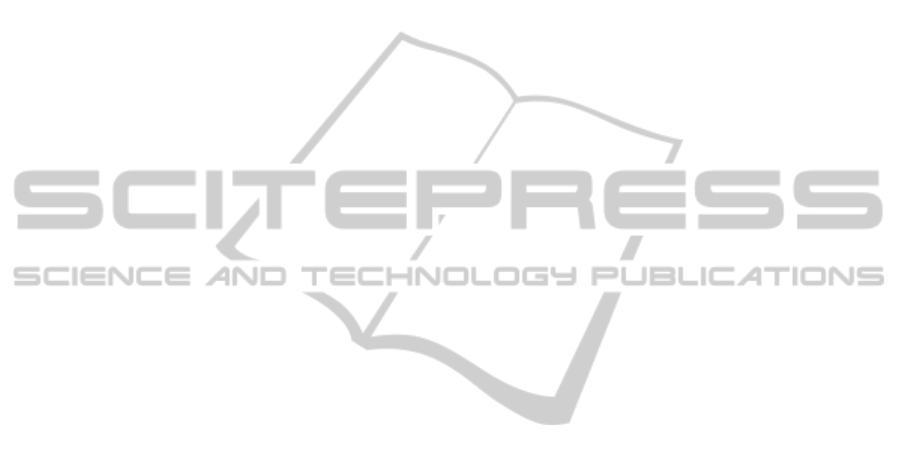
issues shall be addressed in relation to that
operation. These are: the method of dividing feature
space and the formulation of the classification
problem.
There are basically utilized the distance principle
and the decision boundary concepts while
developing classifiers (Snopok and Kruglenko,
2002). In the first approach the class assignment is
performed by calculating the data point distance to
different classes and by choosing the least distant
class. The second strategy involves the division of
the feature space into subspaces by imposing
decision boundaries which are in general
multidimensional surfaces (in 2D space these are
lines). They divide the space into disjoint regions
associated with distinct classes. The advantage of
the second approach consists in proposing the
division of entire feature space directly. Although
far from the regions populated by the calibration
data points the division shall be treated with caution
the feature space is reasonably well structured close
to them. Moreover, the class assignment of the new
data is related to its location with respect to the
displayed decision boundaries. In the particular
application considered in this work, the methods
based on decision boundaries would be favored also
for another reason. We found that the set of points
representing the gas mixture evolution has a
characteristic layout in the feature space, see Fig. 2a.
They are not arranged into a number of disjoint
clusters but they rather form one stretched grouping.
Due to the continuous transition between the stages
of gas mixture evolution the data points representing
subsequent stages are neighbours. In such
circumstances, the methods designed to cope well
with compact and disjoint clusters are inappropriate.
In this work, Discriminant Analysis was applied for
feature space partitioning. It is the most simple and
least time consuming classification method based on
decision boundary concept. We tested whether the
liner technique (LDA) or Quadratic Discriminant
Analysis (QDA) is more suitable for our application.
The way of formulating the classification
problem gains importance while applying multi-
criteria division of the feature space. It is a necessity
upon monitoring the evolution of gas mixture.
Willing to discriminate k stages of gas mixture
emission decay one may decide to apply one
division of feature space into k classes or k-1 two-
part divisions. While the first approach is quite
inflexible, the other leaves space for some
adjustment of the feature space structure to the
relative importance of the individual criteria. In this
work there were applied the criteria allowing to
assess VOCs emission process regarding their odor
impact (CIWEM's, 2011). We were willing to
discriminate between the following three stages of
gas mixture evolution: odor intensity below 1
OU
E
/m
3
, odor intensity between 1 OU
E
/m
3
and 3
OU
E
/m
3
and odor intensity higher than 3 OU
E
/m
3
. In
principle, the examination was performed for each
component of gas mixture separately.
Finally, one shall articulate the criteria of
selecting the best feature space. In this work we
considered two criteria. The first criterion was the
misclassification rate of reference gas mixtures. This
objective criterion indicated how successful was the
particular feature space together with the way of its
partitioning in assigning training patterns,
representing different stages of VOCs emission
process to the appropriate classes. There was also
applied other, subjective criterion. It referred to the
correspondence between the logics of feature space
fragmentation and the logics of gas mixture
evolution. Namely, there were examined the
arrangement, in particular the neighbourhood, of
distinct fragments of feature space, representing the
sequence of VOCs emission decay stages.
4 RESULTS AND DISCUSSION
The accuracy of reference gas mixtures
classification was the first criterion employed for the
assessment of data visualization method dedicated to
semi-quantitative gas assessment using sensor array.
The criterion was aimed to represent the accuracy of
feature space division with respect to the stages of
VOCs emission process. The following aspects of
visualization were examined with this criterion: 1)
selection of feature space coordinates (single sensor
related or sensor array related), 2) method of feature
space partitioning (linear or nonlinear), 3)
formulation of feature space division problem (k-1
divisions into two-part, or one division into k parts).
The misclassification rates of reference gas
mixture patterns are presented in Table 2, Table 3
and Table 4. The values shown in the tables are
medians. They provide a statistical indication of
misclassification rates obtained in feature spaces,
which were built separately for each time point of
sensor signal obtained upon dynamic exposure. Due
to the fact that misclassification rates were low for
most of the time points, the error rates shown in
Table 2, Table 3 and Table 4 well represent
minimum misclassification rates achievable with the
particular approach. The pairs of best sensor are
quoted in Table 5.
SENSORNETS2013-2ndInternationalConferenceonSensorNetworks
166