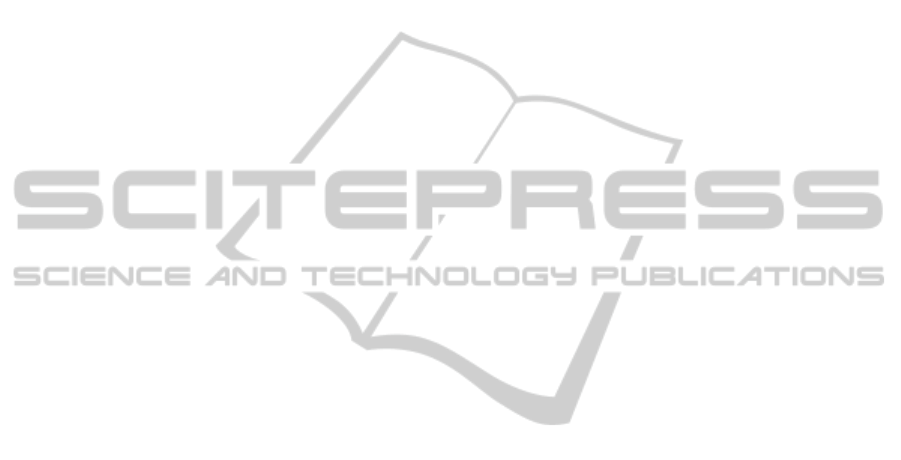
algorithms.
REFERENCES
Le Bihan D., Turner R., Douek P., Patronas N. (1992).
Diffusion MR imaging: clinical applications.
American Journal of Roentgenology,159(3), 591-599.
Basser P. J., Mattiello J., LeBihan D. (1994). Estimation
of the effective self-diffusion tensor from the NMR
spin echo. Journal of Magnetic Resonance - Series B,
103(3), 247-254.
Bammer R. (2003). Basic principles of diffusion-weighted
imaging. European Journal of Radiology, 45(3), 169-
184.
Jones D.K. (2004). The Effect of Gradient Sampling
Schemes on Measures Derived From Diffusion Tensor
MRI: A Monte Carlo Study. Magnetic Resonance in
Medicine. 51(4), 807-815.
Jellison B. J., Field A. S., Medow J., Lazar M., M. Shariar
Salamat and Alexander A. L. (2004). Diffusion Tensor
Imaging of Cerebral White Matter: A Pictorial Review
of Physics, Fiber Tract Anatomy, and Tumor Imaging
Patterns. American Journal of Neuroradiology. 25,
356–369.
Masutani Y., Aoki S., Abe O., Hayashi N., Otomo K.
(2003). MR diffusion tensor imaging: recent advance
and new techniques for diffusion tensor visualization.
European Journal of Radiology, 46, 53-/66.
Madi, S., Hasan, K. M., Narayana, P. A. (2005). Diffusion
tensor imaging of in vivo and excised rat spinal cord at
7 T with an icosahedral encoding scheme. Magnetic
Resonance in Medicine, 53, 118-125.
Campbell, J. S., Siddiqi, K., Rymar, V. (2005). Flow-
based fiber tracking with diffusion tensor and q-ball
data: validation and comparison to principal diffusion
direction techniques. Neuroimage; 27(4) 725-736.
Assaf, Y., Freidlin, R. Z., Rohde, G. K., Basser, P. J.
(2004). New Modeling and Experimental Framework
to Characterize Hindered and Restricted Water
Diffusion in Brain White Matter. Magnetic Resonance
in Medicine, 52, 965–978.
Perrin, M., Poupon, C., Rieul, B., Leroux, P. (2005).
Validation of q-ball imaging with a diffusion fibre-
crossing phantom on a clinical scanner. Philosophical
Transactions of the Royal Society B: Biological
Sciences, 360, 881-891.
Descoteaux, M., Angelino, E., Fitzgibbons, S., Deriche, R.
(2007). Regularized, Fast, and Robust Analytical Q-
Ball Imaging. Magnetic Resonance in Medicine, 58,
497–510.
Freidlin, R. Z., Ozarslan, E., Komlosh, M. E., Chang, L.
(2007). Parsimonious Model Selection for DTI Tissue
Segmentation and Classification: Study on Simulated
and Experimental Data. IEEE Transactions on
Medical Imaging,26(11), 1576-1584.
Sundgren, P. C., Dong, Q., Gómez-Hassan, D., Mukherji
(2004). Diffusion tensor imaging of the brain: review
of clinical applications. Neuroradiology,
46, 339-350.
Tuch D. S. (2004). Q-ball imaging. Magnetic Resonance
in Medicine, 52( 6), 1358-1372.
Ozarslan, E., Shepherd, T. M., Vemuri, B. C., Blackband,
S. J. (2006). Resolution of complex tissue
microarchitecture using the diffusion orientation
transform (DOT). Neuroimage, 31(3), 1086-1103.
Tournier, J. D., Calamante, F., Gadian, D. G., Connelly,
A. (2004). Direct estimation of the fiber orientation
density function from diffusion-weighted MRI data
using spherical deconvolution. Neuroimage, 23( 3),
1176-1185.
Parker, G. J., Alexander, D. C. (2005). Probabilistic
anatomical connectivity derived from the microscopic
persistent angular structure of cerebral tissue.
Philosophical Transactions of the Royal Society B:
Biological Sciences, 360, 893-902.
Klimas, A., Gorczewski, K., Klose, U., Drzazga, Z.
(2008). Preparation and validation of an easy-to-make
organic DTI phantom. 41st Polish Seminar on Nuclear
Magnetic Resonance and Its Applications.
Reese, T. G., Heid, O., Weisskoff, R. M., Wedeen, V. J.
(2003). Reduction of eddy-current-induced distortion
in diffusion MRI using a twice-refocused spin echo.
Magnetic Resonance in Medicine, 49(1), 177-182.
Gorczewski K. (2010). Multi-directional diffusion
weighted imaging: Implementation, verification and
clinical application, PhD thesis.
McEwen J. D., Wiaux Y. (2011). A novel sampling
theorem on the sphere. IEEE Transactions on Signal
Processing, 59, 5876–5887.
Daducci A., McEwen J., Van De Ville D., Thiran J. P.,
Wiaux Y. (2011). Harmonic analysis of spherical
sampling in diffusion MRI. Proceedings of the
International Society for Magnetic Resonance in
Medicine, 3929.
Gorczewski, K., Mang, S, Klose, U. (2009).
Reproducibility and consistency of evaluation
techniques for HARDI data. Magnetic Resonance
Materials in Physics Biology and Medicine, 22(1), 63-
70.
BIOSIGNALS2013-InternationalConferenceonBio-inspiredSystemsandSignalProcessing
406