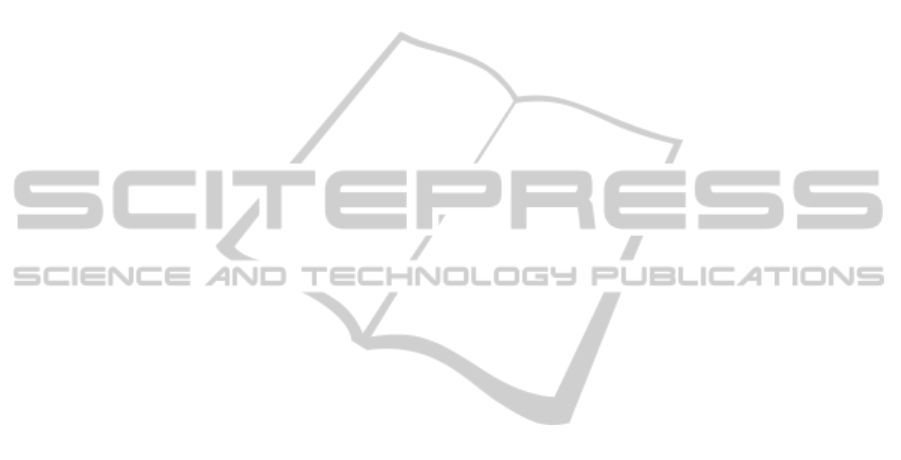
to test the proposed pervasive QoL assessment ap-
proach. Finally, we are planning to assess the validity
of the proposed approach with respect to the tradi-
tional filling of questionnaire.
ACKNOWLEDGEMENTS
The research leading to these results has received
funding from the European Community’s, Seventh
Framework Programme FP7/2007-2013, BackHome
project grant agreement n. 288566.
REFERENCES
Armano, G., de Gemmis, M., Semeraro, G., and Vargiu, E.
(2010). Intelligent Information Access, volume SCI 301.
Springer-Verlag, Studies in Computational Intelligence
series, Heidelberg, Germany.
Brooks, R. (1996). EuroQol: the current state of play.
Health Policy, 37(1):53–72.
Ceccaroni, L. and Subirats, L. (2012). Interoperable knowl-
edge representation in clinical decision support systems
for rehabilitation. International Journal of Applied and
Computational Mathematics, 11(2):303–316.
Cieza, A., Geyh, S., Chatterji, S., Kostanjsek, N.,
¨
Ust¨un, B.,
and Stucki, G. (2005). Icf linking rules: an update based
on lessons learned. Rehabil. Med., 37:212–218.
Clark, R. A., Inglis, S. C., McAlister, F. A., Cleland, J. G. F.,
and Stewart, S. (2007). Telemonitoring or structured
telephone support programmes for patients with chronic
heart failure: systematic review and meta-analysis. BMJ,
334(7600):942.
Daly, J., Armstrong, E., Miralles, F., Vargiu, E., M¨uller-
Putz, G., Hintermller, C., Guger, C., Kuebler, A., and
Martin, S. (2012). BackHome: Brain-neural-computer
interfaces on track to home. In RAatE 2012 - Recent
Advances in Assistive Technology & Engineering.
Geyh, S., Cieza, A., Kollerits, B., Grimby, G., and Stucki,
G. (2007). Content comparison of health-related quality
of life measures used in stroke based on the international
classification of functioning, disability and health (icf): a
systematic review. Quality of Life Research, 16(5):833–
851.
Godoy, D. and Amandi, A. (2005). User profiling in per-
sonal information agents: a survey. Knowl. Eng. Rev.,
20(4):329–361.
Gotay, C. and Moore, T. (1992). Assessing quality of life in
head and neck cancer. Qual Life Res., 1:5–17.
Hays, R., Morales, L., RAND Health, P., and RAND, C.
(2001). The RAND-36 Measure of Health-related Qual-
ity of Life. RAND Health reprint. RAND.
Hitzler, P., Kr¨otzsch, M., Parsia, B., Patel-Schneider, P. F.,
and Rudolph, S., editors (2009). OWL 2 Web Ontology
Language: Primer. W3C Recommendation.
Lerer, L. (2000). The healthcare 2020 platform: The e-
health consumer. PhD thesis, INSEAD.
M¨unssinger, J., Halder, S., Kleih, S., Furdea, A., Raco, V.,
H¨osle, A., and K¨ubler, A. (2010). Brain painting: First
evaluation of a new braincomputer interface application
with als-patients and healthy volunteers. Front Neurosci,
4:182. doi: 10.3389/fnins.2010.00182.
Murphy, B., Herrman, H., Hawthorne, G., Pinzone, T., and
Evert, H. (2000). Australian WHOQoL instruments:
User’s manual and interpretation guide. Australian
WHOQoL Field Study Centre. Melbourne, Australia.
O’Sullivan, S. and Schmitz, T. (2007). Physical Rehabili-
tation. G - Reference, Information and Interdisciplinary
Subjects Series. F.A. Davis.
RAND, C., Health Sciences, P., Hays, R., Sherbourne, C.,
and Mazel, R. (1992). RAND 36-item Health Survey
1.0: RAND Health Sciences Program. RAND reprints.
RAND.
Subirats, L., Ceccaroni, L., and Miralles, F. (2012). Knowl-
edge representation for prognosis of health status in re-
habilitation. Future Internet, 4(3):762–775.
Sutherland, H. and Till, J. (1993). Quality of life assess-
ments and levels of decision making: differentiating ob-
jectives. Qual Life Res, 2(4):297–303.
The Euroqol Group (1990). Euroqol a facility for the mea-
surement of health-related quality of life. Health Policy,
16:199–208.
Vargiu, E., Miralles, F., Martin, S., and Markey, D. (2012).
BackHome: Assisting and telemonitoring people with
disabilities. In RAatE 2012 - Recent Advances in As-
sistive Technology & Engineering.
Ware, J., Kosinski, M., and Dewey, J. (2001). How to Score
Version 2 of the SF-36 Health Survey: Standars & Acute
Forms. QualityMetric.
WHO (2007). Global Age-Friendly Cities: A Guide. Non-
serial Publication. World Health Organization.
Williams, A., of York. Centre for Health Economics, U.,
Consortium, H. E., and of York. NHS Centre for Re-
views & Dissemination, U. (1995). The Measurement
and Valuation of Health: A Chronicle. Centre for Health
Economics discussion paper. University of York, Centre
for Health Economics.
UserProfilingofPeoplewithDisabilities-AProposaltoPervasivelyAssessQualityofLife
357