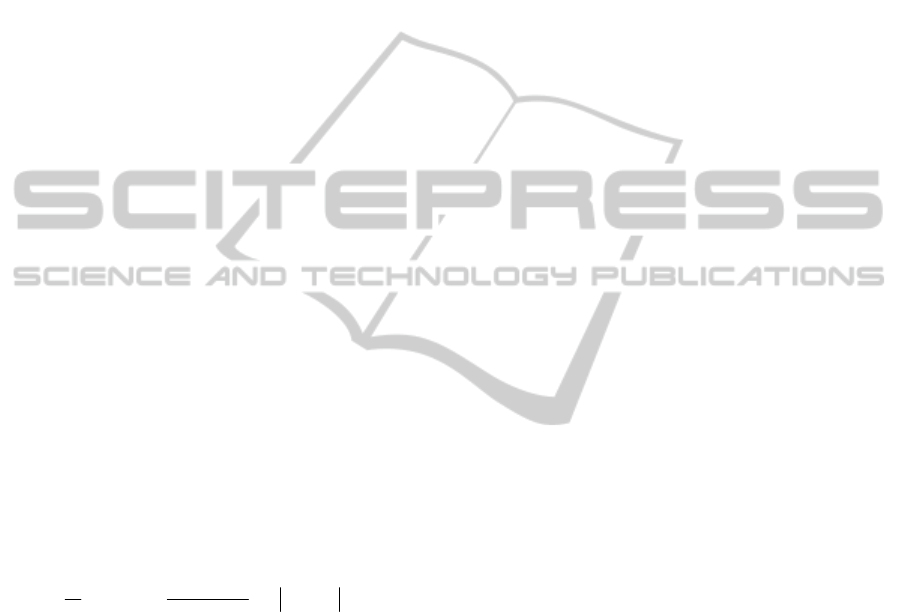
(1983) for the facial and maxilar elevator muscles,
Weytjens and Van Steenberghe (1984) for the biceps
brachii, Englehart and Parker (1994) for the
abductor pollicis. In other cases however, the peaks
of different MUAP trains are smoothed or
completely eliminated by cancellation (De Luca,
1979, Weytjens and Van Steenberghe, 1984). For
this peak to be clearly observed, either the EMG
signal is composed by regular MUAP trains with
similar firing rate, or it is dominated by a MUAP of
large amplitude (De Luca, 1979). With healthy
muscles, the first cause is more likely to be present
than the second one (Basmajian and De Luca 1985).
In neuropathic conditions however, reinervation
processes may create MUs composed of an unusual
high number of muscle fibres and the second
condition may then be present.
Various mathematical models for the EMG
signal have been proposed such as the ones of Lago
and Jones (1977) and De Luca, (1979) where a
MUAP train is modelled by:
k
n
k
tthtu
1
(1)
where h(t) is the temporal MUAP waveform and t
k
are the time instants where the actual MUAPs occur.
Differences between two successive MU firing
instants (t
k
-t
k-1
) are called interpulse intervals (IPIs)
and are modelled as independent random variables
and thus constitute a renewal process. Under
conditions of stationarity, i.e., non-varying h(t) and
non-varying IPI probability density function (PDF),
the power spectrum of a signal corresponding to a
MUAP train is given by:
2
1
Re21
1
jH
jF
jF
S
(2)
where
is the mean IPI, F(j
) is the Fourier
transform of the IPIs PDF and H(j
) is the Fourier
transform of h(t). Various distributions such as
Gaussian, gamma, Poisson and Weibull distributions
have been proposed to accommodate experimental
data (Merletti and Parker, 2004). All of them lead to
one principal peak in the signal power spectrum with
additional smaller ones at subsequent harmonics. All
those peaks are blurred as the coefficient of variation
of the IPI (CVI) increases, particularly as it
approaches values of 0.3 (Weytjens and Van
Steenberghe 1984).
The features of the EMG power spectrum in
relation to the IPI statistical characterization and the
degree of stationarity have been amply studied
through analytical derivation and simulation (Lago
and Jones, 1977), (De Luca, 1979), (Englehart and
Parker, 1994). Other studies have been focussed on
the statistical relationship between EMG variables,
such as the root mean square amplitude or the mean
power frequency, and MU firing rates (Christie et al.
2009), (Fuglesang-Frederiksen and Ronager, 1988).
However, the influence on the EMG power spectrum
of the number of MUAP trains, the mean firing rates
of these trains, the CVI and the signal to noise ratio
(SNR) has not undergone similar systematic studies.
The aim of this work is to present a method for
estimating the frequency range of the firing rates of
the set of MUAP trains that compose an EMG signal
based on the Fourier power spectrum. The capacity
of the approach for varying number of MUAP trains,
actual firing rate range (FRR), ICV and noise level
was explored through extensive simulation runs
using the afore mentioned EMG generation model.
2 MATERIAL
10 s-long simulated EMG signals were obtained as
the sum of several MUAP trains, each of which
generated as the multiple repetition of a given
MUAP waveform. Intervals between MUAP
occurrences followed Gaussian distributions whose
mean was the inverse of the firing rate. The firing
rate and the coefficient of variation for each of these
trains were set as input parameters in the different
analysis tests. MUAP waveforms were taken ‘off-the
self’ from a set of potentials recorded from the
deltoid muscles of different patients in a previous
study (Rodríguez et al., 2010). White Gaussian noise
was added to the signals so that specific levels of
SNR could be tested. The sampling rate of the
simulated signal was set to 20 kHz.
Different tests were performed to evaluate the
performance of the method. In the tests some of the
input parameters were given a fixed value while
some were varied in a systematic way or randomly
within a certain range. Simulations were run 500
independent times for every tested parameter value.
- The first test concerned the detection
performance for different FRR values. 10
MUAP trains composed the simulated signals,
whose mean firing rates were independent
randomly taken in the range [f1-f2], which we
will call nominal frequency range (NFR)
hereafter. f1 was set to 10 Hz and f2 was varied
from 11 to 20 Hz in 1 Hz steps. An SNR of 20
dB, a random variation in the amplitude of the
BIOSIGNALS2013-InternationalConferenceonBio-inspiredSystemsandSignalProcessing
378