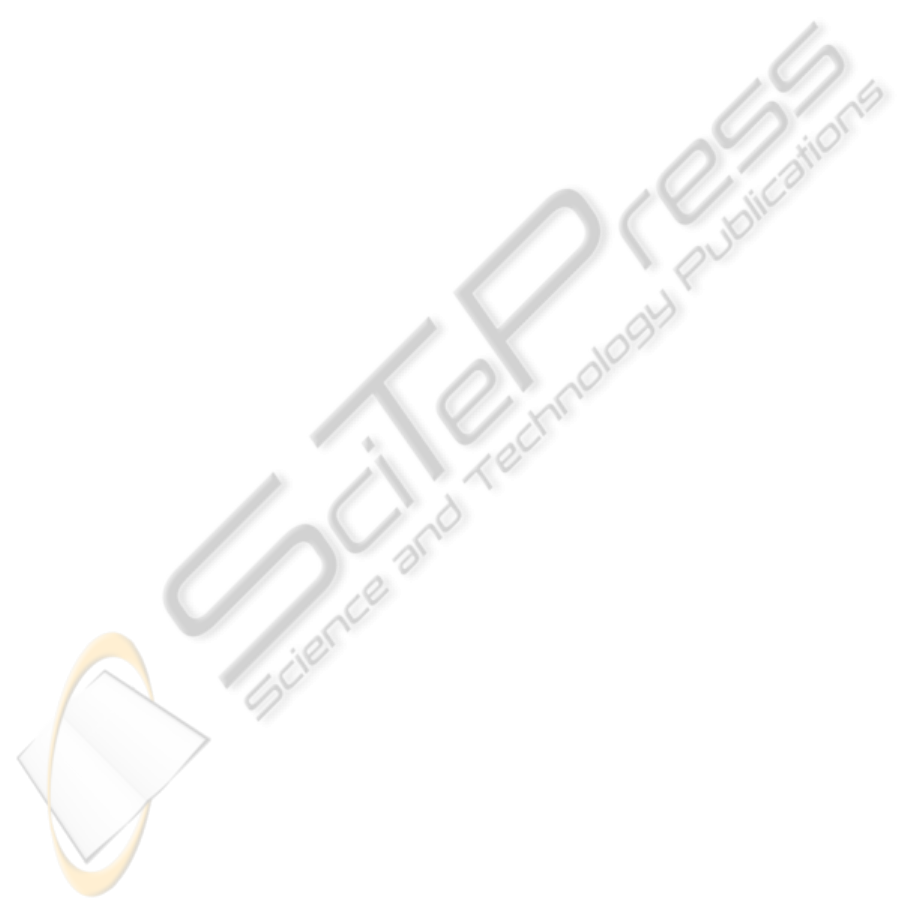
frontal or profile ones and could have varying align-
ments from straight to oblique ones.
Our developed system is based on parametric ac-
tive contours whose innovative automatic initializa-
tion is based on the set of downscaled images and ap-
plies new validation criteria involving skin color and
area information. The evolution of these active con-
tours is guided by the multi-scale, multi-feature vec-
tor flow mechanism which uses the original combi-
nation of edges and regions extracted from the multi-
scale representations of the processed color image.
REFERENCES
Bajpai, S., Singh, A., and Karthik, K. V. (2011). An exper-
imental comparison of face detection algorithms. In
Proceedings of the IEEE International Workshop on
Conference on Machine Vision, pages 196–200.
Bing, X., Wei, Y., and Charoensak, C. (2004). Face contour
extraction using snake. In Proceedings of the IEEE
International Workshop on Biomedical Circuits and
Systems, pages S3.2.5–8.
Charfi, M. (2010). Using the GGVF for automatic initial-
ization and splitting snake model. In Proceedings of
the IEEE International Symposium on I/V Communi-
cations and Mobile Network, pages 1–4.
Gunn, S. R. and Nixon, M. S. (1998). Global and local
active contours for head boundary extraction. Inter-
national Journal of Computer Vision, 30(1):43–54.
Hanmin, H. and Zhen, J. (2008). Application of an im-
proved snake model in face location based on skin
color. In Proceedings of the IEEE World Congress
on Intelligent Control and Automation, pages 6897–
6901.
