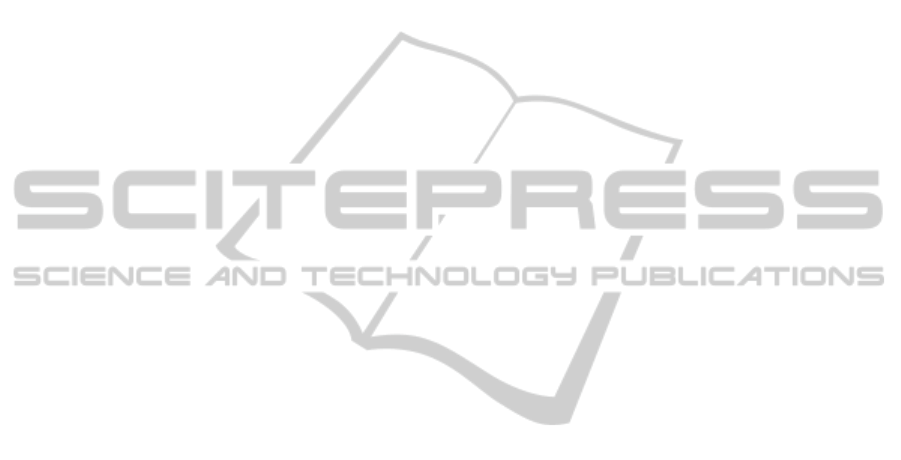
higher number of seeds of different types of species in the field of view of the camera
and therefore contributes to increase the throughput of the monitoring.
4 Conclusions
In this work we have presented an extension of the partial differential equation of [5].
In our case, a detection of the part which requires to be diffused is first performed and
the partial differential equation is then applied locally only in these parts. This presents
the interest to speed up the diffusion process by comparison to a global approach. This
also avoids diffusion artefacts in areas where no diffusion was needed. Application of
our PDE was given here for illustration in the domain of plant science with crossing
seedlings during their elongation stage. In our case the segmentation of the crossing
seedlings was obtainable from a simple threshold and the partial differential equation
was only applied in the crossings area to separate the seedlings. However, for some
species with very thin roots, the contrast between background and seedlings may not
be so favorable and it could be interesting to apply the oriented anisotropic diffusion
locally in the whole image to separate seedling around crossings and also to restore the
edges along the curvated roots in non crossing areas. Plants are highly anisotropic struc-
tures organized along branching structures. Plants growth or plant pathogens spreading
along these structures therefore constitute a natural field of applications for anisotropic
diffusion with PDE to analyze or modelize these spatio-temporal phenomenon.
Acknowledgements
The work received support from the ANR in the framework of the AKER Investissements
d’Avenir project. Landry BENOIT thanks Angers Loire M
´
etropole and SNES-GEVES
for financial support of his PhD Grant.
References
1. Belin, E., Rousseau, D., Rojas-Varela, J., Demilly, D., Wagner, M., Cathala, M., and Durr, C.
(2011). Thermography as non invasive functional imaging for monitoring seedling growth.
Computers and Electronics in Agriculture, 79:236–240.
2. B
¨
odvarsson, B., Klim, S., Morkebjerg, M., Yoon, S. M. C., Chen, J., Maclaren, J., Luther, P.,
Squire, J., Bones, P., and Millane, R. (2008). A morphological image processing method for
locating myosin filaments in muscle electron micrographs. Image and Vision Computing,
26:1073–1080.
3. Chainais, P. and Lebental, B. (2011). Caract
´
erisation statistique d’une assembl
´
ee de nan-
otubes en imagerie microscopique. Actes du 23
`
eme Colloque GRETSI sur le Traitement du
Signal et des Images, Bordeaux, France, 8-11 sept. 2011.
4. Frangi, A., Niessen, W., Vincken, K., and Viergever, M. (1998). Multiscale vessel enhance-
ment filtering. Lecture Notes in Computer Science, Springer, Berlin, pages 130–137.
5. Histace, A., M
´
enard, M., and Cavaro-M
´
enard, C. (2009). Selective diffusion for oriented
pattern extraction: Application to tagged cardiac MRI enhancement. Pattern Recognition
Letters, 30:1356–1365.
8