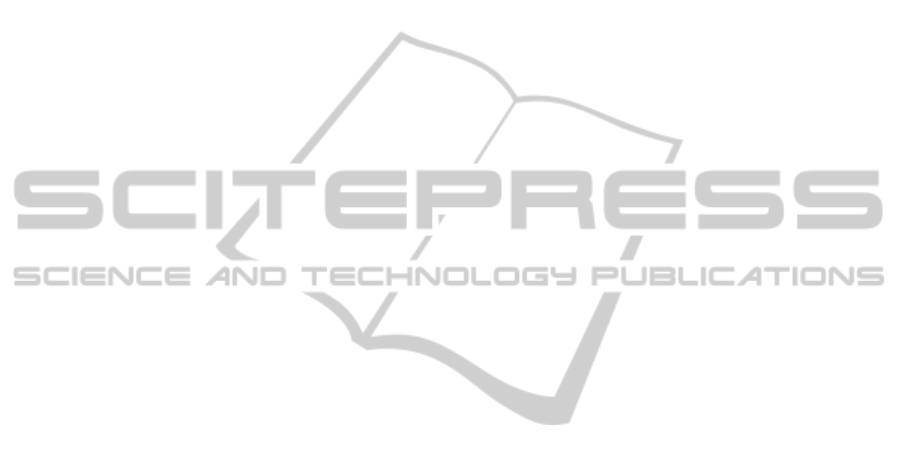
tion, and which existing features should be removed.
Four test subjects suggested to extend the friend-
functionality of the application. Besides adding and
removing users from their friend list, they would like
to see the context of their friends. They also men-
tioned the possibility to recommend items to friends
and to see their friends’ feedback on items. Three
test subjects indicated that the items of the category
“News” might be superfluous.
5 CONCLUSIONS
In this research, we investigated how the current con-
text and activity of the user can be recognized based
on sensor data and the accelerometer of his/her mo-
bile device. The context-recognition framework first
monitors and processes the sensor data to recognize
basic activities or context changes. Then these suc-
cessive basic activities are analyzed to recognize the
overall context of the user. An evaluation of the
framework proved that physical activities and the con-
text of the user can be recognized with a high accu-
racy and that this contextual information can be valu-
able knowledge for a context-aware recommender
system. Besides, the framework can be used for other
applications, e.g., for monitoring the physical activi-
ties of the user in the context of health care.
A user study showed that context-aware recom-
mendations are effective and helpful for discovering
new places and interesting information. Moreover,
user like to receive information tailored to their cur-
rent needs and consider the recommender application
as easy to use. These results confirm the necessity to
adapt (mobile) applications and service to the activity
and context of the user in order to improve the effec-
tiveness and the user experience.
REFERENCES
Adomavicius, G., Sankaranarayanan, R., Sen, S., and
Tuzhilin, A. (2005). Incorporating contextual infor-
mation in recommender systems using a multidimen-
sional approach. ACM Trans. Inf. Syst., 23(1):103–
145.
Bao, L. and Intille, S. (2004). Activity recognition from
user-annotated acceleration data. In Ferscha, A. and
Mattern, F., editors, Pervasive Computing, volume
3001 of Lecture Notes in Computer Science, pages 1–
17. Springer Berlin / Heidelberg.
Biegel, G. and Cahill, V. (2004). A framework for devel-
oping mobile, context-aware applications. In Pro-
ceedings of the Second IEEE International Confer-
ence on Pervasive Computing and Communications
(PerCom’04), PERCOM ’04, pages 361–, Washing-
ton, DC, USA. IEEE Computer Society.
Breese, J. S., Heckerman, D., and Kadie, C. (1998). Em-
pirical analysis of predictive algorithms for collabora-
tive filtering. In Proceedings of the Fourteenth confer-
ence on Uncertainty in artificial intelligence, UAI’98,
pages 43–52, San Francisco, CA, USA.
CultuurNet-Vlaanderen (2012). Uitdatabank developer
tools. Available at http://tools.uitdatabank.be/docs.
Dodson, B. (2012). Wikilocation. Available at http://
wikilocation.org/.
Foursquare (2012). Foursquare API. Available at https://
developer.foursquare.com/.
Google (2012a). Geocoding API. Available at https://devel-
opers.google.com/maps/documentation/geocoding/.
Google (2012b). Places API. Available at https://
developers.google.com/places/documentation/.
Herlocker, J. L., Konstan, J. A., Terveen, L. G., and Riedl,
J. T. (2004). Evaluating collaborative filtering recom-
mender systems. ACM Trans. Inf. Syst., 22(1):5–53.
HLN (2012). Rss news feed. Available at http://
www.hln.be/rss.xml.
Iacono, M., Krizek, K., and El-Geneidy, A. (2008). Ac-
cess to destinations: How close is close enough? esti-
mating accurate distance decay functions for multiple
modes and different purposes. Technical report, Uni-
versity of Minnesota, Twin Cities. Minnesota Depart-
ment of Transportation. ref.: MN/RC 2008-11.
Kenteris, M., Gavalas, D., and Mpitziopoulos, A. (2010). A
mobile tourism recommender system. In Proceedings
of the The IEEE symposium on Computers and Com-
munications, ISCC ’10, pages 840–845, Washington,
DC, USA. IEEE Computer Society.
Kwapisz, J. R., Weiss, G. M., and Moore, S. A. (2011).
Activity recognition using cell phone accelerometers.
SIGKDD Explor. Newsl., 12(2):74–82.
Lee, S.-W. and Mase, K. (2002). Activity and location
recognition using wearable sensors. Pervasive Com-
puting, IEEE, 1(3):24 –32.
Ravi, N., Dandekar, N., Mysore, P., and Littman, M. L.
(2005). Activity recognition from accelerometer data.
In Proceedings of the 17th conference on Innova-
tive applications of artificial intelligence - Volume 3,
IAAI’05, pages 1541–1546. AAAI Press.
Ricci, F. (2010). Mobile recommender systems. Informa-
tion Technology & Tourism, 12(3):205–231.
Savidis, A. and Stephanidis, C. (2010). Software refactor-
ing process for adaptive user-interface composition. In
Proceedings of the 2nd ACM SIGCHI symposium on
Engineering interactive computing systems, EICS ’10,
pages 19–28, New York, NY, USA. ACM.
Tiete, Y., Schmitz, S., and Colpaert, P. (2012). iRail API.
Available at http://project.irail.be/wiki/API/APIv1.
WEBIST2013-9thInternationalConferenceonWebInformationSystemsandTechnologies
490