Harper, P. and Reilly, R. B. (2000). Color based video seg-
mentation using level sets. In Proceedings of the IEEE
International Conference on Image Processing, pages
480–483.
Heiler, M. and Schnoerr, C. (2005). Natural image statistics
for natural image segmentation. International Journal
of Computer Vision, 63(1):5–19.
Hsu, R.-L. and Jain, A. K. (2003). Generating discrimi-
nating cartoon faces using interacting snakes. IEEE
Transactions on Pattern Analysis and Machine Intel-
ligence, 25(11):1388–1398.
Huang, F. and Su, J. (2004). Multiple face contour detection
based on geometric active contours. In Proceedings of
the IEEE International Conference on Automatic Face
and Gesture Recognition, pages 385–390.
Kim, G., Suhr, J. K., Jung, H. G., and Kim, J. (2010). Face
occlusion detection by using B-spline active contour
and skin color information. In Proceedings of the
IEEE International Conference on Control, Automa-
tion, Robotics and Vision, pages 627–632.
Lanitis, A., Taylor, C. J., and Cootes, T. F. (2005). Au-
tomatic face identification system using flexible ap-
pearance models. Image and Vision Computing,
13(5):393–401.
Li, B. and Acton, S. T. (2008). Automatic active model ini-
tialization via Poisson inverse gradient. IEEE Trans-
actions on Image Processing, 17(8):1406–1420.
Li, Y., Lai, J. H., and Yuen, P. C. (2006). Multi-template
ASM method for feature points detection of facial
image with diverse expressions. In Proceedings of
the IEEE International Conference on Automatic Face
and Gesture Recognition, pages 435–440.
Neuenschwander, W., Fua, P., Szekeley, G., and Kubler,
O. (1994). Initializing snakes. In Proceedings of the
IEEE Computer Society Conference on Computer Vi-
sion and Pattern Recognition, pages 658–663.
Ohliger, K., Edeler, T., Condurache, A. P., and Mertins,
A. (2010). A novel approach of initializing region-
based active contours in noisy images by means of
unimodality analysis. In Proceedings of the IEEE In-
ternational Conference on Signal Processing, pages
885–888.
Olszewska, J. I. (2009). Unified Framework for Multi-
Feature Active Contours. PhD thesis, UCL.
Olszewska, J. I. (2012). Multi-target parametric active con-
tours to support ontological domain representation. In
Proceedings of the Conference on Shape Recognition
and Artificial Intelligence (RFIA 2012), pages 779–
784.
Olszewska, J. I. et al. (2007). Non-rigid object tracker based
on a robust combination of parametric active contour
and point distribution model. In Proceedings of the
SPIE International Conference on Visual Communi-
cations and Image Processing, pages 6508–2A.
Olszewska, J. I. et al. (2008). Multi-feature vector flow for
active contour tracking. In Proceedings of the IEEE
International Conference on Acoustics, Speech and
Signal Processing, pages 721–724.
Perlibakas, V. (2003). Automatical detection of face fea-
tures and exact face contour. Pattern Recognition Let-
ters, 24(16):2977–2985.
Pluempitiwiriyawej, C. and Sotthivirat, S. (2005). Active
contours with automatic initialization for myocardial
perfusion analysis. In Proceedings of the IEEE Inter-
national Conference on Engineering in Medicine and
Biology, pages 3332–3335.
Sobottka, K. and Pitas, I. (1996). Segmentation and tracking
of faces in color images. In Proceedings of the IEEE
International Conference on Automatic Face and Ges-
ture Recognition, pages 236–241.
Tauber, C., Batatia, H., and Ayache, A. (2005). A gen-
eral quasi-automatic initialization for snakes: Appli-
cation to ultrasound images. In Proceedings of the
IEEE International Conference on Image Processing,
volume 2, pages 806–809.
Vatsa, M., Singh, R., and Gupta, P. (2003). Face detection
using gradient vector flow. In Proceedings of the IEEE
International Conference on Machine Learning and
Cybernetics, pages 3259–3263.
Wood, R. and Olszewska, J. I. (2012). Lighting-variable
AdaBoost based-on system for robust face detection.
In Proceedings of the International Conference on
Bio-Inspired Systems and Signal Processing, pages
494–497.
BIOSIGNALS2013-InternationalConferenceonBio-inspiredSystemsandSignalProcessing
434