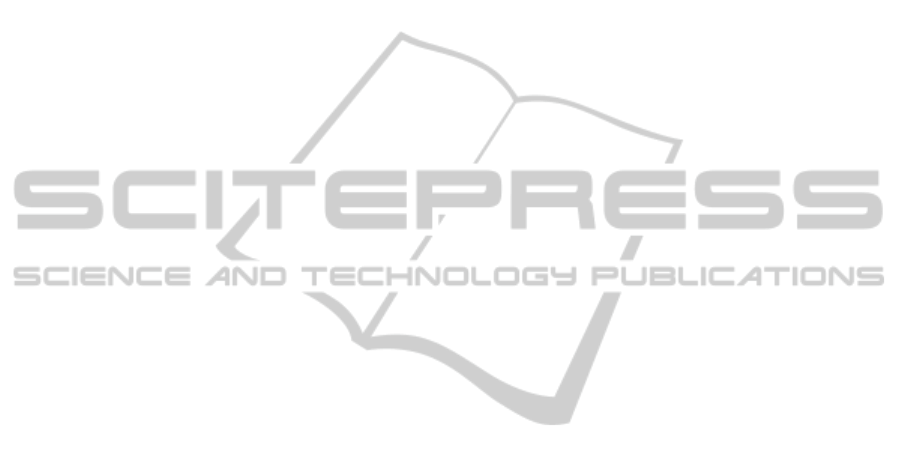
7 CONCLUSIONS
The claim of this paper was to discuss the informed
consent for data release and to point out a social
threat that may stem from some granted patient
consents. A fair consent is proposed to enhance
personalized privacy toward a fair personalized
privacy, hopefully improving privacy protection in
social services. If a privacy-aware system imple-
ments fair personalization, privacy assurance (that
provides how much a party can trust a system as
able to protect privacy) may be enhanced. Patients
who have not enough trust in a health system’s
privacy protection capability might suppress some
relevant information. This could lead to a poor care
treatment and to an increased sanitary risk (e.g., if
an infectious disease is omitted). We also claim that
several implementations of the Fair Consent Principle
are possible and that an acceptable tradeoff between
privacy protection and data utility can be yield if im-
plementations are tailored to the data mining requests.
Further Work. This work, as a position paper, leaves
room for some developments, as for instance specific
implementations, models and policies for a fair pri-
vacy. Let us mention just two scenarios. The one
concerns fair consent policies for health and rights of
donor-conceived children in an ubiquitous computing
environment with weak control on the gathered data
(due to possible data mining in countries with dif-
ferent laws). Such policies involve both technology
and law, technology for effective solutions and for re-
silience to malicious managements. Another scenario
concerns the fair consent model for so called dynamic
data sets gathered from patients monitored via embed-
ded devices during also their movements.
REFERENCES
Applebaum, P. S., Lidz, C. W. and Meisel, A. (1987). In-
formed Consent: Legal Theory and Clinical Practice.
Fair Lawn, NJ: Oxford University Press.
Bertino, E., Ooi, B. C., Yang, Y. and Deng, R. H.(1987).
Privacy and Owneship Preserving of Outsourced
Medical Data. In Proceedings of the 21st Interna-
tional Conference on Data Engeneering (ICDE 2005),
IEEE Conference Publications, 5-8 April 2005.
Brickell, J. and Shmatikov, V. (2008). The Cost of Privacy:
Destruction of Data-Mining Utility in Anonymized
Data Publishing. In Proceedings of KDD’08.
Chen, B.C., Lefevre, K. and Ramakrishnan, R. (2007). Pri-
vacy Skyline: Privacy with multidimensional adversal
knowledge. In Proceedings of the 33rd International
Conference on Very Large Data Bases (VLDB2007).
Chen, B.C., Kifer, D., Lefevre, K. and Machanavajjhala,
A. (2009). Privacy-Preserving Data Publishing. In
Foundations and Trends in Databases, Vol.2, Issues
1-2.
Chen, K. and Liu, L. (2009). Privacy-preserving Multi-
party Collaborative Mining with Geometric Data Per-
turbation. In IEEE Transactions on Parallel and Dis-
tributed Computing, Vol.XX, No. XX. IEEE.
Cimino, J.J. (1996). Review Paper: Coding Systems
in Health care. In Methods of Information in
Medicine,35, 273-84.
De Capitani di Vimercati, S. and Samarati, P. (2006). Pri-
vacy in the electronic society. In Proceedings of the
International Conference on Information Systems Se-
curity (ICISS 2006), Kolkata, India.
Fung, B. C. M., Wang, K., Chen, R. and Yu, Ph. S. (2010).
Privacy-preserving data publishing: A survey of re-
cent developments. In ACM Comput. Surv., 42(4),
ACM, 1-53.
Li, M., Yu, S., Ren, K. and Lou, W. (2010). Secur-
ing Personal Health Records in Cloud Computing:
Patient-Centric and Fine-Grained Data Access Con-
trol in Multi-owner Settings. In SECURCOMM 2010,
Security and Privacy in Communication Networks,
6th Int. ICST Conference.
Poovammal, E. and Ponnavaikko, M. (2009). Categori-
cal Grading Based Personalized Privacy. Preservation
Against Attacks. World Congress on Engineering,
Vol.1, 22-31.
Ren, Y., Cheng, E., Peng, Z., Huang, X. and Song, W.
(2011). A privacy policy conflict detection method for
multi-owner privacy data protection. In Journal Elec-
tronic Commerce Research, Vol.11, Issue 1, 22-31.
Royal College of Nursing Research Society (2011). In-
formed consent in health and social care research.
RCN guidance for nurses, Second edition. Fair Lawn,
NJ: Oxford University Press.
Solanas A. and Castella-Roca J. (2008). RFID Technology
for the Healthcare Sector. Recent Patents on Electrical
Engineering, vol.1, 22-31.
Xiao, X. and Tao, Y. (2006). Personalized Privacy Preser-
vation. SIGMOD.
RevisitingtheInformedConsentPrincipleforDataRelease
97