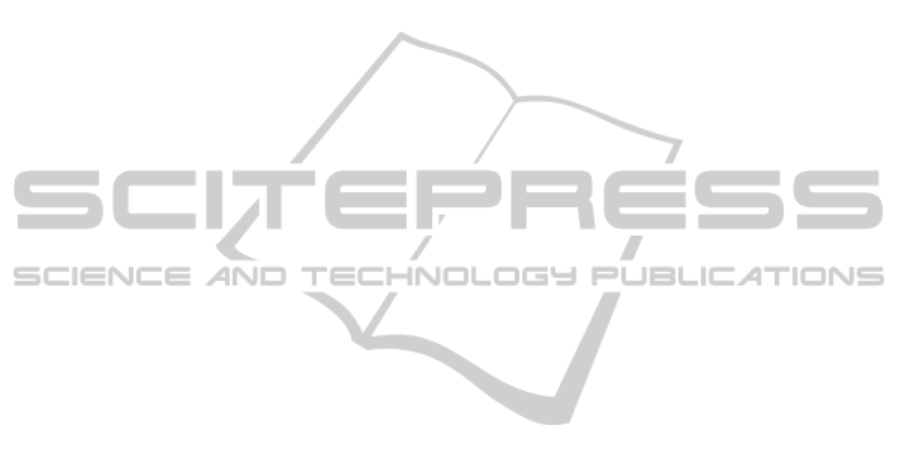
already reported, it provides higher channel density
with more signal resolution and state-of-the-art
sampling rate, which establishes an interesting
tradeoff between power consumption and system
flexibility.
The development of monitoring platforms such
as the one proposed challenges the traditional usage
of microcontrollers to interface with the ADCs and
implement low level hardware operations. Currently,
the powerful ARM processors running embedded
operating systems can be programmed with real-
time constrains at the kernel level to control
hardware, while maintaining their parallel
processing abilities in high level software
applications.
ACKNOWLEDGEMENTS
This work was supported by FCT with the reference
project FCOMP 01 0124-FEDER-010909
(FCT/PTDC/SAU-BEB/100392/2008), FCOMP 01
0124 FEDER 021145 (FCT/PTDC/SAU-
ENB/118383/2010) and by FEDER funds through
the Programa Operacional Fatores de
Competitividade – COMPETE and National Funds
through FCT – Fundação para a Ciência e
Tecnologia with the reference Project: FCOMP-01-
0124-FEDER-022674.
This work is also supported by ADI Project "DoIT -
Desenvolvimento e Operacionalização da
Investigação de Translação" (project nº 13853,
PPS4-MyHealth), funded by Fundo Europeu de
Desenvolvimento Regional (FEDER) through the
Programa Operacional Factores de Competitividade
(POFC).
REFERENCES
Campbell, A., T. Choudhury, et al. (2010). NeuroPhone:
brain-mobile phone interface using a wireless EEG
headset, ACM.
Castellaro, C., G. Favaro, et al. (2011). Hardware for
seizure prediction: Towards wearable devices to
support epileptic people, IEEE.
Chin-Teng, L., H. Hung-Yi, et al. (2006). Development of
portable wireless brain computer interface with
embedded systems. Biomedical Circuits and Systems
Conference, 2006. BioCAS 2006. IEEE.
Christoforou, C., N. Mavridis, et al. (2010). Android tele-
operation through Brain-Computer Interfacing: A real-
world demo with non-expert users.
Hsieh, H. Y., S. F. Liang, et al. (2006). Development of a
real-time wireless embedded brain signal
acquisition/processing system and its application on
driver's drowsiness estimation, IEEE.
Hung-Yi, H., L. Sheng-Fu, et al. (2006). Development of a
Real-Time Wireless Embedded Brain Signal
Acquisition/Processing System and its Application on
Driver's Drowsiness Estimation. Systems, Man and
Cybernetics, 2006. SMC '06. IEEE International
Conference on.
Lin, C. T., Y. C. Chen, et al. (2008). "Development of
wireless brain computer interface with embedded
multitask scheduling and its application on real-time
driver's drowsiness detection and warning."
Biomedical Engineering, IEEE Transactions on 55(5):
1582-1591.
Lin, C. T., F. C. Lin, et al. (2010). "EEG-based Brain-
computer Interface for Smart Living Environmental
Auto-adjustment." Journal of Medical and Biological
Engineering 30(4): 237-245.
Majoe, D., L. Widmer, et al. (2012). A Reconfigurable
Multi-core Computing Platform for Robotics and e-
Health Applications. Computer and Information
Science (ICIS), 2012 IEEE/ACIS 11th International
Conference on, IEEE.
Matthews, R., N. McDonald, et al. (2007). "Novel hybrid
bioelectrodes for ambulatory zero-prep EEG
measurements using multi-channel wireless EEG
system." Foundations of Augmented Cognition: 137-
146.
Modarreszadeh, M. and R. N. Schmidt (1997). Wireless,
32-channel, EEG and epilepsy monitoring system.
Engineering in Medicine and Biology Society, 1997.
Proceedings of the 19th Annual International
Conference of the IEEE.
Niedermeyer, E. and F. L. da Silva (2004).
Electroencephalography: basic principles, clinical
applications, and related fields, Lippincott Williams &
Wilkins.
Park, J., L. Xu, et al. (2011). Wireless dry EEG for
drowsiness detection. Engineering in Medicine and
Biology Society, EMBC, 2011 Annual International
Conference of the IEEE, IEEE.
Parthasarathy, J., J. Hogenson, et al. (2006). Battery-
operated high-bandwidth multi-channel wireless
neural recording system using 802.11 b, IEEE.
Penhaker, M., M. Stankus, et al. (2010). Design and
Application of Mobile Embedded Systems for Home
Care Applications, IEEE.
Pichiorri, F. and et al. (2011). "Sensorimotor rhythm-
based brain–computer interface training: the impact on
motor cortical responsiveness." Journal of Neural
Engineering 8(2): 025020.
Sellers, E., P. Turner, et al. (2009). "A novel dry electrode
for brain-computer interface." Human-Computer
Interaction. Novel Interaction Methods and
Techniques: 623-631.
Shao-Yen, T., T. Chung-Han, et al. (2009). A wireless
biomedical sensor network using IEEE802.15.4. Life
Science Systems and Applications Workshop, 2009.
LiSSA 2009. IEEE/NIH.
Sullivan, T. J., S. R. Deiss, et al. (2007). A low-noise,
AWirelessEEGAcquisitionPlatformbasedonEmbeddedSystems
195