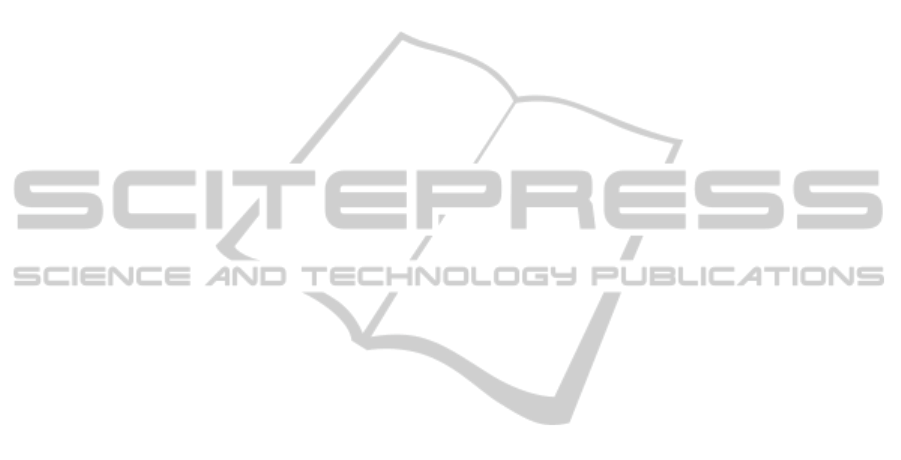
2 METHOD DESCRIPTION
The block diagram of Figure 1 shows how the steps
are organized. The UAV reaches (using GPS) the
search space (the neighborhood area of the reference
image) by GPS and then activate the vision system.
Initially, the canopy pattern is eliminated via
multiresolution analysis of images based on wavelet
transform. In this case, at a larger scale it is possible
to extract only the salient features and suppress the
nonrelevant ones (Fonseca et al, 2008). This is
necessary, otherwise during feature extraction
hundreds of useless features, related to leaves,
branches, and other small image elements related to
a typical canopy texture, will be found.
We use multiresolution analysis to adapt the SIFT
in order to eliminate the canopy pattern.
In summary, the current video frames are
processed online to compare their keypoints with the
ones at the reference image. This way the natural
landmark is tracked. As soon as the landmark is
found the vision system locks it, while preparing to
search the next landmark.
2.1 Nonrelevant Features Suppression
The wavelet transform is an useful and powerful tool
for image local analysis and processing. As digital
images are discrete data, we use the discrete wavelet
transform (DWT). This way, only really large and
robust features will be well represented.
According to (Meddeber et al, 2009), the same
image is represented in different resolutions and
scales in each decomposition level. Thus, the
nonrelevant features disappear in the low resolutions
(large scales) and the biggest and really important
features can be identified more easily.
In each decomposition level is created four
images (sub-bands): LL, LH, HL and HH. This
decomposition can be repeated recursively, reaching
other levels. Therefore, LL sub-band contains
maximum information compared to others sub-bands
(Malviya and Bhirud, 2009), and it is considered the
approximation of original image, also with the small
features suppressed.
2.2 Keypoints Identification and Point
Matching
The main process is the identification of keypoints
in the acquired image and matching them with the
reference image keypoints set. In this paper, the
keypoints can be rivers, roads, its details (corners,
turns, margins, etc) or any information that does not
correspond only to trees.
There are automatic methods to identify
keypoints, developed from algorithms that use
similarity measurements. The same method applied
in the identification of the keypoints of the reference
image (done offline) has to be applied in the sensed
video frame (during the flight). The SIFT, developed
by (Lowe, 2004) was utilized in this work to
generate descriptors of keypoints which are invariant
to scale, rotation and partially invariant to change in
illumination.
There are also other methods for automatic point
identification and matching, such as SURF (Speed
Up Robust Features) and ASIFT (Affine SIFT).
Both are extensions of the SIFT. The SURF (Bay et
al, 2006) uses integral images for image convolution
and thus computing and comparing features much
faster. And the ASIFT (Morel & Yu, 2009) uses the
same SIFT techniques to be invariant to scale and
rotation, combined with point of view simulations,
which provides much more correct matches.
Therefore, as confirmed by the experimental results
in the next section, SIFT is by far the best suited
method for feature matching to our scenario of
application.
2.3 SIFT Adaptation
As wavelet advantages mentioned before, the SIFT
algorithm was adapted in order to detect only
relevant features for this problem, in addition to
reduce your runtime. In (Kim et al, 2007), they
propose a SIFT adaptation that uses Difference of
Wavelets (DoW) for detection of local extrema.
Applied to forest images, this method extract a lot of
features including canopy pattern, it can be verified
in the next section.
Inspired in DoW method, we use the LL sub-
bands of wavelet decomposition just to be the first
image of each octave in the space-scale generated by
SIFT (Figure 2). This way, the wavelet transform is
responsible to suppress nonrelevant features each
next octave.
3 PRELIMINARY RESULTS
The software application was implemented using
C++ language with OpenCV library. The images and
videos used were obtained by flights over forest
areas near the cities of Manaus and Belo Horizonte
(Brazil).
Visual-basedNaturalLandmarkTrackingMethodtoSupportUAVNavigationoverRainForestAreas
417