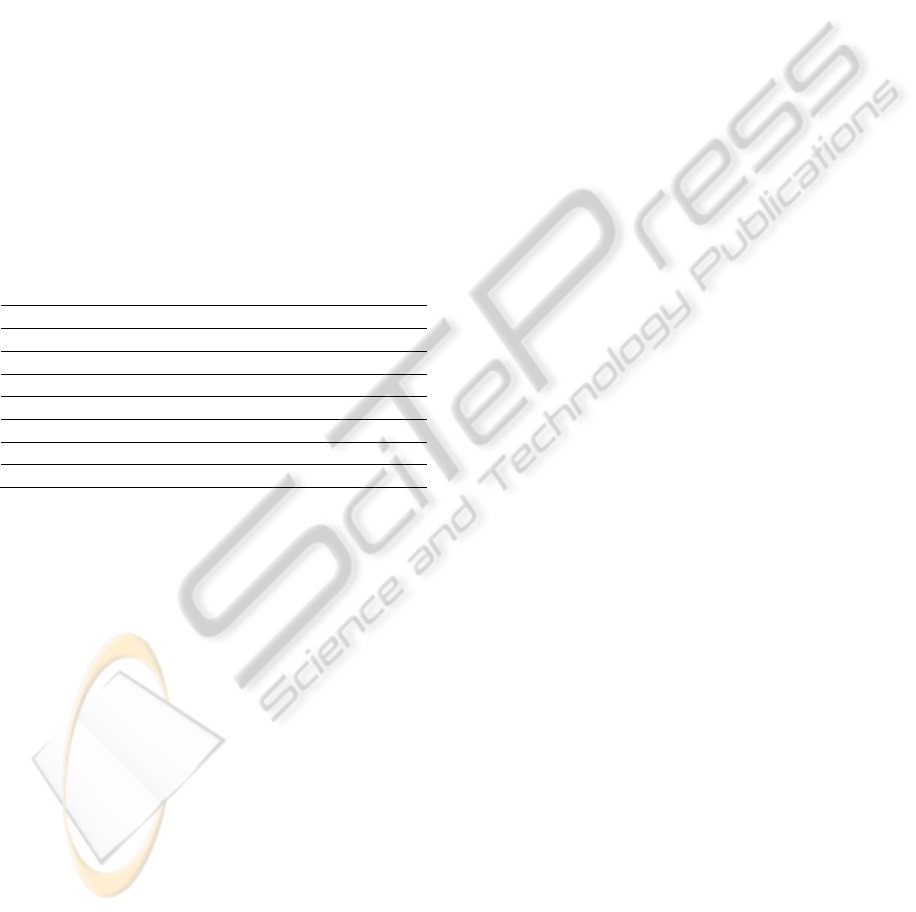
sole microRNA and sole mRNA datasets in general.
When we examined the results, we also noticed that
every selection method we used tends to chose a
mixture of features as best features from both
miRNA and mRNA but never from only one.
The best classification accuracy we obtained is
96.6% (ANN with SVM Attribute selection). This
result is better than the best LOOCV performance on
the same dataset in the literature, which was reported
as 95.8% by Peng et al. (2009). LOOCV accuracy
comparison of multi-class classification using the
GCM datasets is given in Table 2. In addition to
performance comparison of the datasets, we
compared the performances of the classifiers on
these experiments. According to the results ANN
performed best followed by SVM. But SVM have a
larger optimization capacity and much faster training
performance so they can yield better accuracies in
our future work.
Table 2: Comparison with other results (LOOCV accuracy
of cancer classification on GCM datasets).
Studies Accuracy (%)
Ramaswamy et al., 2001 78.0
Su et al., 2003 81.3
Peng et al., 2003 85.2
Lin et al., 2006 84.3
Liu and Xu, 2009 91.8
Peng et al., 2009 95.8
This study 96.6
4 CONCLUSION AND FUTURE
WORK
We evaluate to what extend the integration of
microRNA and mRNA expression data can improve
the prediction accuracy of multi-category cancer
classifiers. Based on the results of a rigorous
experimental study, we have shown that with proper
feature selection strategies, the integration of
microRNA and mRNA data by feature-level fusion
can significantly improve the prediction
performance and provide better classification
accuracy than single use of mRNA and microRNA
data.
Later on this study, we will continue to optimize
the feature selection and classification methods for
better accuracy. We will also be working with
different datasets comprising paired microRNA and
mRNA expression profiles over diseased samples.
We will especially focus on predicting subtypes of
vital cancers. Another future direction is to compare
the performance of potential knowledge-driven
feature selection methods with data-driven methods
used here. We aim to come up with an integrated
hybrid solution for cancer classification and provide
a web server for the use of biomedical researcher
working in this domain.
ACKNOWLEDGEMENTS
This study was supported by the Scientific and
Technological Research Council of Turkey
(TUBİTAK) under the Project 110E160.
REFERENCES
Bartel, D. P., 2004. MicroRNAs: genomics, biogenesis,
mechanism, and function. Cell, 116, 281-297.
Bishop C. M., 2006. Pattern Recognition and Machine
Learning. Springer-Verlag New York, NJ, USA.
Cai, Z., Goebel, R., Salavatipour, M. R., and Lin, G.,
2007. Selecting dissimilar genes for multi-class
classification, an application in cancer subtyping.
BMC Bioinformatics, 8, 206.
Caruana, R., Niculescu-Mizil, A., 2006, An Empirical
Comparison of Supervised Learning Algorithms,
Proceedings of the 23rd International Conference on
Machine Learning, Pittsburgh, PA.
Chan, E., Patel, R., Nallur, S., Ratner, E., Bacchiocchi, A.,
Hoyt, K., Szpakowski, S., Godshalk, S., Ariyan, S.,
Sznol, M., Halaban, R., Krauthammer, M., Tuck, D.,
Slack, F.J., Weidhaas, J.B., 2011. MicroRNA
signatures differentiate melanoma subtypes, Cell
Cycle, 10, 1845-1852.
Guyon, I., Weston, J., Barnhill, S., and Vapnik, V., 2002.
Gene selection for cancer classification using support
vector machines. Machine Learning, 46, 389-422.
Hall, M. A., 1998. Correlation-based Feature Subset
Selection for Machine Learning. PhD Thesis,
Hamilton, New Zealand.
Huopaniemi, I., Suvitaival, T., Nikkilä, J., Orešič, M.,
Kaski, S., 2010. Multivariate multi-way analysis of
multi-source data. Bioinformatics, 26, i391-i398.
Klami, A., Kaski, S., 2008. Probabilistic approach to
detecting dependencies between data sets.
Neurocomputing, 72, 39-46.
Lin, T. C., Liu, R. S., Chen, C. Y., Chao, Y. T., and Chen,
S.Y., 2006. Pattern classification in DNA microarray
data of multiple tumor types. Pattern Recognit., 39,
2426-2438.
Liu, K. H., and Xu, C. G., 2009. A genetic programming-
based approach to the classification of multiclass
microarray datasets. Bioinformatic,s 25, 331-337.
Liu, K. H., and Xu, C. G., 2009. A genetic programming-
ICPRAM2013-InternationalConferenceonPatternRecognitionApplicationsandMethods
506