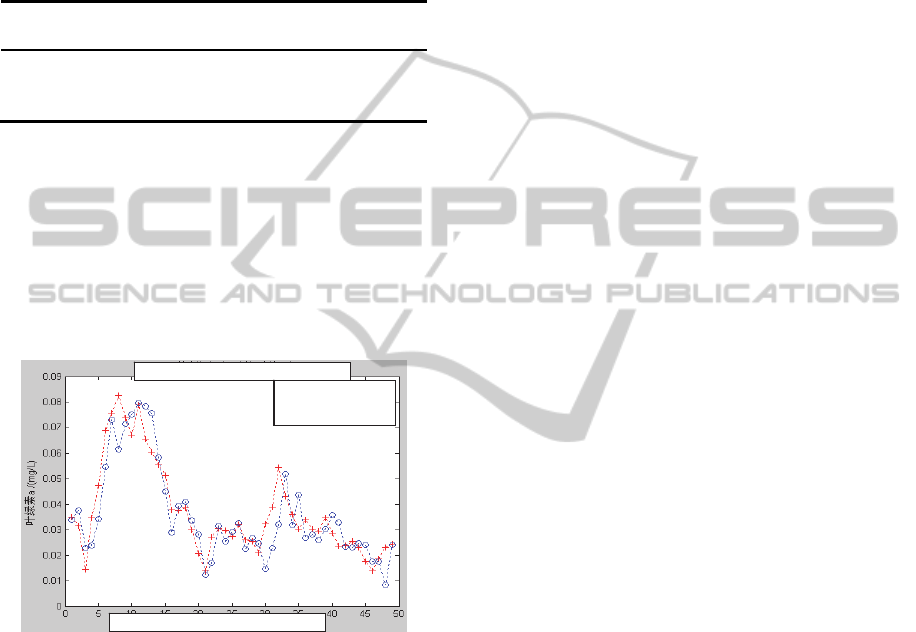
function generalization ability is greatly enhanced;
RBF neural network is widely used which has good
prediction accuracy in short-term water bloom
prediction. But
as the prediction period increases, its prediction
accuracy will be affected to some extent.
Table 2: Prediction accuracy comparison between LSSVM,
SVM and RBF.
Prediction
accuracy
LSSVM SVM RBF
Chl_a value two
days later after
prediction
94. 23%
82.
64%
72.
58%
From Table 2, prediction accuracy of LSSVM is
higher than that of SVM whose prediction accuracy
is higher than RBF neural network. LSSVM is
improved based on SVM in algorithm so that its
function generalization ability is greatly enhanced;
RBF neural network is widely used which has good
prediction accuracy in short-term water bloom
prediction. But as the prediction period increases, its
prediction accuracy will be affected to some extent.
Figure 4: Chl-a value two days later in SVM.
5 CONCLUSIONS
After analyzing and discussing the main factors, two
kinds of short-term and medium-term intelligent
predicting models of water bloom based on RBF
neural networks and LSSVM respectively
researched, and also analyzed and compared with
each other.
First, short- term predict method of water bloom
based on RBF network is put forward, including
research on the monitoring learning algorithms to
the center, width and weight of basis function of
RBF network, as well as error-corrected algorithm
based on gradient descent. The function and
influence, which the number of RBF hidden level
nodes brings to the performance of network, are
analyzed; the width of RBF and fitting and
generalization abilities of network are analyzed and
compared. According to the training and predict
results, the short- term change of Chl-a can be
predicted by using RBF neural network; soft sensing
model of water bloom based on RBF has strong
generalization ability, high predict precision and
good fitting performance, so that an newly effective
method can be provided to predict water flower in
short time.
Then LSSVM is approached, which improves the
algorithm of SVM., and it needs a small amount of
samples, has long-term prediction period, strong
generalization ability and high prediction accuracy.
From the results of models, the fitting precision of
models is relatively good, and it can better predict
the medium-term change rule of Chlorophyll and
provide a new efficient way for water bloom
medium-term intelligent prediction.
ACKNOWLEDGEMENTS
Supported by Beijing Natural Science Foundation
(8101003), National Natural Science Foundation of
China (51179002), and the Beijing Municipal
Commission of Education (PHR201007123,
PHR201008238) and the Beijing Municipal
Commission of Education Science and Technology
Foundation Project.
REFERENCES
Welch E B. Spyridakis D E. Shuster J. Declining lake
sediments phosphorus release and oxygen diversion
Journal of Water Pollution Control Fedration . 1986.
58(1) :92-96.
Somlyody L. Eutrophication modeling, management and
decision making: the Kis-Balaton case. Water Science
and Tecnology, 1998,37(3):165-175.
Vollenweider R A. Input-Output Models with Special
Reference to the Phosphorus Loading Concept in
Limnology. Schweizeische Zeitschrift Hydrol,
1975,37:53—84.
Guisen Du, Yumei Wu, Zhongshan Yang, etc. Analysis of
Water Quality on Urban Rivers and Lakes in Beijing,
Journal of lake sciences, 2005, 17(4):373 - 377.
Qing Liu. Rough set and rough reasoning, Beijing Science
Press. Beijing. 2001, 12-15.
Wu H J, Lin Z Y and Guo S L. Application of artificial
neural network in predicting resources and environment.
Resource and Environment in the Yangtze Basin,
Prediction value via ever
da
Real value and
rediction value
+ Actual Value
○ Predict Value
ICAART2013-InternationalConferenceonAgentsandArtificialIntelligence
596