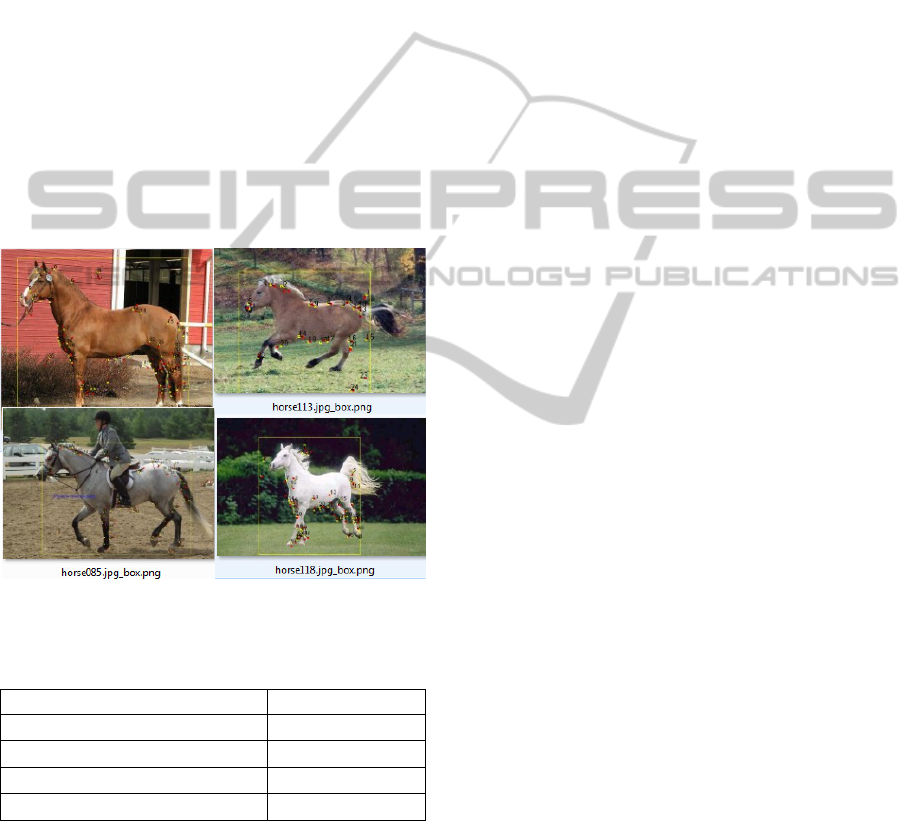
6 DISCUSSION
There are other works that used turning angles for
contour recognition e.g. (Rusinal et al., 2007;
Kpalma et al., 2008), but those works either tested
on simple images or used turning angles which are
based on technical or mathematical arguments,
whereas our work are derived from psychological
and physiological research.
This work is closest with Shotton et al. (2008)
work, and their results are so far the best, but
compared to Shotton et al., our method achieved
comparable results on the same Weizmann Horse
database. Both approaches work well, despite the
rather challenging images with background clutter;
and wide variety of poses and sizes. The images that
are misclassified are due to significant pose
differences, the small size of the target object and
similarity of the background edges to the training
model edges.
Figure 4: Results of Horse recognition with automatically
detected bounding box (yellow).
Table 1: Comparison of classification results.
Results ROC AUC
Shotton-Boosted Edge 0.9518
Shotton (retrained)-Canny 0.9400
SVM-SIFT 0.8468
Our method 0.9966
Shotton et al., (2008) use a total 228 horse images
and Caltech 101 background set for tests, whereas
we use 238 horse images (from the same Weizman
database) against 244 animals and buildings images
from the Broderbund 65,000 ClickArt collection.
The Caltech 101 background category consist of
assorted scenes around the Caltech campus is
comparable to the building images that we use. The
animal category that we use is likely to be more
challenging and not used in Shotton et al. Based on
the published results (Table 1), our method achieved
a better classification rate.
Our method do not require building a codebook
of contours, as we used turning points that made
comparison easier as we are comparing points with
points, wherease Shotton et al. (2008) used a
comparatively more complicated chamfer distance
measure that required the contour need to be aligned,
complicating the procedure.
In summary, we have presented a perceptually
justified edge boundary feature based on psychology
and neurophysiological research.
REFERENCES
F. Attneave, 1954. "Some informational aspects of visual
perception," Psychological Review, vol. 61, pp. 183-
193.
X. Bai, Q. Li, L. J. Latecki, and W. Liu, 2009. "Shape
band: A deformable object detection approach," in
IEEE Computer Society Conference on Computer
Vision and Pattern Recognition, Miami, Florida, pp.
1335-1342.
S. Belongie, J. Malik, and J. Puzhicha, 2002. Shape
Matching and Object Recognition Using Shape
Contexts," IEEE Transactions of Pattern Analysis and
Machine Intelligence, vol. 24, pp. 509-522.
J. Feldman and M. Singh, 2005. "Information Along
Contours and Object Boundaries," psychological
Review, vol. 112, pp. 263-252.
V. Ferrari, F. Jurie, and C. Schmid, 2010. "From Images to
Shape Models for Object Detection," International
Journal in Computer Vision, vol. 87.
D. D. Hoffman and M. Singh, 1997. "Salience of visual
parts.," Cognition, vol. 63, pp. 29-78.
K. Kpalma, M. Yang, and J. Ronsin, 2008. "Planar Shapes
Descriptors Based on the Turning Angle Scalogram,"
in ICIAR '08 Proceedings of the 5th international
conference on Image Analysis and Recognition, pp.
547-556.
A. Kristjansson and P. U. Tse, 2001. "Curvature
discontinuities are cues for rapid shape analysis,"
Perception & Psychophysics, vol. 3, pp. 390-403.
A. Opelt, A. Pinz, and A. Zisserman, 2006. "A Boundary-
Fragment Model for Object Detection.," in European
Conference on Computer Vision, pp. 575-588.
A. Pasupathy and C. E. Connor, 2001. "Shape
representation in area V4: Position-specific tuning for
boundary conformation," The Journal of
Neurophysiology, vol. 86, pp. 2505-2519.
M. Rusinol, P. Dosch, and J. Llados, 2007. "Boundary
Shape Recognition Using Accumulated Length and
Angle Information," Lecture Notes in Computer
Science, vol. 4478, pp. 210-217.
J. Shotton, A. Blake, and R. Cipolla, 2008. "Multi-Scale
VISAPP2013-InternationalConferenceonComputerVisionTheoryandApplications
490