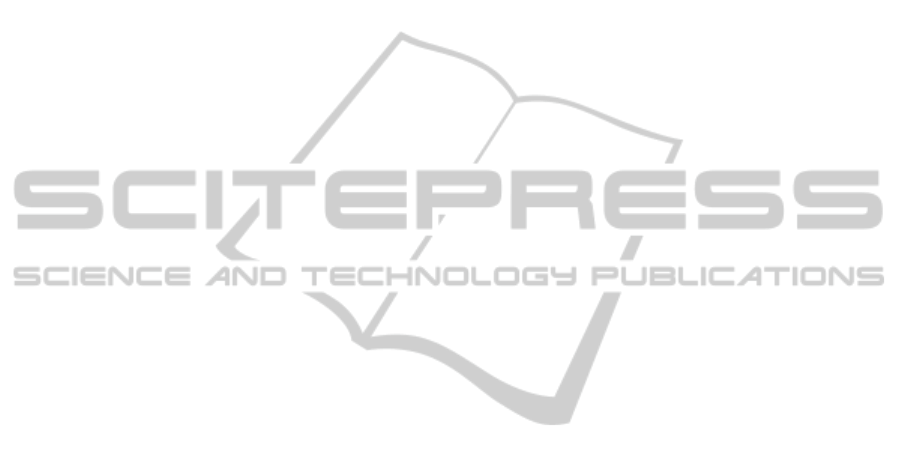
power. Traders may, also, unleash several agents
with multiple identities for false name bidding.
Hence, this work establishes, denying e-traders (e-
buyers and e-sellers) expressing strategic conduct,
allegedly, to improve computation efficiency as in
Google DoubleClick, Microsoft AdECN, Yahoo
Right Media, and Facebook FBX, is unsubstantiated
of dire business impact, given modern enabling hi-
tech is transforming computation into commodity.
Indeed, the flexible expressions of fair strategic
conduct that bring self-prosperity would, ultimately,
mitigate adverse strategies that may defy markets,
considering, often, higher risks and lower expected
returns (Fair vs. adverse strategies to be examined in
a coming work), given the dynamics of smart
learning thru social interactions and repetitive trades.
In fact, Adam Smith envisions traders interacting in
free markets act as if guided by “invisible hand” that
leads to desirable outcomes (i.e. efficiency and
stability) due to markets’ inherent flexibility of
natural free choice and smart interaction. In fact,
free market dynamics promote realizing impact of
continuous learning on strategic conduct. However,
free market efficiency would, often be realized with
market thickness, uncongested interaction, and safe
privacy (Roth, 2007). This work extends it, also, to
applying fair rules of game-theoretic encounter (i.e.
no enforced monopolistic rules). However, while
online services gold rush and thriving technologies
have tilted trader tactics to conceding to e-market
restrictions, apparently, at the expense of strategic
benefits, for the direct gains of easy access to the
wealth of inventories and information liquidity,
sustainability would be exposed, at which priorities
align with the natural expression of strategic conduct
higher returns, and better quality of service.
In the second issue, the work establishes the lack
of rapid consumer-to-marketplace automation during
bidding lifecycles is another compelling challenge to
expressing strategic conduct. In fact, the time wasted
in bidding processes at e-marketplaces like eBay,
Amazon, etc. is an irritating engagement experience.
For instance, a bidding lifecycle may take days, for
an e-Bay auction, with rather manual configurations.
Hence, the work introduces the concept of “bidding
lifecycle”, examine it effectiveness in divers trades
and establishes, the flexible expressions of strategic
rules (i.e. sub-programs) during the bidding process
that are collected, stored and exploited by the smart
exchange (SX), to deliver rapid bidding lifecycles.
The third challenge relates to the mounting
combinatorial complexity of online ad problem
evident in the emerging real-time bidding (RTB) of
single users’ attentions. RTB allows advertisers bid
for single impressions, using user profiles, cost
thresholds, and campaign goals to optimally assign
bid values at real-time. RTB provides more liquidity,
visibility, and competitive bidding, essential for the
sustainable growth. In fact, contemporary e-markets
are exploiting the complex multichannel engagement
user experiences of online services that facilitate
better market openness, and transparency. However,
the combinatorial complexity (i.e. cherry-picking) of
user level trades lack of efficient control, massive
growth, and fierce competition are main concerns
Finally, a common issue in the decentralized e-
markets relates to the implemented computation
mechanism design for SX-CAP. The game-theoretic
economics and computation complexities of the SX-
CAP are observed in the GSP auction (Varian, 2007)
(Edelman, Ostrovsky, & Schwartz, 2007), while it is
allocative efficient (AE), it is not incentive
compatible (IC) and often, maximizes auctioneer’s
revenue, rather than traders’. Conversely, while
VCG auction is efficient and stable it is, often,
intractable and runs at deficit. The iterative models
(Ausubel & Milgrom, 2006) (Parkes, 2006), take
longer time to converge with no guarantees of either
AE or stability, an issue tackled, for instance, by
iterative VCG (Parkes, 2001).The work, hence,
targets a SX model that delivers an efficient, stable
and tractable e-trading allocation for self-interested
rational traders with independent private information
and strategic conduct of rather conflicting goals.
This work examines and reflected on overlooked
issues and, ultimately, develops a novel “rule-based”
bidding language (RBBL) for smart exchange (SX)
that allows for flexible expressions of smart strategic
rules formulae. The RBBL is fully symmetric that
enables flexible and rapid e-trading while unlocking
the natural expressions of strategic conduct, not only
for e-buyers, but, also, for e-sellers, often, confined
with the reserved values. The RBBL empowers the
SX to deliberate smart rules for rapid preference
elicitations and valuations that ultimately, delivers
rapid bidding lifecycle. The inherent game-theoretic
economics and computation complexities of SX and
the emerging combinatorial complexity of e-trading
of user attentions, inspire designing the GSP based
double auction (DA) matching (GSPM) that
uniquely blends forward and reverse GSP auctions
to achieving self-prosperity (i.e. max utility), social
efficiency, strategic stability and computational
tractability. The GSPM exploits the recent business
successes and endorsements of the efficient, yet
simple GSP auction (Edelman, Ostrovsky, &
Schwartz, 2007) (Varian, 2007) and the theoretical
Nash stability of GSP repeated best response auction
(Nisan, Schapira, Valiant, & Aviv, 2011). The
RBBL and GSPM, ultimately, empower bidders and
SX with flexible expressions of smart rules and
interaction pattern, smart preferences elicitation and
efficient winner determination. The SX would,
eventually, provide a timely seamless access to the
TowardsaSustainableSmarte-Marketplace-AStable,EfficientandResponsiveSmartExchangewithStrategicConduct
339