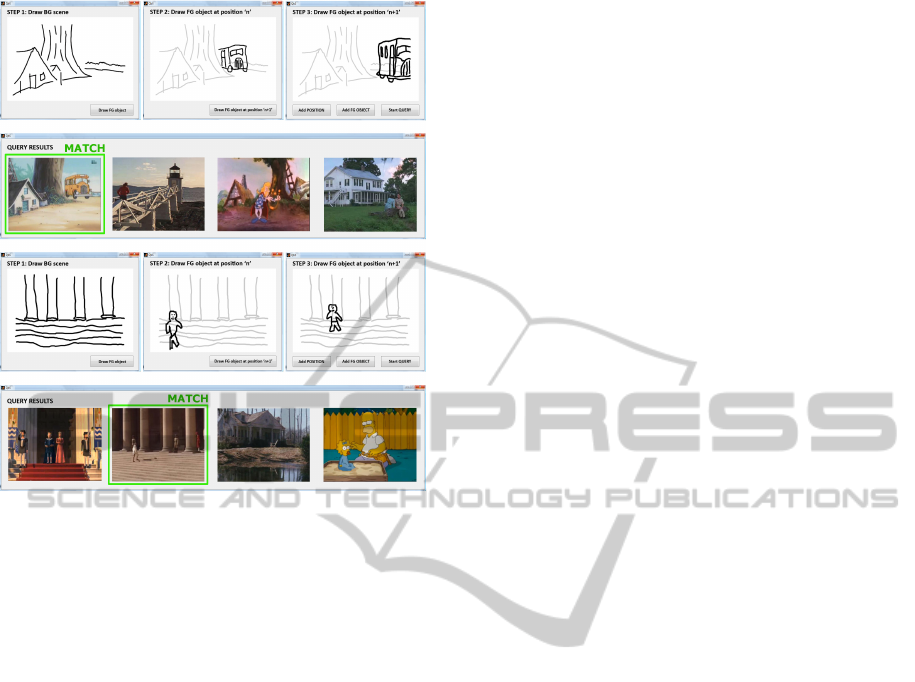
Figure 11: Exemplary animated sketching CBVR results.
results indicate that the proposed system has a posi-
tive usability score, although there are still a number
of areas, such as the user interface, that could be im-
proved. Multiple users also suggested to extend the
system to a hybrid CBVR system that allows them
to search by either text or sketch at any point of the
searching process. This would provide them with a
more flexible and powerful way of searching.
6 CONCLUSIONS
This paper proposes a novel intuitive querying
method to improve content-based searching in dig-
ital video. By animated sketching users can easily
define the spatial and temporal characteristics of the
video sequence they are looking for. To find the best
match for the user input, the proposed algorithm first
compares the edge histogram descriptors of the BG
and FG objects in the sketch and the set of video
sequences. This spatial filtering already results in
sequences with similar scene characteristics as the
sketch. However, to find the sequences in which the
specific sketched action occurs, this set of sequences
is further queried by matching their motion history
values to those of the sketch. The sequences with the
highest match are returned to the user. Experiments
show that the system yields appropriate query results.
REFERENCES
Aslandogan, A. Y. and Yu, C. T. (1999). Techniques and
systems for image and video retrieval. IEEE Transac-
tions on knowledge and data engineering, 11:56–63.
Blank, M., Gorelick, L., Shechtman, E., Irani, M., and
Basri, R. (2005). Actions as space-time shapes. In-
ternational Conference on Computer Vision, pages
1395–1402.
Bobick, A. F. and Davis, J. W. (2001). The recognition of
human movement using temporal templates. Trans-
actions on Pattern Analysis and Machine Intelligence,
23:257–267.
Brahmi, D. and Ziou, D. (2004). Improving cbir systems by
integrating semantic features. Canadian Conference
on Computer and Robot Vision, pages 233–240.
Chang, S. F., Chen, W., and Sundaram, H. (1998). Videoq:
a fully automated video retrieval system using motion
sketches. IEEE Workshop on Applications of Com-
puter Vision.
Collomosse, J., McNeill, G., and Qian, Y. (2009). Story-
board sketches for content based video retrieval. In-
ternational Conference on Computer Vision.
Eitz, M., Hildebrand, K., Boubekeur, T., and Alexa, M.
(2009). A descriptor for large scale image retrieval
based on sketched feature lines. Eurographics Sympo-
sium on Sketch-Based Interfaces and Modeling, pages
29–38.
Laptev, I., M., M. M., Schmid, C., and Rozenfeld,
B. (2008). Learning realistic human actions from
movies. Computer Vision and Pattern Recognition,
pages 1–8.
Lee, A. J. T., Hong, R. W., and Chang, M. F. (2004). An ap-
proach to content-based video retrieval. International
Conference on Multimedia and Expo, pages 273–276.
Otsu, N. (1979). A threshold selection method from gray-
level histograms. Transactions on Systems, Man and
Cybernetics, 9:62–66.
Petkovic, M. and Jonker, W. (2004). Content-Based Video
Retrieval: A Database Perspective, volume 1. Kluwer
Academic Publishers, Norwell, MA, 1st edition.
Sclaroff, S., Cascia, M. L., Sethi, S., and Taycher, L. (1999).
Unifying textual and visual cues for content-based im-
age retrieval on the world wide web. Vision and Image
Understanding, 75:86–89.
Sikora, T. (2001). The mpeg-7 visual standard for content
description - an overview. Transactions on Circuits
and Systems for Video Technology, 11:696–702.
Suma, E. A., Sinclair, C. W., Babbs, J., and Souvenir, R.
(2008). A sketch-based approach for detecting com-
mon human actions. International Symposium on Vi-
sual Computing, pages 418–427.
Tomasi, C. and Manduchi, R. (1998). Bilateral filtering for
gray and color images. International Conference on
Computer Vision, pages 839–846.
Yang, C. C. (2004). Content-based image retrieval: a com-
parison between query by example and image brows-
ing map approaches. Journal of Information Science,
30:254–267.
VISAPP2013-InternationalConferenceonComputerVisionTheoryandApplications
728