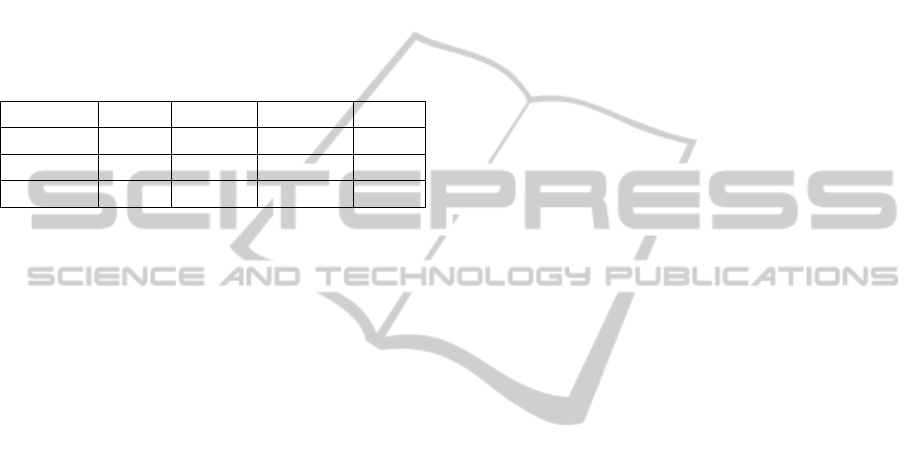
To asses the differences between both
approaches with varying time windows, the
experiment was replicated 6 times calculating error
indicators in all cases. With each new repetition, the
latest month was removed and a new split between
train and test sets was introduced using the latest
remaining month as test set. In all cases, models
were re-calculated with identical independent
variables and input parameters for both SPSS and
Weka. MAE, RMSE and MAPE were calculated in
all cases for the test set for both approaches. The
SVM approach produced better results in all cases.
Table 3: Model comparison.
Mean St.Dev. St.Err. p
∆ MAE
9.829 8.389
3.425
0.0349
∆ RMSE
10.759 9.055
3.697
0.0339
∆ MAPE
2.882 2.667
1.089
0.0456
A paired T-test was performed in order to
compare the differences between the ARIMA and
SVM approaches. The differences between all
comparisons were found to be statistically
significant in all cases with a 95% CI.
3 RESULTS
An evaluation of SARIMA and regression-based
SVM prediction models for ED arrivals has been
performed.
The SARIMA approach produced low error fits
on the train set, however the errors on the test set
were higher than with the SVM approach. In order
to generalize this approach, testing on different
hospital datasets is necessary; however our empirical
evidence shows promising results.
Further development will lead to the construction
of an automated ED admission prediction system
based on the SVM approach. Due to the violation of
stationarity conditions, ARIMA ED admission
predictive models have to be regularly re-generated
in order to be useful (Sun et al., 2009). This can be
due to the frequent variability of factors which
influence ED arrivals. Changing emergency care
patterns, notably a higher percentage of medium and
high clinical severity cases are likely to lower the
time series accuracy. This variability would also
affect the SVM model non short-term predictions.
Hence, this system will automatically re-calculate
the SVM model frequently and produce daily
forecasts, as this is easily achievable with the Weka
software package.
4 CONCLUSIONS
Roca and Vilardell have shown that for certain
datasets, emergency ward arrivals do not follow a
Poisson distribution, are self-similar and have a
fractal nature (Monte et al., 2002) over long periods
of time. Constant variance assumptions do not apply
and therefore the process cannot be assumed to be
stationary. Furthermore, the usage of queue and
Markov chain models, which are widely used in ED
computer simulations, is likely to yield inadequate
results when compared with actual ED patient flow.
Although a reasonable accuracy has been
achieved for short-term predictions in our dataset,
the practical applicability of time of both ARIMA
and SVM-based time series models presented in this
paper is nevertheless problematic since neither of
these is likely to successfully predict “burst” or
periods of high demand, where predictions are most
needed (Jones et al., 2002). However, the SVM
approach is still more likely to yield better results as
a “burst” mode may be included with extra
independent variables and non-linear modeling.
Models able to stratify admission predictions in
severity levels are more useful for healthcare
management. An hourly model would also allow for
better crowding management and prediction. Also,
the SVM approach should be compared to more
sophisticated time series models which can be fit to
high volatility periods such as GARCH and its
variations. Further research will try to address these
issues.
REFERENCES
Monte, E., Roca, J. and Vilardell, L. On the self-similar
distribution of the emergency ward arrivals time
series. Fractals-an Interdisciplinary Journal on the
Complex Geometry 10, 413-428 (2002).
Wargon, M., Guidet, B., Hoang, T. D. and Hejblum, G. A
systematic review of models for forecasting the
number of emergency department visits. Emerg Med J
26, 395-399, doi:26/6/395 [pii] (2009).
Batal, H., Tench, J., McMillan, S., Adams, J. and Mehler,
P. S. Predicting patient visits to an urgent care clinic
using calendar variables. Academic Emergency
Medicine 8, 48-53 (2001).
Jones, S. A., Joy, M. P. and Pearson, J. Forecasting
demand of emergency care. Health Care Management
Science 5, 297-305 (2002).
Schweigler, L. M. et al. Forecasting models of emergency
department crowding. Acad Emerg Med 16, 301-308,
doi:ACEM356 [pii] (2009).
Metzger, K. B. et al. Ambient air pollution and
cardiovascular emergency department visits.
HEALTHINF2013-InternationalConferenceonHealthInformatics
248