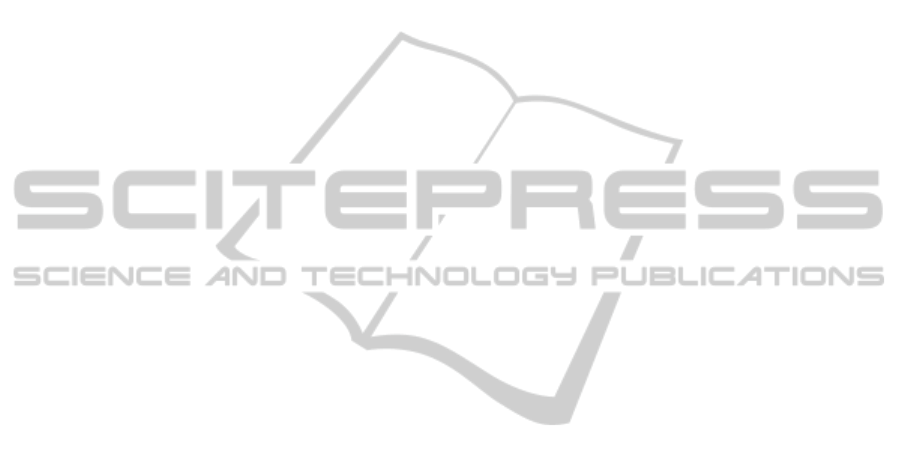
REFERENCES
Argenziano, G., Soyer, H. P., De Giorgi, V., Piccolo, D.,
Carli, P., Delfino, M., Wolf, I. H., (2000). Interactive
atlas of dermoscopy CD: EDRA Medical Publishing
and New Media, Milan.
Argenziano, G., Soyer, H. P., Chimenti, S., Talamini, R.,
Corona, R., Sera, F., Kopf, A. W., (2003).
Dermoscopy of pigmented skin lesions: results of a
consensus meeting via the Internet. Journal of the
American Academy of Dermatology, 48(5), 679-83.
Bickers, D. R., Lim, H. W., Margolis, D., Weinstock, M.
A., Goodman, C., Faulkner, E., Dall T., (2006). The
burden of skin disease: 2004, a joint project of the
American Academy of Dermatology Association and
the Society for Investigative Dermatology. Journal of
the Academy of Dermatology, 55(3), 490-500.
Blois, M. S., (1980). Clinical judgment and computers.
New England Journal of Medicine, 303(4), 192-7.
Burnet, N. G., Jefferies, S. J., Benson, R. J., Hunt, D. P.
and Treasure, F. P., (2005). Years of life lost (YLL)
from cancer is an important measure of population
burden—and should be considered when allocating
research funds. British Journal of Dermatology, 92(2),
241-5.
Caselles, V., Kimmel, R., Sapiro, G. (1997). Geodesic
active contours, International Journal of Computer
Vision, 22(1): 61-79.
Cheng, B., Stanley, R. J., Stoecker, W. V., Stricklin, S.
M., Hinton, K. A. Nguyen, T. K., Moss, R. H. (2012).
Analysis of clinical and dermoscopic features for basal
cell carcinoma neural network classification. In press,
Skin Research and Technology.
Drugge, R. J., Nguyen, C., Gliga, L. and Drugge, E. D.
(2010). Clinical pathway for melanoma detection
using comprehensive cutaneous analysis with
Melanoscan. Dermatology Online Journal, 16(8), 1.
Guvenc, P., LeAnder, R. W., Kefel, S., Stoecker, W. V.,
Rader, R. K., Hinton, K. A., Moss, R. H. (2012) Sector
expansion and elliptical modeling of blue-gray ovoids
for basal cell carcinoma discrimination in dermoscopy
images. In press, Skin Research and Technology.
Kefel, S., Guvenc, P., LeAnder, R., Stricklin, S. M.,
Stoecker, W. V. (2012). Discrimination of basal cell
carcinoma from benign lesions based on extraction of
ulcer features in polarized-light dermoscopy images.
Skin Research and Technology, 18(4), 471-5.
Menzies, S. W., Kreusch, J., Byth, K., Pizzichetta, M. A.,
Marghoob, A., Braun, R, Johr, R. (2008).
Dermoscopic evaluation of amelanotic and
hypomelanotic melanoma. Archives of Dermatology,
144(9), 1120-7.
Perona, P. and Malik, J. (1990). Scale-space and edge
detection using anisotropic diffusion. In IEEE
Transactions on Pattern Analysis and Machine
Intelligence, 12(7), 629-39.
Salama, A. K., Rosa, N. D., Scheri, R. P., Herndon, J. E.,
Tyler, D. S., Marcello, J., Abernethy, A. P. (2012).
The effect of metastatic site and decade of diagnosis
on the individual burden of metastatic melanoma:
contemporary estimates of average years of life lost.
Cancer Investigation, 30(9), 637-41.
Slue, W., Kopf, A.W. and Rivers, J. K., (1988). Total-
body photographs of dysplastic nevi. Archives of
Dermatology, 124(8), 1239-43.
Stanley, R. J., Stoecker, W. V., Moss, R. H., Gupta, K.,
Jella, P. (2005). Color and structural features for
automatic skin lesion discrimination in dermoscopy
images. 6th World Congress on Melanoma,
Vancouver, BC, September 7, 2005.
Stricklin, S. M., Stoecker, W. V., Malters, J. M., Drugge,
R., Oliviero, M. & Rabinovitz, H. S., (2012).
Melanoma in situ in a private practice setting 2005
through 2009: Location, lesion size, lack of concern.
Journal of the American Academy of Dermatology,
67(3), e105-9.
VISAPP2013-InternationalConferenceonComputerVisionTheoryandApplications
508