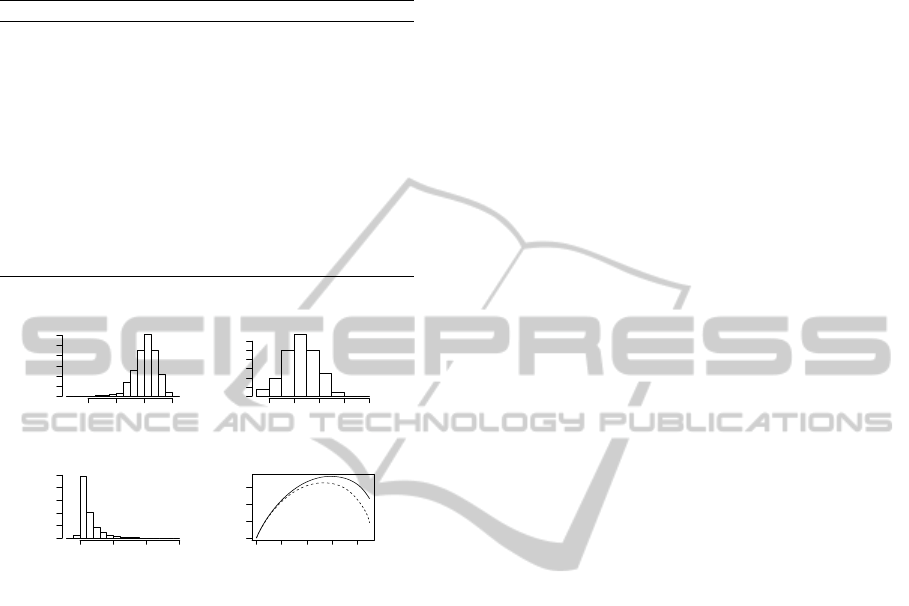
Table 2: Descriptive statistics of the campaign data set, note
that k
′
= 1 represents household insurance coverage and that
k = 2 represents car insurance coverage.
Constant Min Max Mean
ˆ
λ
i1
- 0.0083 3.92 0.64
ˆ
θ
01
1.12 - - -
l
{A
i2
}
- 0 1 0.040
ˆp
i2
- 0.0040 0.13 0.069
ˆ
θ
i2
- 0.71 2.05 0.95
ˆ
θ
02
0.91 - - -
ˆ
λ
2
0.375 - - -
ˆm
2
($) 2, 025 - - -
ˆ
π
2
($) 949 - - -
ˆ
ω
2
($) 15 - - -
ˆe
2
3 - - -
Histogram of µ
ik
(0)
µ
ik
Frequency
−40 −20 0 20
0 400 800 1200
Histogram of µ
ik
(c
ik
*
)
µ
ik
(c
ik
*
)
Frequency
Histogram of c
ik
*
c
ik
*
Frequency
0.0 0.5 1.0 1.5
0 1000 2000
0 1000 3000
0 5000 15000
Number of contacts
Expected profit, µ
ik
Figure 1: Top left is a histogram over expected profits asso-
ciated with no price change µ
ik
(0), top right is a histogram
over expected optimal profits µ
ik
c
∗
ik
, bottom left is a his-
togram of the optimal price changes c
∗
ik
and the bottom right
graph shows cumulative sorted sums
l
∑
i=1
ˆµ
i2
(dotted) and cu-
mulative sorted sums
l
∑
i=1
ˆµ
∗
i2
(solid).
sociated with c
ik
= 0 this is 2647, while applying the
optimal price change to each customer c
∗
ik
, results in
2934 customers having a positiveexpected profit. The
resulting total expected profit from these 2647 and
2934 customers is $16, 416 and $18, 338, an increase
of almost 12%. The average value of price changes is
calculated to 0.13, i.e. the optimal prices offered to
the cross-sale customers is 13% above the tariff price.
6 CONCLUSIONS
In this paper, we have derived a closed form expres-
sion for the customer specific optimal price change
and the corresponding optimal profit. The staring
point is the model of Kaishev et al. (2012) which has
been generalised towards a situation where the prob-
ability of success, of a cross-sale attempt, is depen-
dent of the price at which the product is offered. The
new approach is tested on data from a major Scan-
dinavia insurance company where the target group of
customers (associated with positive expected profit)
increase as optimal price changes are applied. The re-
sulting formula for optimal price change is not only of
interest to researcher and practitioners of cross-selling
but could be applied in a far broader context of pricing
in general.
REFERENCES
Bult, J. R. and Wansbeek, T., 1995. Optimal selection for
direct mail, Marketing Science, 14(4), 378-394.
B¨uhlmann, H. and Gisler, A., 2005. A Course in Credi-
bility Theory and its Applications (Berlin, Germany:
Springer Verlag).
Englund, M., Gustafsson, J., Nielsen, J. P. and Thuring, F.,
2009. Multidimensional Credibility with Time Effects
- An Application to Commercial Business Lines, The
Journal of Risk and Insurance, 76(2), 443-453.
G¨on¨ul, F. F. and Hofstede, F.T., 2006. How to compute
optimal catalog mailing decisions, Marketing Science,
25(1), 65-74.
Kaishev, V. K., Nielsen, J. P., Thuring, F. 2012. Opti-
mal customer selection for cross-selling of financial
services products, Expert Systems with Applications,
forthcoming.
Kamakura, W.A., 2007. Cross-Selling: Offering the Right
Product to the Right Customer at the Right Time in
Lehzan Aksoy, Timothy Keiningham and David Be-
jou (Eds.) Profit Maximization through Customer Re-
lationship Marketing , Haworth Press.
Kamakura, W. A., Kossar, B. and Wedel, M., 2004. ”Iden-
tifying innovators for the cross-selling of new prod-
ucts,” Management Science, 50, 1120-1133
Kamakura, W. A., Wedel, M., de Rosa, F. and Mazzon, J.
A., 2003. Cross-selling through database marketing: a
mixed data factor analyzer for data augmentation and
prediction, International Journal of Research in Mar-
keting, 20(1), 45-65.
Kamakura, W. A., Ramaswami, S. and Srivastava R., 1991.
Applying latent trait analysis in the evaluation of
prospects for cross-selling of financial services, Inter-
national Journal of Research in Marketing, 8, 329-
349.
Knott A., Hayes, A. and Neslin, S. A., 2002. Next-product-
to-buy models for cross-selling applications, Journal
of Interactive Marketing, 16(3), 59-75.
Li, S., Sun, B. and Montgomery, A. L. 2010. Cross-Selling
the Right Product to the Right Customer at the Right
Time, Journal of Marketing Research, 48(4), 683-700.
Li, S., Sun, B. and Wilcox, R. T., 2005. Cross-selling se-
quentially ordered products: an application to con-
sumer banking services, Journal of Marketing Re-
search, 42, 233-239.
SegmentingandSelectingCross-saleProspectsusingDynamicPricing
305