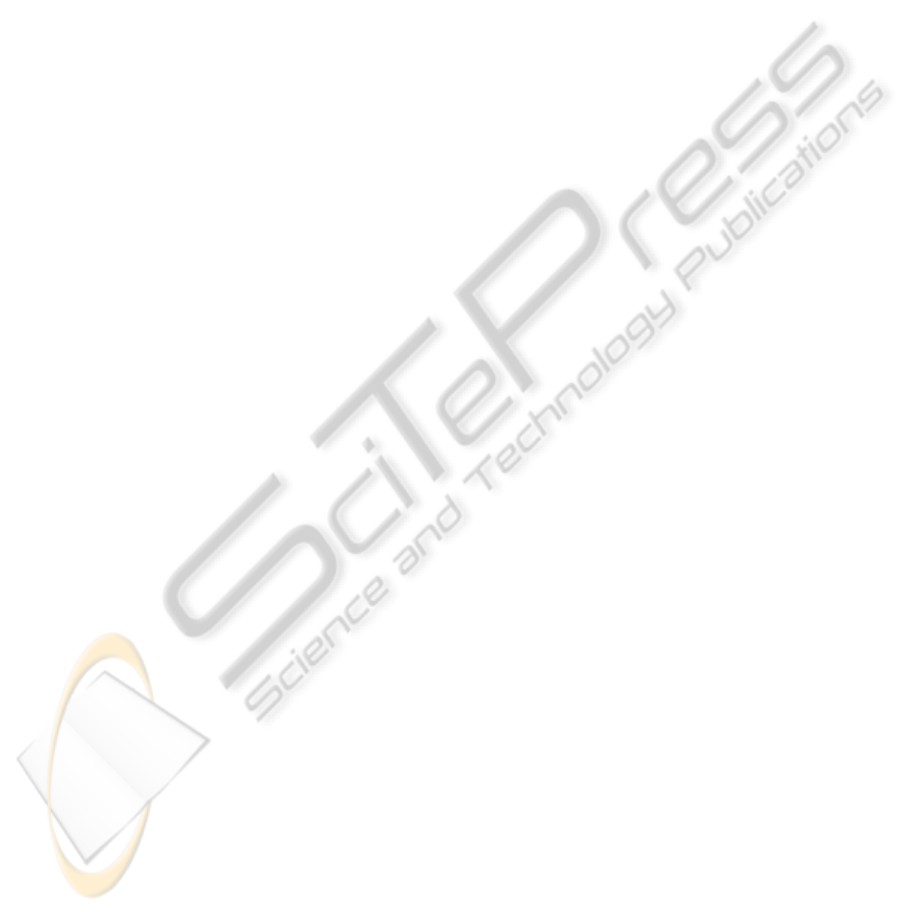
the same number of IMF for both images. One
image is the image to be classified (input, unknown
image), and the other one is a reference image of one
class. This decomposition is performed with each
one of the reference image of each class. Once the
decompositions are computed, the distance between
the modes, arranged as a matrix, are computed. The
classification is done using two different methods. In
the first one the classification is only based on the
lowest distance between input image and reference
images decompositions, and in the other method the
classification uses these distances as input vector of
a MLP.
Three different distance measures were analyzed
and Frobenius norm distance measure gave the best
results when the association is based exclusively on
the distance. The combination of the three distances
gave the best result when an ANN was used as a
classifier.
The success of the proposed method is promising
and will encourage us to continuing investigating the
use of mEMD decomposition as a feature extracting
system for face recognition problems, with new and
bigger data base.
ACKNOWLEDGEMENTS
This work has been partially supported by the
University of Vic under the grant R904, and under a
predoctoral grant from the University of Vic to Mr.
Esteve Gallego-Jutglà, ("Amb el suport de l'ajut
predoctoral de la Universitat de Vic"); and by
SAIOTEK from the Basque Government, to Dra.
Karmele López-de-Ipiña.
REFERENCES
Bishop, C. M., (1995). Neural Networks for Pattern
Recognition. Oxford University Press.
Diez, P. F., Mut, V., Laciar, E., Torres, A., Avilla, E.
(2009). Application of the Empirical Mode
Decomposition to the Extraction of Features form
EEG signals for Mental Task Classification. 31
st
Annual International Conference of the IEEE EMBS.
Freeman, J. A., Skapura, D. M. (1991). Neural Networks:
Algorithms, Applications and Programming
Techniques. Addison-Wesley Publishing Company,
Inc. Reading, MA
Gallego-Jutglà, E., Solé-Casals, J., “Exploring mEMD for
face recognition”, BIOSIGNALS conference
proceedings, BIOSTEC 2012.
Huang, N. E., Shen, Z., Long,S. R., Wu, M. C., Shih, H.
H:, Zheng, Q., Yen, N. C., Tung, C. C., Liu, H. H.
(1998). The empirical mode decomposition and the
Hilbert spectrum for nonlinear and non-stationary time
series analysis. Proc. R. Soc. Lond., 495, 2317-2345.
Hush, D. R., Horne, B. G. (1993). Progress in supervised
neural networks. IEEE Signal Processing Magazine,10
(1), pp. 8-39.
Iancu, C., Corcoran, P., Costache, G. (2007). A Review of
Face Recognition Techniques for In-Camera
Applications. International Symposium on Signals,
Circuits and Systems,1,1-4.
Lippmann, D. E. (1987). An Introduction to Computing
with Neural Networks. IEEE ASSP Magazine, 3(4),
pp. 4-22
Molla, K. I., Tanaka, T., Rutkowski, T. M., Cichocki, A.,
(2010). Separation of EOG artifacts from EEG singals
using bivariate EMD. Acoustics Speech and Signal
Processing (ICASSP), 2010 IEEE Interational
Conference On. 562-565.
Mutlu, A. Y., Aviyente, S. (2011). Mutivariate Empirical
Mode Decomposition for Quantifying Multivariate
Phase Synchronization. EURASIP Jounal on Advances
in Signal Processing. Article ID 615717
Rehman, N., Mandic, D. P., (2010). Multivariate empirical
mode decomposition. Proc. R. Soc. A. 466, 1291-
1302.
Travieso, C. M., Solé-Casals, J., Zaiats, V., Alonso, J. B.,
Ferrer, M.A., “Reducción del Vector de Características
en Reconocimiento Facial”, XXIII Simposium
Nacional de la Unión Científica Internacional de
Radio URSI 2008.
Woodward, J. D., Orlans, N.M., Higgins P. T. (2003).
Biometrics. McGraw-Hill.
Xiao Q. (2007). Technology review - Biometrics-
Technology, Application, Challenge, and
Computational Intelligence Solutions. IEEE
Computational Intelligence Magazine, 2, 5-25.
BIOSIGNALS2013-InternationalConferenceonBio-inspiredSystemsandSignalProcessing
450