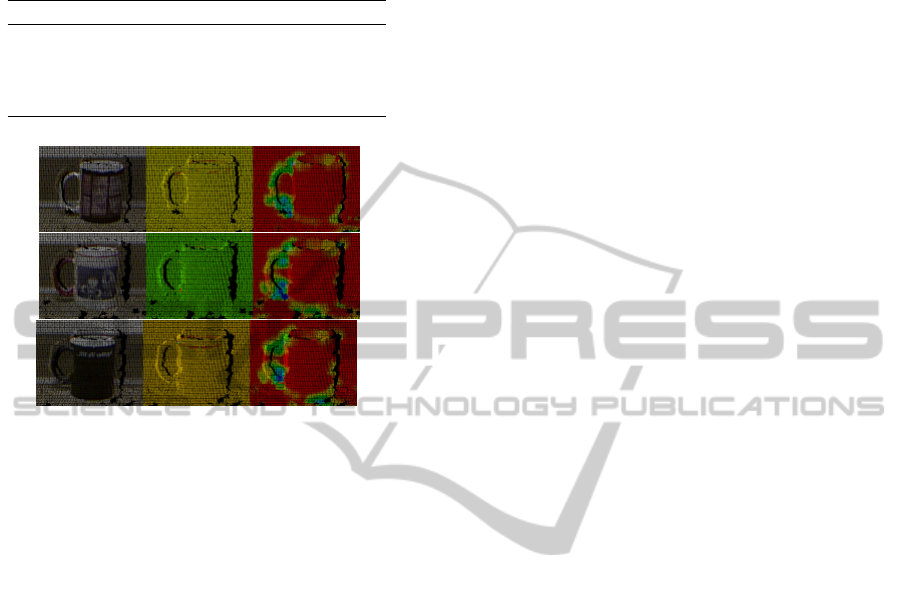
Table 1: Corners repeatability mean and standard error de-
viation in strong light variation for 2D and 3D Harris corner
detectors. P
i
1≤i≤3
are the input clouds. The mean error
¯
ε
2D
high values show how light affects 2D corners.
pairs P
1
− P
2
P
1
− P
3
P
2
− P
3
¯
ε
2D
177034 695510 698783
¯
ε
3D
0 0 0
σ
2D
1.54e + 06 5.42e + 06 5.81e + 06
σ
3D
0 0 0
Figure 4: Geometric corners detection: texture invariance.
Cornerness is displayed from green (weak) to red (strong).
3D cornerness - right column - is almost the same for all the
objects although they carry different textures while 2D cor-
nerness - middle column - varies according to the texture.
and normals. Detection results (non filtered) are pre-
sented in Figure 4.
The results shown on Figure 4, confirm our intu-
ition: since we rely solely on geometric data varia-
tion for this experiment, objects of the same shape
should have similar signature. Lecturers can easily
notice that 3D cornerness measure is analogous for
the whole 3
rd
column while it differs depending on
the object texture along the 2
nd
column.
5 CONCLUSIONS
This paper addresses the problem of corner detection
in RGB-D space to improve repeatability under strong
light variation or in texture-less environments. The
novelty of the proposed solution is the use of a geo-
metric criterion to assess the nature of a point. The
novel detectors are extension of popular 2D images
corner detectors: second moment matrix and self dis-
criminality ones.We prove stability of designed de-
tectors through experimental validation. Future work
include application to point cloud registration, object
recognition and tracking.
ACKNOWLEDGMENTS
This work has been supported both by the French na-
tional research agency (ANR) by the project ANR As-
sist ANR-07-ROBO-0011, and by the Willow Garage
company, Menlo Park, California, USA.
REFERENCES
Bay, H., Tuytelaars, T., and Van Gool, L. (2006). Surf:
Speeded up robust features. pages 404–417.
Harris, C. and Stephens, M. (1988). A combined corner and
edge detection. In Proceedings of The Fourth Alvey
Vision Conference, pages 147–151.
Knopp, J., Prasad, M., Willems, G., Timofte, R., and
Van Gool, L. (2010). Hough transform and 3d surf for
robust three dimensional classification. In Proceed-
ings of the 11th European conference on Computer vi-
sion: Part VI, ECCV’10, pages 589–602, Berlin, Hei-
delberg. Springer-Verlag.
Lai, K., Bo, L., Ren, X., and Fox, D. (2011). A large-
scale hierarchical multi-view RGB-D object dataset.
In ICRA, pages 1817–1824. IEEE.
Li, J. and Allinson, N. M. (2008). A comprehensive review
of current local features for computer vision. Neuro-
comput., 71(10-12):1771–1787.
Moravec, H. P. (1981). 3d graphics and the wave theory. In
SIGGRAPH ’81: Proceedings of the 8th annual con-
ference on Computer graphics and interactive tech-
niques, pages 289–296, New York, NY, USA. ACM.
Redondo-Cabrera, C., Lopez-Sastre, R. J., Acevedo-
Rodriguez, J., and Maldonado-Bascon, S. (2012).
SURFing the point clouds: Selective 3D spatial pyra-
mids for category-level object recognition. In IEEE
CVPR.
Sipiran, I. and Bustos, B. (2011). Harris 3d: a robust exten-
sion of the harris operator for interest point detection
on 3d meshes. Vis. Comput., 27(11):963–976.
Smith, S. M. and Brady, J. M. (1995). Susan - a new ap-
proach to low level image processing. International
Journal of Computer Vision, 23:45–78.
Trajkovic, M. and Hedley, M. (1998). Fast corner detection.
Image Vision Comput., 16(2):75–87.
Tuytelaars, T. and Mikolajczyk, K. (2008). Local invariant
feature detectors: a survey. Found. Trends. Comput.
Graph. Vis., 3(3):177–280.
KeypointsDetectioninRGB-DSpace-AHybridApproach
499