationalConferenceonComputerVisionTheoryandApplications
826