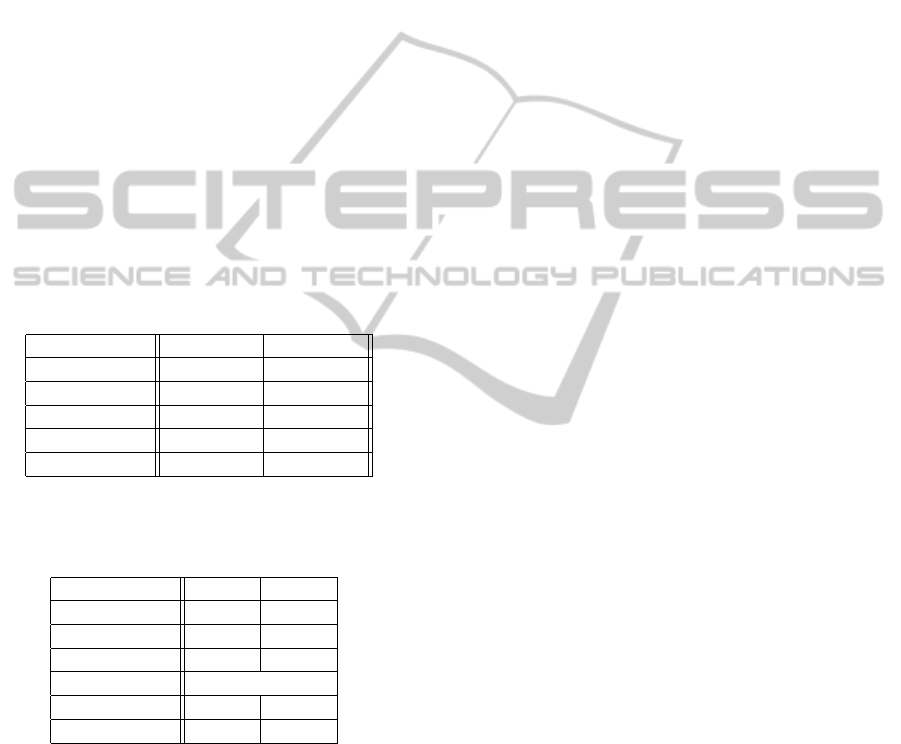
solely by unseen subjects. The results are averaged
over ten random splits. As can be seen, our proposed
method achieved the best performance. We can also
observe that the proposed approach can be superior
to the classical LDE adopting predefined graphs. The
recognition rates were relatively low since the ground
truth data associated with Pointing’04 database were
not accurate.
Table 5 shows the average error in the estimation
of the pitch and yaw angles for the raw images and the
Laplacian of Gaussian transformed images. For both
kinds of images the errors were relatively small given
the fact that the resolution of the pitch and yaw angle
was 15
◦
. We can also observe that (i) for the pro-
posed method, the angle estimation (pitch and yaw)
obtained with the raw images is slightly better than
that obtained with the LOG transformed images, and
(ii) for the classic LDE, the angle estimation based on
the raw images was slightly worse than that based on
the LOG transformed images.
Table 4: Best average classification accuracy (%) on Point-
ing’04 data set for pitch and yaw angles (over 10 random
splits). The training sets contained 20 images.
Pointing’04 Pitch Yaw
PCA 46.5% (70) 47.8% (70)
LPP 45.3% (40) 44.9% (20)
ANMM 48.8% (70) 50.3% (70)
Classic LDE 45.1% (70) 44.7% (70)
Proposed LDE 52.5% (50) 49.9% (30)
Table 5: Average error in estimating the pitch and yaw an-
gles in Pointing’04 database on raw and LOG images (with
20 train image for each class).
Pointing’04 Pitch Yaw
Raw images
Classic LDE 14.12
◦
11.79
◦
Proposed LDE 11.64
◦
10.09
◦
LOG images
Classic LDE 13.86
◦
11.57
◦
Proposed LDE 13.02
◦
11.10
◦
4 CONCLUSIONS
We proposed a self-optimized Local Discriminant
Embedding method. We applied it to the problem
of model-less coarse 3D head pose estimation. We
used the proposed method as a generic (i.e. person-
independent) algorithm for head pose estimation. Un-
like many graph-based linear embedding techniques,
our proposed method does not need user-defined pa-
rameters. Experimental results carried out on the
problem demonstrate the advantage over some state-
of-art solutions and the classic LDE.
REFERENCES
Aghajanian, J. and Prince, S. (2009). Face pose estimation
in uncontrolled environments. In British Machine Vi-
sion Conference.
Belkin, M. and Niyogi, P. (2003). Laplacian eigenmaps
for dimensionality reduction and data representation.
Neural Computation, 15(6):1373–1396.
Chen, H., Chang, H., and Liu, T. (2005). Local discriminant
embedding and its variants. In IEEE International
Conference on Computer Vision and Pattern Recog-
nition.
Dornaika, F. and Ahlberg, J. (2004). Face and facial fea-
ture tracking using deformable models. International
Journal of Image and Graphics, 4(3):499–532.
Dornaika, F. and Davoine, F. (2006). On appearance
based face and facial action tracking. IEEE Transac-
tions on Circuits and Systems for Video Technology,
16(9):1107–1124.
Fu, Y. and Huang, T. (2006). Graph embedded analysis for
head pose estimation. In IEEE International Confer-
ence on Automatic Face and Gesture Recognition.
Guo, G., Fu, Y., Dyer, C., and Huang, T. (2008). Head
pose estimation: Classification or regression? In IEEE
International Conference on Pattern Recognition.
Ma, B., Zhang, W., Shan, S., Chen, X., and Gao, W. (2006).
Robust head pose estimation using lgbp. In Int. Con.
on Patt. Recog. ICPR’06.
Murphy-Chutorian, E. and Trivedi, M. (2009). Head
pose estimation in computer vision: a survey. IEEE
Trans. on Pattern Analysis and Machine Intelligence,
31(4):607–626.
Raytchev, B., Yoda, I., and Sakaue, K. (2004). Head pose
estimation by nonlinear manifold learning. In IEEE
International Conference on Pattern Recognition.
Wang, F., Wang, X., Zhang, D., Zhang, C., and Li, T.
(2009). Marginface: A novel face recognition method
by average neighborhood margin maximization. Pat-
tern Recognition, 42:2863–2875.
Yan, S., Wang, H., Fu, Y., Yan, J., Tang, X., and Huang,
T. (2009). Synchronized submanifold embedding
for person-independent pose estimation and beyond.
IEEE Trans. on Image Processing, 18(1):202–210.
Yan, S., Xu, D., Zhang, B., Zhang, H., Yang, Q., and Lin,
S. (2007). Graph embedding and extension: a gen-
eral framework for dimensionality reduction. IEEE
Trans. on Pattern Analysis and Machine Intelligence,
29(1):40–51.
VISAPP2013-InternationalConferenceonComputerVisionTheoryandApplications
352