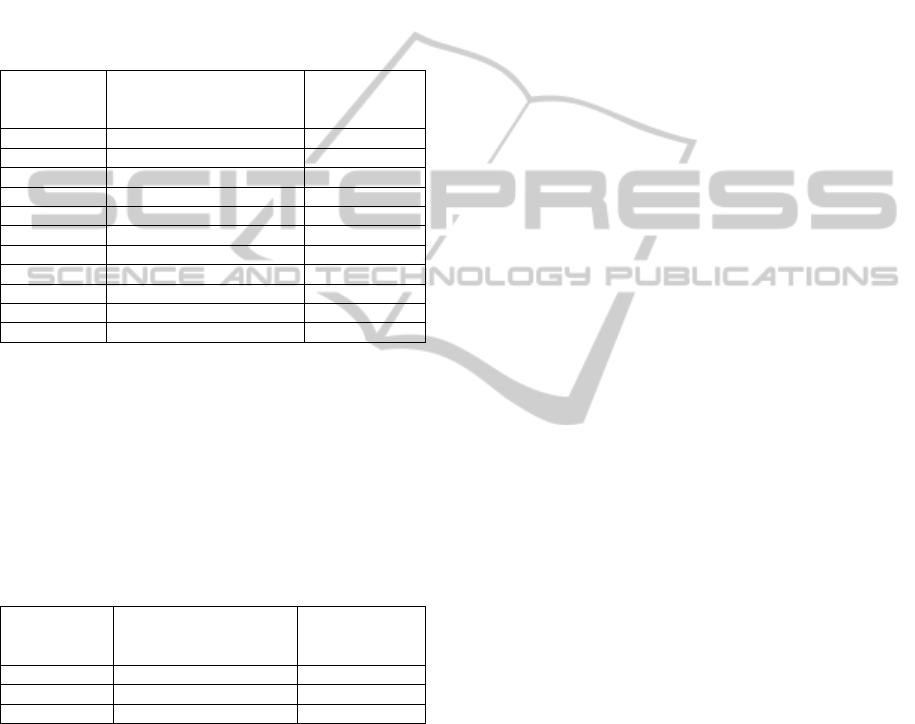
Table 1: Data set A. Illustration of the most relevant
characteristics of the dynamic data set used for the first
experimental setting. This data set is composed of NMR
data collected using a total echo time (TE) equal to 23ms
and a repetition time (TR) of 1070ms from 11 healthy
patients. Each data matrix A
i
(i =1,2,..,11) is composed
approximately of 300 rows and 1068 columns. In other
words, the samples belong to an input space of 1068
dimensions. The notation used for the voxel location in
NMR Spectroscopy is “Left-Right” (L/R) for the “x”
dimension, “Anterior-Posterior” (A/P) for the “y”
dimension and “Inferior-Superior” (I/S) for the “z”
dimension. Voxel dimensions are expressed in
millimetres.
Data Set A
TE = 23ms
TR= 1070ms
Voxel Location Voxel Size
[A
1
] [L,P,I]=[0.9,6.3,17.2] [20,20,20]
[A
2
] [L,P,S]=[8.1,27.7,51.5] [29,20,27]
[A
3
] [L,P,S]=[6.7,9.5,15.1] [20,20,20]
[A
4
] [L,P,S]=[0.3,6.6,44.9] [20,20,20]
[A
5
] [L,P,S]=[1.2,18.5,61.6] [20,20,20]
[A
6
] [R,P,S]=[0.3,14.6,68.8] [20,20,20]
[A
7
] [L,P,S]=[2.79,25.97,60.9] [20,18,15]
[A
8
] [R,P,S]=[21.3,92.8,43.2] [20,20,20]
[A
9
] [L,P,S]=[27.4,25,63.8] [20,20,20]
[A
10
] [L,P,S]=[23.2,26.1,38.7] [20,20,20]
[A
11
] [R,P,S]=[4.1,13.3,45.1] [29,20,27]
Table 2: Data set B. Illustration of the most relevant
characteristics of the dynamic data set used for the second
experimental setting. This data set is composed of NMR
data collected from 3 patients using a total echo time (TE)
equal to 35ms and a repetition time (TR) of 1500ms. Each
data matrix B
i
(i =1,2,3) is composed approximately of
200 rows and 1068 columns. The data matrix B
2
corresponds to a patient who was diagnosed with a
tumour. The rest of data correspond to healthy patients.
The notation used for voxel location and the units used for
the voxel size are identical to those used in table 1.
Data Set B
TE = 35ms
TR= 1500ms
Voxel Location Voxel Size
[B
1
] [L,A,S]=[19.4,14.8,96.3] [16.5,17.7,17]
[B
2
] [R,A,S]=[20.9,35.5,76.2] [20,29.6,20]
[B
3
] [L,A,S]=[30,16,64.7] [20,20,20]
All the spectral data were generated and pre-
processed using a spectroscopic and processing
software package from GE Medical Systems
(SAGE). This tool comes with a set of built-in
functions (macro reconstruction operations) which
provide different useful processing options of raw
FID data. We used a macro reconstruction operation
which provides internal water referencing, spectral
apodization, zero filling, convolution filtering and
Fourier transform operation on each of the acquired
frames. However, it is important to note that the
convolution filtering and water suppression options
were not selected. The result of this processing step
is a data matrix where each column represents a
temporal series spectrum of a specific metabolic
signal and each row represents a sample or pattern
from the brain region of interest. Each sample
belongs to a space of 1068 dimensions
corresponding to a chemical shift range of [-0.8, 4.3]
ppm. As mentioned in section 2, this interval
corresponds to the range where the main resonances
concerning the 35 known metabolites involved in
brain metabolism are located.
4.2 Experimental Results
The first experiment conducted was designed to
check the variance of the measures corresponding to
different healthy subjects. At this point, it is
important to remember that both data sets described
in the previous section are dynamic. In addition, the
set of parametric measures introduced in section 3.2
were proposed in a supervised learning context. In
this kind of machine learning paradigm knowledge
about the problem is represented by means of input-
output examples, specifically, examples in the form
of vector of attribute values and known classes. This
means that the samples of the data set must be rated
as belonging to a predefined set of categories. In our
case, the categories are defined according to the
number of different subjects that compose the
database. For data set A there are samples coming
from eleven different individuals, therefore
according to the proposed schema we have eleven
different categories for the samples. Moreover,
samples belonging to subject A
i
are rated as
belonging to the class C
i
where i = 1,2,…11. At this
point, it is important to highlight the fact that we
have chosen this categorization scheme for two
reasons: firstly, as stated above, in order to check the
performance of the proposed structural parameters
and secondly, because of a lack of data from patients
presenting disorders that could bias the results.
Ideally, for diagnosis purposes we would have used
just two categories for discriminating disease.
Table 3 (see the appendix for details), shows the
results obtained after computing the dispersion for
data set A after the categorization procedure
described above. The first thing to note is that most
of the dispersion values are below one, thereby
indicating a high degree of overlapping between
classes. These results would suggest that the absence
of substantial differences from data collected from
different patients and brain regions is a plausible
indication of the existence of similar metabolic
processes. It is important to emphasize that
StructuralAnalysisofNuclearMagneticResonanceSpectroscopyData
217