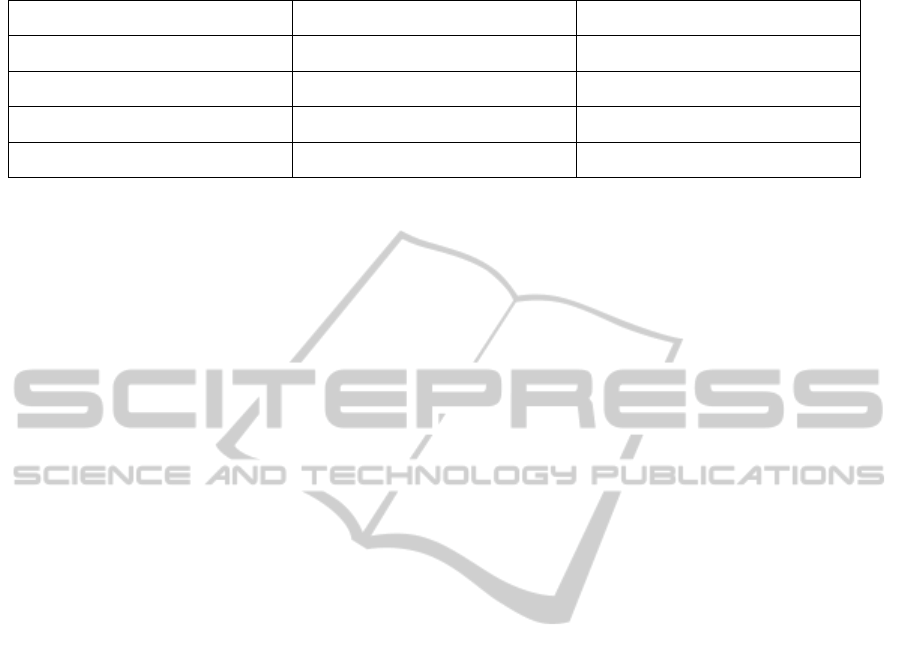
Table 3: Experimental Result.
Precision ratio (%) Recall ratio (%)
Name Similarity 44.44 80
Structure Similarity 50 90
Individual Similarity 27.78 100
Compositive Similarity 94.44 94.44
seen that, compositive measure we proposed
performs well in heterogeneous ontology mapping in
material domain.ontology mapping in material
domain.
5 CONCLUSIONS
The experiments show that compositive measure
achieves higher precision ratio and recall ratio
compared to single similarity measure. We can
conclude that compositive similarity measure is
suitable for heterogeneous ontology mapping in
material domain. In addition, our work will be
developed further in the future: In the Ontology
building section : Rules should be improved in
order to realize automatic mapping between
relational database and OWL ontology in material
domain. In the ontology mapping section:For the
word which is not included in WordNet, word
segmentation should be done before similarity
computing. Firstly, in ontology construction section:
rules should be improved in order to realize
automatic mapping between relational database and
OWL ontology in material domain. Secondly, in the
ontology mapping section: the word which is not
included in WordNet, word segmentation should be
done before similarity computing.
ACKNOWLEDGEMENTS
Supported by the Key Science-Technology Plan of
the National ‘Eleventh Five-Year-Plan’ of China
under Grant No. 2011BAK08B04, the R&D
Infrastructure and Facility Development Program
under Grant No. 2005DKA32800, and the 2012
Ladder Plan Project of Beijing Key Laboratory of
Knowledge Engineering for Materials Science under
Grant No. Z121101002812005.
REFERENCES
Budanitsky A., Hirst G. 2006. Evaluating WordNet-based
Measures of Lexical Semantic Relatedness.
Computational Linguistics, 32(1), 13-47.
Cullot N., Ghawi R., Yétongnon K. 2007. DB2OWL: A
Tool for Automatic Database to Ontology Mapping In:
Ceci et al., eds. Proceedings of the 15th Italian
Symposium on Advanced Database Systems, Torre
Canne, Italy. SEBD, 491-494.
Doan A., Madhavan J., Dhamankar R., Domingos P.,
Halevy A. 2003. Learning to match ontologies on the
Semantic Web. The VLDB Journal, 12(4) pp.303-319.
Ehrig, M., Staab, S. 2004. QOM - Quick Ontology
Mapping In: S. A. Mcllraith, D. Plexousakis, F. V.
Harmelen, eds. 3th International Semantic Web
Conference, Hiroshima, Japan, November 7-11, 2004.
Berlin: Springer Berlin Heidelberg, 683-697.
Euzenat J, Valtchev P.2004. Similarity-based ontology
alignment in OWL-lite In: R. Lopez and L. Saitta, eds.
Proceedings of the European Conference on Artificial
Intelligence, Valencia, Spain, August 22-27, 2004.
Amsterdam: IOS Press, 333-337.
Hu W., Jian N. S., Qu Y. Z., Wang Y. B. 2005. GMO: A
graph matching for ontologies In: Ashpole, Benjamin
and Ehrig et al., eds. Proceedings Of the KCAP 2005
workshop on Integrating Ontologies, Canada, October,
2005. Germany, CEUR-WS.org, 43-50.
Mahapatra L., Mohan M., Khapra M. 2010. OWNS:
Cross-lingual Word Sense Disambiguation Using
Weighted Overlap Counts and Wordnet Based
Similarity Measures In: Proceedings of the 5th
International Workshop on Semantic Evaluation,
Sweden, 2010. PA, USA: Association for
Computational Linguistics Stroudsburg, 138-141.
Tang J., Li J. Z., Liang B. Y. 2006. Using Bayesian
decision for ontology mapping. Journal of Web
Semantics, 4(4), 243-262.
Similarity-basedOntologyMappinginMaterialScienceDomain
269