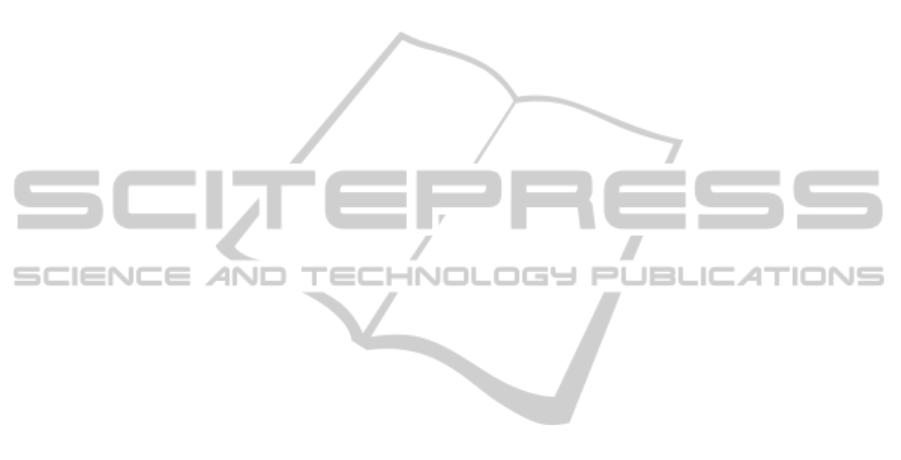
exchanged context information. Standardized login
credentials are also needed. Initial contributions pro-
pose an ”identity aggregator” to store these creden-
tials and transparently map the services to the proper
credentials (Beach et al., 2010). However, the ”iden-
tity aggregator” idea faces its own set of challenges
such as security threats, ad hoc communication, cen-
tralized versus distributed processing. Furthermore,
research studies are needed to determine the best dis-
tribution of the expected collection of data for satis-
fying optimal processing times and storage and I/O
cost.
For power conservation, some research contribu-
tions propose solutions such as balancing process-
ing and communication among the resource-rich and
resource-weak devices (Schuhmann et al., 2010). The
frequencyof context information update could also be
set based on its frequent change and urgency (Beach
et al., 2010), and trends to use renewable power
sources such as solar power and wind in charging the
SPSs (Cook and Das, 2012) could be used. More in-
novative energy harvesting techniques can be adopted
such as transforming the electromagnetic waves of
the surrounding objects or the negative human en-
ergy (NHE) into an electric form suitable for charging
wearable/mobile devices. On another front, inclusion
of NHE-detection sensors in mobile devices, coupled
with the user status extracted from OSN, can guide
both systems to adapt their themes/applications to the
currently detected negative user mood.
Distributed processing and Cloud computing are
among the promising venues that support SPS scal-
ability and compensate for resource deficiency in
resource-weak devices. Cloud computing also shows
potential in resolving the stress of massive real-time
processing. Besides, we believe that predicting con-
text offline can save time and reduce the stress of the
huge real-time processing.
Finally, for privacy preservation, users may set
context-aware privacy settings that change per loca-
tion, time, or user mood. In addition, secure zones
through which SPS can safely migrate their context
data could be researched.
REFERENCES
Baldauf, M. et al. (2007). A survey on context-aware sys-
tems. Int. J. Ad Hoc and Ubiquitous Computing,
2(4):263–277.
Beach, A. et al. (2010). Fusing mobile, sensor, and social
data to fully enable context-aware computing. In Hot-
Mobile, pages 60–65.
Bellavista, P. and Helal, S. (2008). Location-Based Ser-
vices: Back to the Future. Per. Comp., 7(2):85–89.
Boyd, D. and Ellison, N. (2008). Social Network Sites:
Definition, History, and Scholarship. J. of Computer-
Mediated Communication, 13(1):210–230.
Cook, D. and Das, S. (2012). Pervasive computing at scale:
Transforming the state of the art. Pervasive and Mo-
bile Computing, 8(1):22–35.
den Broeck, W. et al. (2010). The Live Social Semantics ap-
plication: a platform for integrating face-to-face pres-
ence with on-line social networking. In PERCOM
Workshops, pages 226–231.
Eagle, N. (2010). Big data, global development, and com-
plex social systems. In ACM SIGSOFT, pages 3–4.
Eagle, N. and Pentland, A. (2005). Social Serendipity: Mo-
bilizing Social Software. Per. Comp., 4:28–34.
Eagle, N. and Sandy, A. A. (2006). Reality mining : sens-
ing complex social systems. Personal and Ubiquitous
Computing, 10(4):255–268.
Garcia-Crespo, A. et al. (2009). SPETA: Social perva-
sive e-Tourism advisor. Telematics and Informatics,
26(3):306–315.
Gay, G. (2009). Context-Aware Mobile Computing: Affor-
dances of Space, Social Awareness, and Social Influ-
ence. Morgan & Claypool.
Kompatsiaris, Y. et al. (2010). Information Extraction from
Social Sites. In SSMS.
Kwon, O. (2009). A social network approach to resolving
group-level conflict in context-aware services. Expert
Systems with Applications, 36(5):8967–8974.
Lehsten, P. et al. (2010). A Service-oriented Approach to-
wards Context-aware Mobile Learning Management
Systems. In PERCOM, pages 268–273.
Lovett, T. et al. (2010). The Calendar as a Sensor: Anal-
ysis and Improvement Using Data Fusion with Social
Networks and Location. In UbiComp, pages 3–12.
Mahmud, J. et al. (2010). AVARA: A system to improve
user experience in web and virtual world. In IUI Pro-
ceedings, pages 349–352.
Mendes, J. (2008). SOCIALNETS: Social networking for
pervasive adaptation. Technical Report 217141, EC.
Mokhtar, S. B. et al. (2010). A self-organising directory and
matching service for opportunistic social networking.
In SNS Workshop, pages 1–6.
Quercia, D. et al. (2010). Using Mobile Phones to Nurture
Social Networks. Per. Comp., 9(3):12–20.
Sambasivan, N. et al. (2009). UbiComp4D: Infrastruc-
ture and Interaction for International Development-the
Case of Urban Indian Slums. In ACM UbiComp.
Santos, A. et al. (2009). Context Inference for Mobile Ap-
plications in the UPCASE Project. In MOBILe Wire-
less MiddleWARE - MOBILWARE, pages 352–365.
Sapuppo, A. and Sø rensen, L. (2011). Local Social Net-
works. In ICTTA, volume 5, pages 15–22.
Satyanarayanan, M. (2001). Pervasive computing: vision
and challenges. Personal Communications, IEEE,
8(4):10–17.
Schuhmann, S. et al. (2010). Efficient Resource-Aware Hy-
brid Configuration of Distributed Pervasive Applica-
tions. In PERCOM, pages 373–390.
Tian, Y. et al. (2010). Social Multimedia Computing. IEEE
Computer Society, 43(8):27–36.
Vassena, L. (2009). Context-aware retrieval going social. In
FDIA Proceedings, pages 62–68.
PECCS2013-InternationalConferenceonPervasiveandEmbeddedComputingandCommunicationSystems
124