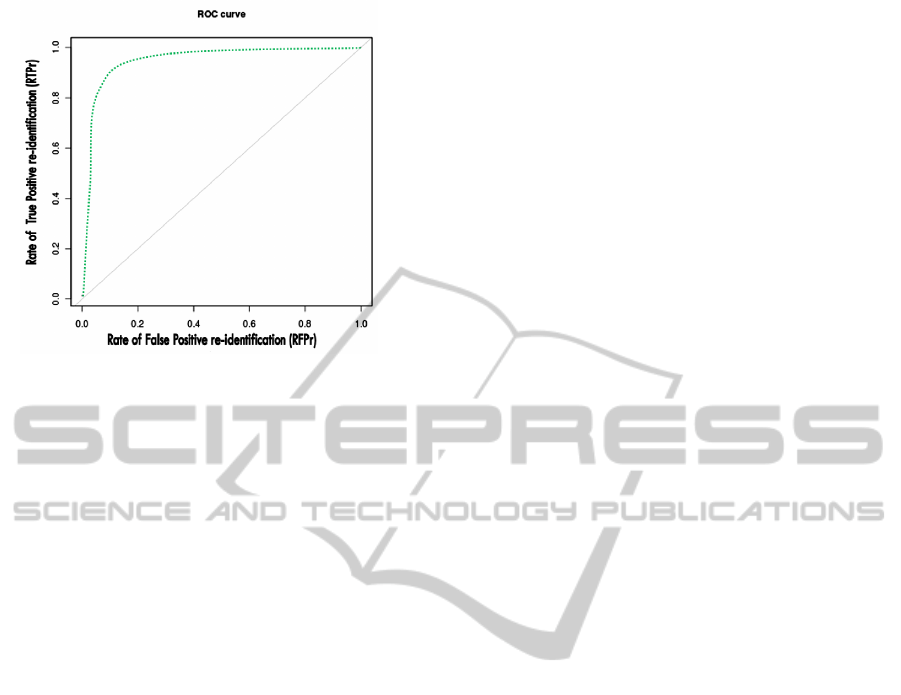
Figure 3: Roc Curve relativeto the variation of the matching
threshold between objects (RTPr in function of RFPr).
7 CONCLUSIONS
In this paper we have presented a new distributed ap-
proach for inter-camera re-identification based on the
consensus principle reached through the collaboration
between smart-cameras. Firstly, we have presented
a completely decentralized system based on the dis-
tributed inferences where each camera is modelled
by an autonomous agents. Secondly, to reach a con-
sensus about objects identities a new distributed ap-
proach was presented based on the collaboration be-
tween agents. The obtained results proved the ro-
bustness of the proposed approach. From this work
we conclude that the decentralisation of the infer-
ences is an important issue to the design of real-time
and robust re-identificationand tracking frameworkin
multi-camera/Multi-sensor network. In the same con-
text we need to developed sophisticated algorithms
able to reach a consensus between smart-cameras. Fu-
ture works including the integration of auctions for
ambiguity management to reach a consensus and im-
proved the inter-camera color mapping through the
use of invariant visual cues inter-camera.
REFERENCES
Bousetouane, F., Dib, L., and Snoussi, H. (2011). Robust
detection and tracking pedestrian object for real time
surveillance applications. In SPIE, volume 8285, page
828508.
Bousetouane, F., Dib, L., and Snoussi, H. (2012). Improved
mean shift integrating texture and color features for
robust real time object tracking. The Visual Computer
J, Springer, pages 1–16.
Chen, K.-W., Lai, C.-C., Lee, P.-J., Chen, C.-S., and
Hung, Y.-P. (2011). Adaptive learning for target track-
ing and true linking discovering across multiple non-
overlapping cameras. Multimedia, IEEE Transactions
on, 13(4):625 –638.
Doretto, G., Sebastian, T., Tu, P., and Rittscher, J. (2011).
Appearance-based person reidentification in camera
networks: problem overview and current approaches.
Journal of Ambient Intelligence and Humanized Com-
puting, 2:127–151.
Farenzena, M., Bazzani, L., Perina, A., Murino, V., and
Cristani, M. (2010). Person re-identification by
symmetry-driven accumulation of local features. In
(CVPR),IEEE Conference on, pages 2360 –2367.
Gilbert, A. and Bowden, R. (2006). Tracking ob-
jects across cameras by incrementally learning inter-
camera colour calibration and patterns of activity. In
Computer Vision ECCV 2006, volume 3952 of LNCS,
pages 125–136. Springer.
Gray, D. and Tao, H. (2008). Viewpoint invariant pedes-
trian recognition with an ensemble of localized fea-
tures. In Proceedings of the 10th ECCV, pages 262–
275. Springer.
Javed, O., Shafique, K., Rasheed, Z., and Shah, M. (2008).
Modeling inter-camera space-time and appearance re-
lationships for tracking across non-overlapping views.
Comput. Vis. Image Underst., 109(2):146–162.
Meden, B., Sayd, P., and Lerasle, F. (2011). Mixed-state
particle filtering for simultaneous tracking and re-
identification in non-overlapping camera networks. In
Image Analysis, LNCS, pages 124–133. Springer.
Motamed, C. and Wallart, O. (2007). A temporal fusion
strategy for cross-camera data association. Pattern
Recognition Letters, 28(2):233–245.
Olfati-Saber, R. and Sandell, N. (2008). Distributed track-
ing in sensor networks with limited sensing range. In
American Control Conference, pages 3157 –3162.
Porikli, F. and Divakaran, A. (2003). Multi-camera calibra-
tion, object tracking and query generation. In ICME
’03. Proceedings., volume 1, pages I – 653–6 vol.1.
Prosser, B., Gong, S., and Xiang, T. (2008). Multi-camera
matching using bi-directional cumulative brightness
transfer functions. In Proceedings of the BMVC, pages
64.1–64.10. BMVA Press.
Soto, C., Song, B., and Roy-Chowdhury, A. (2009). Dis-
tributed multi-target tracking in a self-configuring
camera network. In CVPR 2009. IEEE Conference
on, pages 1486 –1493.
VISAPP2013-InternationalConferenceonComputerVisionTheoryandApplications
346